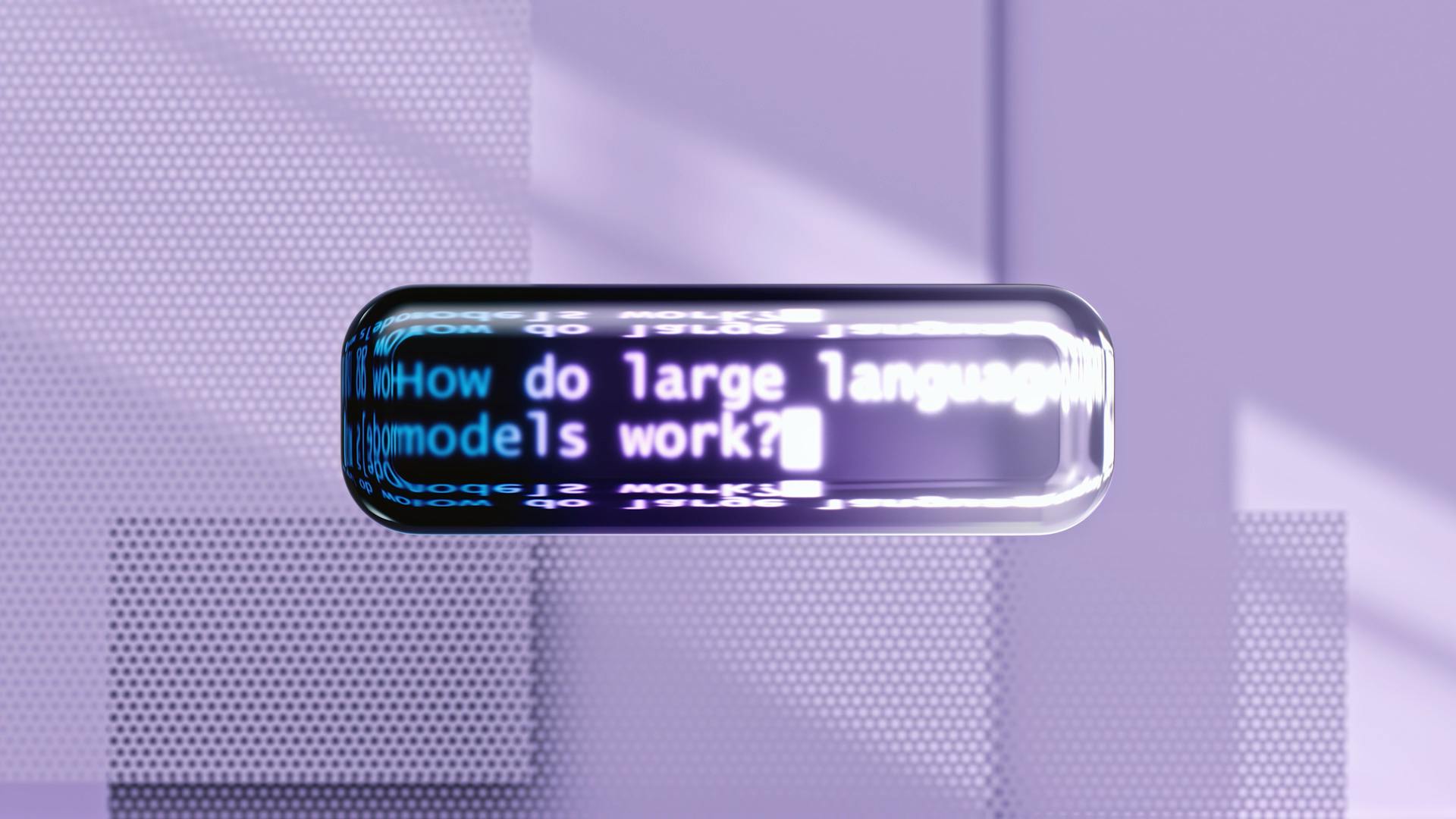
Generative AI biotech is revolutionizing the way we approach discovery and design in the biotech industry. By leveraging machine learning algorithms, researchers can now generate new molecules, proteins, and even entire organisms with unprecedented speed and accuracy.
