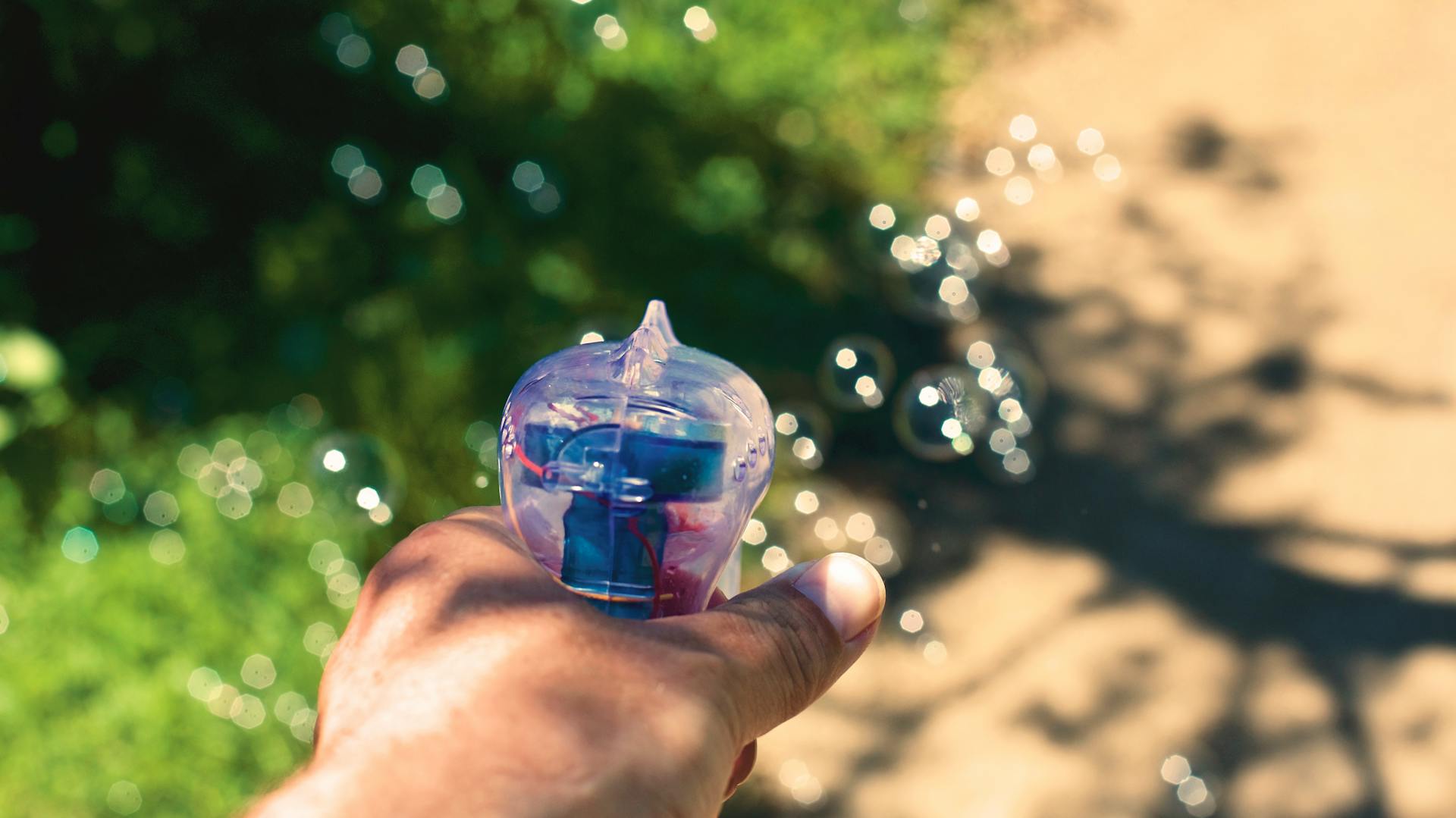
The generative AI bubble is a growing concern, with many experts warning of an impending collapse.
Investors have poured billions of dollars into generative AI startups, leading to a surge in valuations and hype.
This rapid growth has created unrealistic expectations and a sense of urgency around the technology's adoption.
