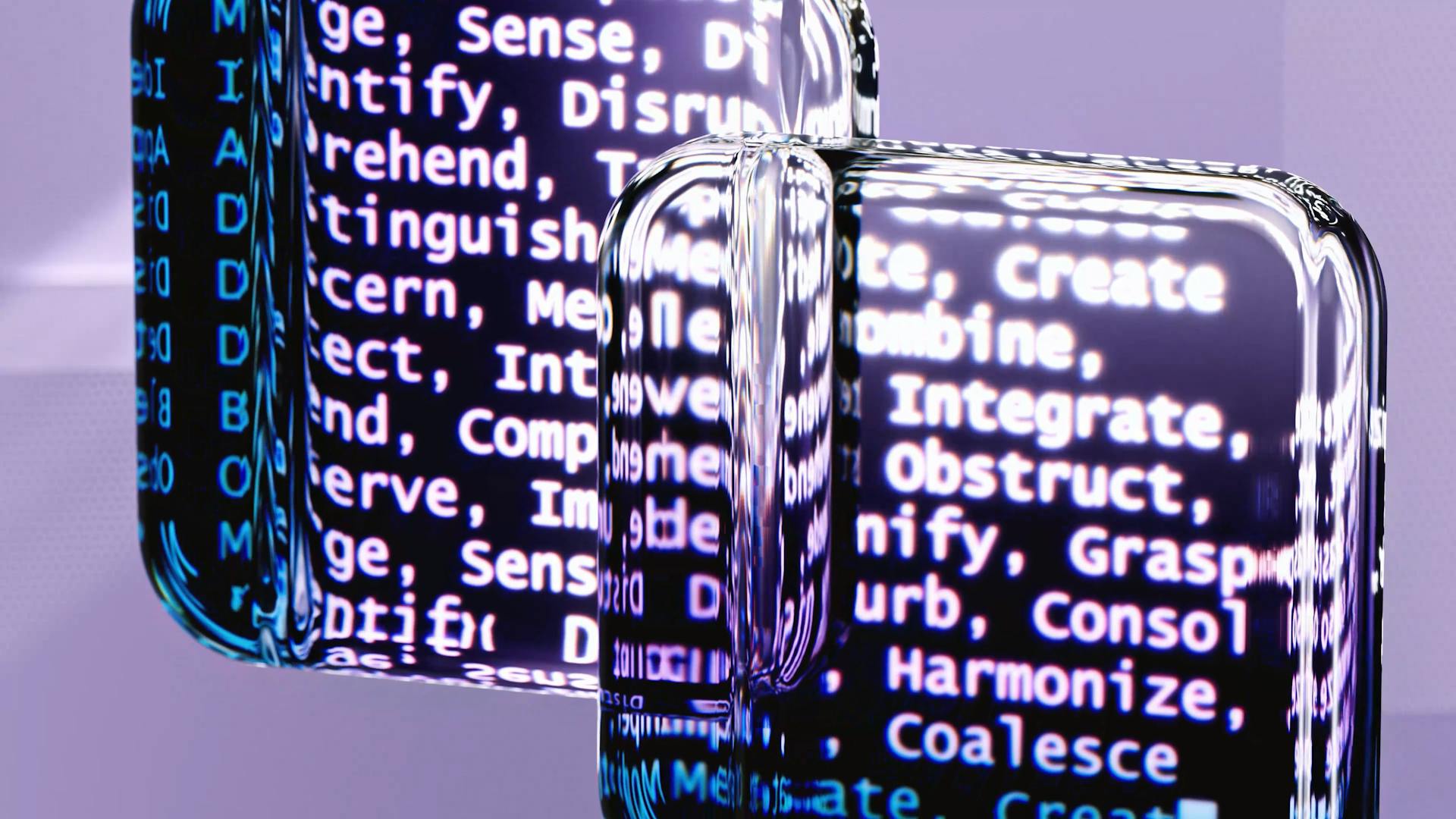
Generative AI for business is revolutionizing the way companies operate, and it's not just a buzzword.
AI-powered solutions are transforming industries by automating manual tasks and freeing up human resources for more strategic work.
According to a recent study, companies that adopt AI-powered solutions see an average increase in productivity of 25%.
This shift towards automation is not just about efficiency; it's also about staying competitive in a rapidly changing market.
By leveraging generative AI, businesses can create new products, services, and experiences that meet the evolving needs of their customers.