This technology has the potential to accelerate the discovery of new medicines and treatments, which could lead to significant breakthroughs in the fight against diseases. For example, researchers have used generative AI to design new antibodies that are highly effective against certain types of cancer.
One of the key benefits of generative AI biotech is its ability to analyze vast amounts of data and identify patterns that would be impossible for humans to detect on their own. This allows researchers to make more informed decisions and design more effective experiments.
Discover more: Role of Generative Ai in Drug Discovery
Generative AI in Biotech
Generative AI has the potential to accelerate the process of identifying targets, developing validation assays, and preclinical testing to determine the effectiveness of new drugs, with a potential opportunity of $15 billion to $28 billion.
Explore further: The Economic Potential of Generative Ai
Pharmaceutical manufacturers are already using foundational models for these purposes, including image models to analyze microscopy and pathology data, and chemistry models to improve predictions for functional readouts of small-molecule data.
Gen AI can help accelerate gen AI's impact across pharmaceutical research, perhaps cutting drug discovery timelines in half, by augmenting foundational capabilities with broader, more established forms of AI.
GPT-powered knowledge extraction can alleviate the burden of extracting and summarizing information in documents such as patents, scientific publications, and trial data, providing a more than 30 percent increase in initial manual assessments of drug targets.
Researchers can pose open-ended Q&As, easily shift between different tasks, and frictionlessly integrate additional evidence through prompt engineering, without requiring little to no additional training to tailor information to specific use cases.
Gen AI tools trained on approved content can rapidly pull together tailored materials, including text, data tables, figures, infographics, videos, and audio, leading to a two to three times boost in engagement.
Generative AI in drug discovery leverages machine learning models, particularly generative adversarial networks (GANs) and transformer models, to create new drug candidates by predicting the components in a molecule's structure.
AI can design new molecules, optimize existing compounds, and predict biological activity, helping to prioritize the most promising candidates for further development.
Our Methodology
We based our analysis on the McKinsey Global Institute's impact assessment of 63 individual use cases, which were then mapped to specific life-science domains, such as commercial and clinical research.
Our quantification of the economic impact was a bottom-up approach, using expert opinion, McKinsey's internal experiments, and published research to estimate the potential range of impact for specific use cases, like the percentage of time saved when generative AI tools review clinical-research literature.
We scaled the impact to the life-science industry by considering the percentage of domain operating costs spent on tasks related to a generative AI use case, such as reviewing clinical research, and the percentage of industry revenues spent on a domain, like R&D.
The impending gen AI-driven life science revolution promises unquantifiable effects on human health and well-being, including accelerated drug discovery and more personalized treatments.
Generative AI can accelerate the screening process with state-of-the-art foundational chemistry models that can map millions of known chemical compounds by their structure and function.
For more insights, see: Impact of Generative Ai on Tax Industry
An up to 2.5 times increase in the performance of chemical compound activity models and a more than fourfold boost in speed in identifying new leads are potential outcomes of using generative AI in drug development.
Generative AI can be applied at various stages of drug discovery, starting with target identification, where it can analyze genomic data to pinpoint exact targets for new drug development.
By automating tedious and time-consuming tasks like document creation and record keeping, gen AI stands to boost the productivity of researchers and medical liaisons, allowing them to better serve clinicians and patients.
Take a look at this: Google Cloud Skills Boost Generative Ai
Benefits and Impact
Generative AI is revolutionizing the biotech industry, offering numerous benefits and a significant impact on the development of new treatments.
It can accelerate the drug discovery timeline, reducing it from over a decade to just a few years. This is crucial for bringing life-saving treatments to patients faster.
Developing a new drug typically costs around USD 2.6 billion, but generative AI can reduce these costs by streamlining the discovery phase and minimizing the need for extensive lab work.
Generative AI utilizes advanced algorithms to design drug molecules with high precision, ensuring that the compounds created possess the intended properties.