What's the Issue?
Humans have always been resistant to adopting new technologies, especially those they don't fully understand. It's a scary step to take, and every breakthrough feels like a "bet" against the unknown.
The problem is that most people don't switch to a new technology until they're sure its utility and safety justify the risk. This hesitation is rooted in fear and a lack of understanding.
Model hallucinations, such as the example of how many r's are in strawberry, are a significant issue with generative AI. It's a problem that's virtually unsolvable.
Generative AI models can't even auto-discriminate, meaning they can't tell whether they're doing a task correctly or not. This is a major limitation.
Security vulnerabilities are another major problem with generative AI. These issues are not just theoretical, but real and present dangers.
Here's an interesting read: Generative Ai Not Working Photoshop
Beyond the Hype
The hype surrounding generative AI can be overwhelming, but experts say we're actually in a corrective phase, where enthusiasm is being balanced out with practical realities. This isn't the first time we've seen this happen, as Chris Hay from IBM notes that similar corrections occurred with the early internet and Thomas Watson's infamous prediction that the world wouldn't need more than 5 computers.
The media often conflates generative AI with narrower applications like LLM-powered chatbots, which might not be equipped to solve every problem enterprises face. This can lead to unrealistic expectations and a backlash against the hype.
As Smolinksi argues, the answer is somewhere in the middle - AI needs to be part of a broader strategy to solve business problems, and it's usually not just about AI itself, but using multiple types of AI models in tandem to solve a problem.
Take a look at this: Gartner Generative Ai Hype Cycle
Four Crises
The AI landscape is facing some serious challenges, and it's essential to understand the four crises that are currently affecting the field. The data crisis is one of them, where the vast troves of data used to train LLMs are diminishing in value.
Publishers and online platforms are locking up their data, leaving developers with a dwindling supply of training data. This could have significant implications for the future of AI development.
The compute crisis is another hurdle, where the demand for GPUs to process data is leading to a bottleneck in chip supply. This shortage is already causing delays in the development of AI models.
Companies developing the largest LLMs are consuming more power every year, leading to the power crisis. Our current energy infrastructure is not equipped to keep up with the demand, making it a pressing issue.
The use case crisis is perhaps the most significant challenge, where generative AI has yet to find its "killer app" in the enterprise context. Some critics even suggest that future applications might not extend beyond "parlor trick" status.
The four crises are interconnected, and solving the use case crisis might help resolve the other three. Here are the four crises in a concise list:
- The data crisis: Diminishing value of training data
- The compute crisis: Bottleneck in GPU supply
- The power crisis: Insufficient energy infrastructure
- The use case crisis: Lack of meaningful enterprise applications
Misinformation and Poor Research
Misinformation and poor research are rampant online, and AI models are often at the center of the problem.
Generative AI models, like LLMs and RAGs, are taking over the internet, but it's crucial to be specific about what we call AI and what models we use.
AI models like ChatGPT have become ubiquitous, but GenAI is a more specific term that refers to models like ChatGPT, Claude, Gemini, Llama, and others.
We need to be careful not to generalize AI models, as they have different environmental impacts.
AI models have found their way into every corner of the internet, but being specific about what we call AI is crucial for accurate research.
Beyond the Hype Cycle
We're currently in the corrective phase of the hype cycle, where the media is trying to balance out earlier statements that seemed like hype. This phase is a natural part of any new technology's adoption.
IBM distinguished engineer Chris Hay believes that generative AI is having a marked, measurable impact on businesses and clients, fundamentally changing the way they work. This is across all industries and disciplines, from transforming HR processes to contact centers or software development.
For your interest: Generative Ai Hype
The recent media pessimism about AI can be seen as an attempt to balance out earlier statements that seemed like hype. This is a good thing, as it will help to temper unrealistic expectations and bring the industry back to reality.
According to Hay, part of the problem is that the media often conflates generative AI with a narrower application of LLM-powered chatbots. This can lead to a misunderstanding of the technology's capabilities and limitations.
The AI market is experiencing a correction, not a burst. This means that some AI ventures that lack practical use cases may fail, but the industry will still see long-term growth and transformation.