In fact, a survey found that 70% of businesses believe that AI will be crucial to their future success.
Consider reading: Generative Ai Companies
What Generative AI Can Do
Generative AI can automate manual or repetitive tasks, allowing employees to focus on more strategic work, leading to significant cost optimization for businesses of all sizes.
Generative AI can accelerate tasks like content creation and data analysis, saving businesses time and money. For example, generative AI can generate personalized marketing campaigns, product descriptions, and customer support responses.
Generative AI can help businesses increase revenue by enhancing customer engagement and driving sales. For example, generative AI can be used to create targeted recommendations, personalized product suggestions, and engaging social media content.
Generative AI can help businesses identify patterns, trends, and potential issues in data, enabling them to proactively address challenges and minimize risks. For example, generative AI can be used to identify fraud, detect cybersecurity threats, etc.
Generative AI can help businesses innovate by generating creative content and ideas. Generative AI business ideas can help them explore new possibilities and develop new products and services. For example, generative AI can be used to design new drug molecules, create innovative advertising campaigns, and develop new business models.
Here are some specific use cases for generative AI in business:
- Automated content creation: Generative AI can create marketing materials, product descriptions, and social media posts.
- Report generation: Generative AI can automate the generation of reports, charts, and summaries.
- Content scaling: Generative AI can automate the creation of blog posts, articles, and other content.
- Graphic design: Generative AI can create graphics, logos, and visual assets.
- Artistic content: Generative AI can assist artists and designers in generating creative concepts for projects.
- New product development: Generative AI can expedite the design process and produce optimized designs grounded on specific parameters.
By leveraging generative AI, businesses can streamline operations, ignite creativity, drive innovation, optimize investments, and elevate customer satisfaction.
Applications in Business
Generative AI can be applied in various business areas, including customer service, personalization, and business process automation. It can help improve the overall customer experience by providing prompt and precise answers to inquiries, making tailored suggestions based on purchase history and preferences, and identifying and resolving customer complaints and issues.
For your interest: Ai Software for Business
Generative AI can also be used for business process automation, streamlining tasks such as performing managerial tasks, searching for information, and creating documents and reports. This can increase productivity and efficiency, with McKinsey estimating that strategic use of generative AI can automate up to 70% of repetitive tasks.
Some businesses have successfully implemented generative AI to improve their operations, such as Leroy Merlin, which used it to accelerate the refund process, reducing the time from 15 days to 1.5-2 days.
If this caught your attention, see: Generative Ai and Cybersecurity
Customer Service
Customer service is a vital field where generative AI tools like ChatGPT can address challenging business problems. Generative AI can provide customers with prompt and precise answers to their inquiries, improving the overall customer experience.
Chatbots powered by ChatGPT can make tailored suggestions to customers based on their purchase history and preferences. For instance, a retailer may employ ChatGPT to create a chatbot that can help customers locate products, answer inquiries, and process orders.
For more insights, see: Chatgpt Openai Generative Ai Chatbot Can Be Used for
A healthcare provider may deploy a ChatGPT-driven chatbot that can help patients schedule appointments, provide information about medical procedures, and answer health-related questions. This can help healthcare providers streamline their operations, reduce wait times, and enhance patient outcomes.
By leveraging generative AI, businesses can boost their customer experience, enhance operational efficiency, and drive growth. Research shows that companies can see a 14% increase in issue resolution per hour and a 9% reduction in issue handling time by using generative AI in customer service.
Generative AI can also be used to identify and resolve customer complaints and issues, ensuring timely resolution and customer satisfaction. For example, Expedia Group uses ChatGPT to power an AI assistant in their app, enabling users to consult an AI-powered personal assistant for travel advice.
The AI assistant can suggest destinations, hotels, and transportation options based on customer preferences and behavior. This can help users make informed decisions about their travel plans and potentially earn rewards.
Here are some key industries that can benefit from generative AI in customer service:
- Retail
- Healthcare
- Banking
- Insurance
- Capital markets
- Energy
- Utilities
These industries can use generative AI to create personalized product recommendations, targeted marketing campaigns, and customized customer support interactions. By doing so, they can enhance customer satisfaction, loyalty, and retention.
For your interest: Generative Ai Customer Experience
Handling HR Processes
Handling HR processes is a crucial aspect of any business, and generative AI can greatly support corporate HR operations. ChatGPT, for example, can mechanize repetitive HR chores while delivering exact and swift answers to staff inquiries.