Integrating generative AI in drug discovery leads to higher success rates, as it accurately predicts molecular behavior and biological interactions.
It facilitates the development of personalized treatments by analyzing individual patient data, such as genetic profiles.
Virtual screening powered by generative AI allows researchers to quickly analyze how millions of compounds interact with specific biological targets.
Generative AI can help mitigate risks by identifying potential pitfalls early in drug development, minimizing the risk of expensive failures in clinical trials.
The cost of bringing one new drug to market is rising, but gen AI could help accelerate the process of identifying targets, developing validation assays, and singling out the most promising leads.
Pharmaceutical manufacturers are already using foundational models for these purposes, and augmenting these capabilities with broader forms of AI can accelerate gen AI’s impact across pharmaceutical research.
This could potentially cut drug discovery timelines in half, bringing new treatments to market faster and improving patient outcomes.
Recommended read: Generative Ai for Software Development
Optimization and Efficiency
Generative AI in biotech is revolutionizing the way we approach complex problems, and one of the most exciting areas is optimization and efficiency. By leveraging the power of next-generation LLMs, researchers can predict the disease-treating potential of large molecules, such as proteins and mRNA, which hold promise for more targeted therapies and better vaccines.
This can lead to an acceleration of more than three times in large-molecule design, making it a game-changer for the industry. By automating the design process, researchers can focus on more critical tasks and make breakthroughs faster.
Gen AI can also help optimize clinical trials by identifying the right patients to study and predicting their response to different treatments. This can lead to a 10 percent increase in the possibility of success for trials, a 20 percent reduction in their cost and duration, and a time to approval accelerated by one to two years.
With gen AI models built on data from medical-imaging techniques, scientists can even identify entirely new biomarkers, leading to unforeseen new treatments.
Check this out: Which Is One Challenge in Ensuring Fairness in Generative Ai
Optimizing Large Molecules
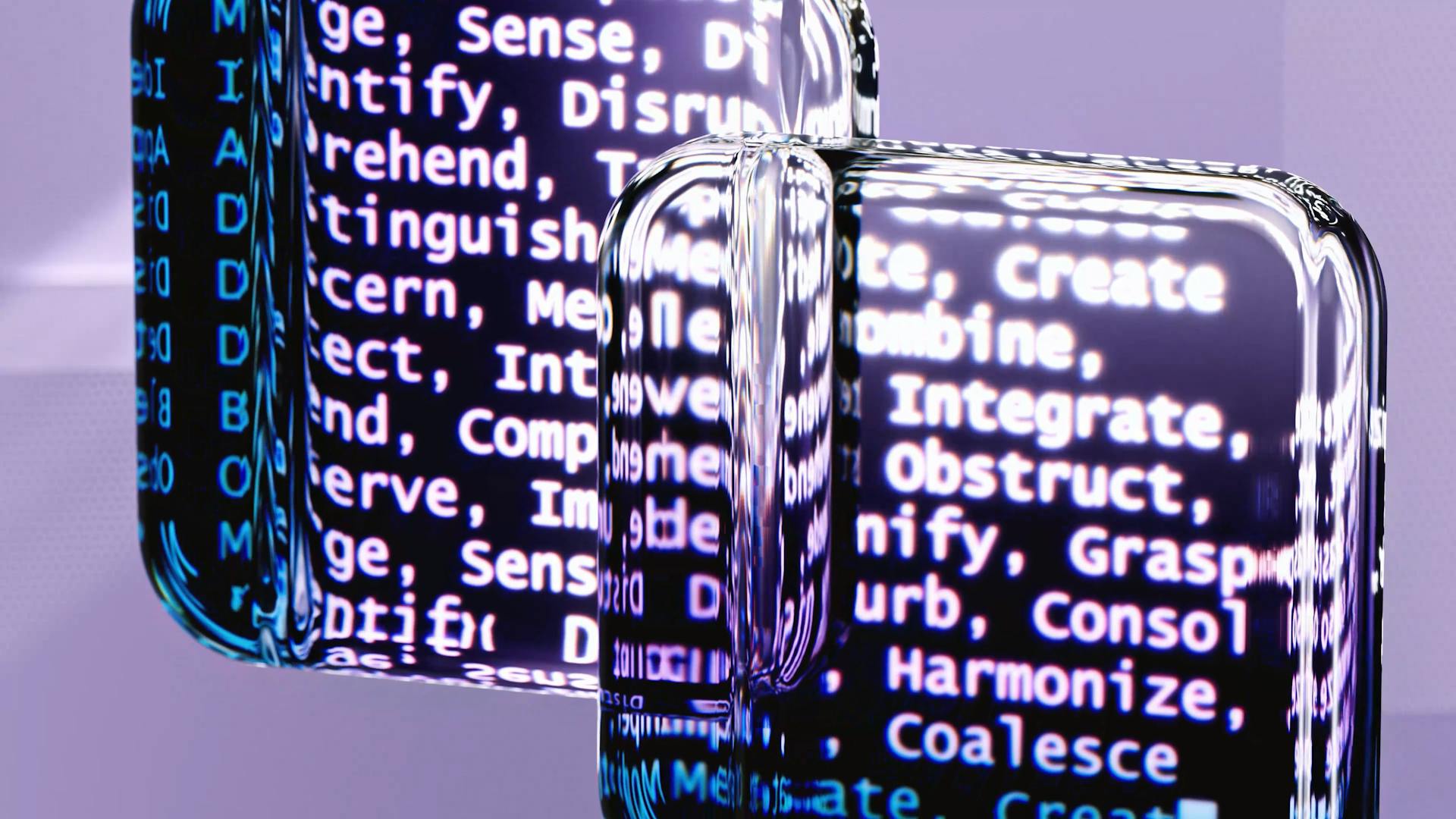