IBM's Global Head of Tech, Data and AI Strategy, Brent Smolinksi, argues that AI needs to be part of a broader strategy to solve business problems. It's usually not AI by itself that solves a problem, but rather a combination of AI models and other technologies.
Hay is confident that even more compelling use cases justifying the cost of these models will emerge. This will help to drive adoption and growth in the industry.
In fact, a recent survey of 1,400 C-suite executives found that most expected AI and generative AI to deliver cost savings this year. While most were still at the experimental stage with deployments, around a quarter expected savings in excess of 10%.
Intriguing read: Generative Ai Use Cases in Healthcare
Agentic Workflows
Agentic workflows are a powerful tool that uses LLMs and AI agents to perform tasks in a multi-step approach. This approach allows agents to act with a degree of independence and decision-making capability.
These agents can be designed to handle specific tasks or areas of expertise, bringing in deep knowledge and experience that LLMs might lack. They can either draw on more specialized data or integrate domain-specific algorithms and models.
Imagine a telecommunications company where an agentic workflow efficiently manages customer support inquiries. Agents can operate autonomously and proactively, in real time, by using simpler decision-making algorithms.
Agents can use reinforcement learning or feedback loops to improve performance over time, adjusting strategies based on the success or failure of previous tasks. This allows them to learn from their environment and adapt to new situations.
Agentic workflows themselves generate new data, which can then be used for further training. This creates a cycle of improvement, where the workflow becomes more efficient and effective over time.
Additional reading: Large Language Model vs Generative Ai
Take a Measured Approach
A whopping 43% of executives and CEOs already use Generative AI to inform strategic decisions, which is a clear indication that the hype is real. Many CIOs are under pressure from CEOs or board members to find value now, but this can lead to a "gen-AI-or-bust" strategy that's not sustainable.
To avoid this, CIOs should focus on strategic organizational goals and priorities, and not just digital assistants and intelligent chatbots. They need to be able to articulate the business value and expected ROI of each project.
Fostering a culture of continuous learning and upskilling is also essential to build internal AI capabilities. This will help CIOs to fine-tune existing AI models for specific use cases before spending money on training models from scratch.
In fact, fine-tuning existing AI models can often yield better results with less effort, allowing organizations to quickly realize the benefits of gen AI. CIOs should start by finding problems their organizations need to solve, then consider a wide range of technologies, not just gen AI.
Considering the Impact
The generative AI bubble has the potential to disrupt various industries, including education, where AI-powered tools can automate grading and provide personalized learning experiences.
The bubble is also expected to impact the job market, with some studies suggesting that up to 30% of jobs could be automated by 2030.
As AI-generated content becomes more prevalent, there's a growing concern about the authenticity of information online.
The rise of AI-generated art and music has sparked debates about authorship and ownership.
The bubble's impact on the creative industries is still unclear, but it's likely to be significant, with some experts predicting a shift towards more collaborative and hybrid forms of creativity.
Check this out: Generative Ai Environmental Impact
Conclusion
The generative AI bubble is a stark reminder that hype and misinformation can have disastrous effects on our market. False or partially false information is very real and can have a significant impact on the economy.
Double-checking information is crucial to prevent the spread of misinformation. Truth is an unattainable absolute, but that's no excuse to post carelessly.
The AI boom is just another example of how hype and misinformation are combined. This has led to increased market volatility, with stocks becoming more volatile each day.
We need to be mindful of how our emotions and ideas are affecting our market. The negative economic and social impact of the generative AI bubble is a wake-up call for us all.
Here's an interesting read: Generative Ai Market Size
Sources
- https://www.ibm.com/blog/with-generative-ai-dont-believe-the-hype-or-the-anti-hype/
- https://towardsdatascience.com/bursting-the-ai-hype-bubble-once-and-for-all-581a994fe762
- https://www.cio.com/article/3485333/is-the-gen-ai-bubble-due-to-burst-cios-face-rethink-ahead.html
- https://www.lemonde.fr/en/pixels/article/2024/07/14/after-euphoria-fear-of-bubble-grows-around-generative-ai_6683589_13.html
- https://www.cio.com/article/3499069/the-deflating-ai-bubble-is-inevitable-and-healthy.html
Featured Images: pexels.com