Generative AI can be utilized to devise a virtual HR assistant that helps employees with tasks like managing leaves, administering benefits, and introducing new hires to the organization. This virtual assistant can also offer tailored career development recommendations to workers based on their skills and interests, thereby improving employee participation and retention.
According to McKinsey, generative AI can automate up to 70% of repetitive tasks, significantly increasing productivity with an annual improvement rate of 3.3%. This means businesses can optimize their HR procedures, downsize labor costs, and enhance worker satisfaction and retention.
Here are some examples of how generative AI can improve HR processes:
- Automating tasks like managing leaves and administering benefits
- Providing tailored career development recommendations
- Screening resumes and job descriptions to spot competent candidates for unoccupied positions
- Scheduling interviews and streamlining the recruitment process
These benefits can lead to a more efficient and effective HR department, allowing businesses to focus on more strategic and creative tasks.
Process Optimization Across Industries
Process optimization is a crucial aspect of business, and generative AI can play a significant role in achieving it. By automating repetitive and rule-based tasks, generative AI can mitigate the risk of errors and accelerate task completion.
Generative AI can be used to optimize various business processes, including supply chain management, quality control, and workflow optimization. For instance, it can predict demand, optimize inventory, and anticipate supply chain disruptions, leading to cost savings and better inventory management.
In the manufacturing industry, generative AI can be used to monitor manufacturing processes and identify product defects, ensuring consistent product quality. Additionally, it can be used to automate tasks such as data entry, report generation, and content creation, freeing up human resources for more strategic and creative tasks.
Here are some examples of industries that can benefit from generative AI-powered process optimization:
- Supply chain management: Predict demand, optimize inventory, and anticipate supply chain disruptions.
- Quality control: Implement AI systems to monitor manufacturing processes and identify product defects.
- Task automation: Automate repetitive and rule-based tasks to mitigate errors and accelerate task completion.
By leveraging generative AI, businesses can streamline their processes, reduce costs, and improve overall efficiency. As the technology continues to evolve, its potential applications will only continue to grow.
Technologies and Techniques
Conducting a technical feasibility assessment is a crucial step in integrating generative AI into existing systems. This involves evaluating the complexity of integration and determining whether custom model development is necessary or if pre-trained models can be utilized.
Custom model development may be necessary for certain use cases, while pre-trained models can be a more efficient option for others. The computational requirements for different use cases should also be considered.
Pre-trained models can be a great starting point, but they may need to be fine-tuned for specific business needs. Techniques like transfer learning can help adapt pre-trained models to your business needs.
Here are some examples of how generative AI can be applied in various industries:
- Voice synthesis: Synthesize realistic human-like voices for voice assistants.
- Audiobook narration: Convert text into natural-sounding audio for producing audiobooks, podcasts, and voiceovers.
Types of Generative AI
Generative AI models have revolutionized various industries with their ability to enhance medical diagnoses and transform content generation processes. These versatile models are redefining the way we approach complex problems and tasks.
There are multiple generative AI models excelling in specific domains and applications, including Transformer-Based Models, Variational Autoencoders (VAEs), Unimodal Models, Multimodal Models, Generative Adversarial Networks (GANs), and Large Language Models (LLMs).
Transformer-Based Models, such as GPT-3 and BERT, are exceptional for text generation and code completion. They are designed to handle complex tasks like natural language processing and machine translation.
VAEs are effective in creating realistic images, audio, and video content. They operate with an encoder-decoder architecture, allowing them to generate content while preserving key features.
Unimodal Models focus on tasks such as text generation, image synthesis, or audio generation based on the specific input data type. Examples of Unimodal Models include WaveGAN and BigGAN.
Multimodal Models are tailored to accept various types of inputs and prompts when generating outputs. For example, GPT-4 is a multimodal AI model capable of processing both text and images.
GANs consist of two neural networks, a generator, and a discriminator, that work in opposition to produce realistic outputs. They are ideal for tasks like image duplication and synthetic data generation.
LLMs are the most widely recognized generative AI models today, capable of generating and completing content on a large scale. They are widely used in tasks like generating news articles, answering questions, and creating conversational agents.
Expand your knowledge: Telltale Words Identify Generative Ai Text
Here's a brief overview of the different types of generative AI models:
Diffusion
Diffusion models are perfect for image generation and image synthesis. They can be used to recreate photographs from noisy inputs.
Diffusion models apply a gradual noise reduction process to generate high-quality images. This process can also be used to generate realistic scenes and objects.