Optimizing large molecules is a significant challenge in drug discovery, but next-generation LLMs can help by learning to predict the next substructure of large molecules.
These molecules, such as proteins and mRNA, hold promise for more targeted therapies and better vaccines, but their complexity increases development costs and discovery timelines.
Researchers can use LLMs to generate insights about large-molecule chemistry, which can be used for in silico design of new drug vectors.
This can lead to an acceleration of more than three times in large-molecule design, making it a game-changer in the field of drug discovery.
Additional reading: Generative Ai with Large Language Models
Optimizing Trials and Portfolios
Clinical trials often struggle to identify the right patients to study, which can slow down treatment development. This is because researchers can't easily pinpoint who will respond to a treatment.
Gen AI is changing this by helping researchers use biomarkers to stratify patients according to their probability of progression or response to treatment. This is already happening in precision oncology, where it's greatly improved the chances of success.
By analyzing genetic and phenotypic data alongside real-world data, researchers can better understand why different subgroups of patients respond differently to the same treatments. This knowledge will help assemble more diverse and representative clinical-trial populations.
Using Gen AI models built on data from medical-imaging techniques like X-rays and MRIs could even identify new biomarkers and lead to unforeseen new treatments. This could shorten trials and increase their chances of success.
The potential impact of Gen AI on clinical trials is significant: a 10 percent increase in the possibility of success, a 20 percent reduction in cost and duration, and a one- to two-year acceleration in time to approval. This could lead to a double-digit impact on the net present value of assets or portfolios.
If this caught your attention, see: Generative Ai Environmental Impact
Sources
- https://www.mdpi.com/2673-7426/4/2/79
- https://blogs.nvidia.com/blog/drug-discovery-bionemo-generative-ai/
- https://www.mckinsey.com/industries/life-sciences/our-insights/generative-ai-in-the-pharmaceutical-industry-moving-from-hype-to-reality
- https://www.veritis.com/blog/from-concept-to-cure-generative-ai-in-drug-discovery/
- https://www.forbes.com/sites/bernardmarr/2024/06/19/how-generative-ai-is-accelerating-drug-discovery/
Featured Images: pexels.com