Worth a look: Can I Generate Code Using Generative Ai
Potential Career Paths
In the field of business-applied generative AI, there are many exciting career paths to explore.
If you're interested in creative roles, you might consider becoming an AI Art Director, leveraging generative techniques to create innovative visuals.
Generative AI is also being used in content creation, with AI Content Creators/ Writers generating high-quality content for various industries.
For those with a technical background, AI Developer (Generative Models) and Machine Learning Engineer (Generative AI) are in-demand roles that involve designing and implementing generative models.
Data Scientists with expertise in virtual modeling are also in high demand, as they can provide valuable insights using generative AI techniques.
For another approach, see: Generative Ai Content Creation
Deep Learning Specialists (Generative Algorithms) work on developing and improving generative algorithms, which are the backbone of many AI applications.
In addition to these technical roles, there are also opportunities in business and management, such as Generative AI Product Manager and Supply Chain Project Manager.
Here are some examples of potential career paths in business-applied generative AI:
Design PoC
Designing a proof of concept (PoC) is a crucial step in implementing generative AI in your business. It involves outlining the technical requirements, model selection, and integration points with existing systems.
Data preprocessing is a key aspect of a PoC, as it determines the quality and quantity of data that will be used to train the generative AI model. This requires careful consideration of data integration points with existing systems.
Performance metrics and evaluation criteria should also be established to measure the success of the PoC. This will help you determine whether the generative AI solution is meeting its intended goals.
On a similar theme: Velocity Model Prediciton Using Generative Ai
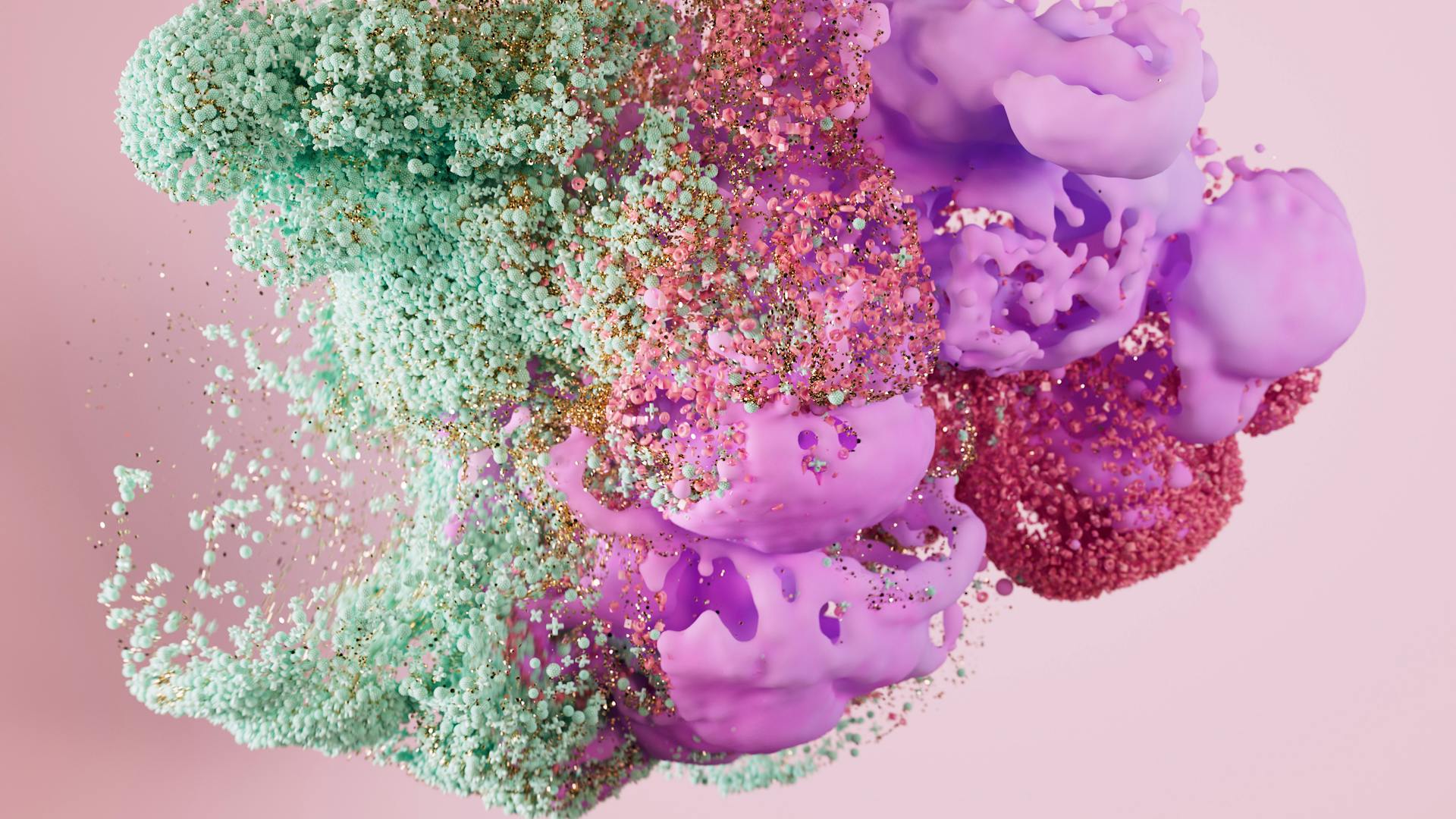
Here are some key points to consider when designing a PoC:
By considering these aspects, you can create a comprehensive PoC that sets your business up for success with generative AI.
Tech Stack for Integration
To build a robust tech stack for generative AI integration, consider the following key components:
A well-structured tech stack serves as the foundation for integrating generative AI solutions seamlessly into your operations. This stack should include cloud computing platforms like AWS, Google Cloud, or Azure, which provide scalable infrastructure to handle the computational demands of AI workloads.
Developers can utilize foundation models (FMs) from organizations such as OpenAI, Anthropic, or Cohere, or opt for open-source alternatives. Custom models can also be trained to meet specific requirements.
Hosting generative AI models on dedicated servers or deploying them on edge devices and browsers can enhance security while reducing latency and costs.
A comprehensive inventory of existing data sources is crucial for successful generative AI implementation. This includes identifying all potential data sources across the organization, including structured, semi-structured, and unstructured data.
Curious to learn more? Check out: Google Cloud Skills Boost Generative Ai
Here are the key components of a generative AI tech stack:
By assembling these components, you'll be well on your way to building a robust tech stack for generative AI integration.
Real-Life Examples and Industries
Generative AI has been successfully implemented in various businesses to solve complex problems, with real-life examples including customer service, supply chain management, and marketing.
Businesses in the customer service sector are using AI to enhance their services, with real-world use cases including AI-assisted chatbots and virtual assistants.
Generative AI is also being used in marketing through seven use cases, including content generation, sentiment analysis, and predictive analytics.
A fresh viewpoint: Generative Ai for Customer Support
Industries That Can
Generative AI is transforming various industries in remarkable ways. One exciting example is its application in employment, where it can scrutinize job data, align candidates with suitable opportunities, and optimize recruitment processes for employers.
Appinventiv successfully implemented generative AI to reduce JobGet's job fulfillment period to just 3 days, making the employment landscape more accessible, instant, and efficient. This led to a significant funding of $52M.
Generative AI is also making waves in manufacturing, where it can automate complex decision-making processes, making things more efficient and pushing innovation at every step of the production lifecycle. This technology is no longer just a trendy addition but a game-changer.
Material science is another arena where generative AI is making a significant impact. Researchers at the Center for Functional Nanomaterials have successfully employed generative AI to discover novel self-assembling nanostructures, opening up possibilities for generative AI business applications.
Generative AI is poised to revolutionize whole industries, transforming the way we create art, music, and content, and disrupting education, healthcare, logistics, and product development. Its potential is infinite, and it's changing the game in many areas.
Material Science
Material science is an exciting field where generative AI is making a real difference. Researchers at the Center for Functional Nanomaterials have successfully used generative AI to discover novel self-assembling nanostructures.
This breakthrough has opened up possibilities for generative AI to uncover materials that improve the efficiency of batteries, solar cells, automotive parts, and other technologies.
Benefits and ROI
By adopting generative AI solutions, businesses can expect a positive Return on Investment (ROI) through various means.
Cost optimization is one of the significant benefits of generative AI, allowing employees to focus on more strategic work and leading to significant cost savings for businesses of all sizes.
Generative AI can accelerate tasks like content creation and data analysis, saving businesses time and money. For example, generative AI can generate personalized marketing campaigns, product descriptions, and customer support responses.
Increased revenue is another key benefit, as generative AI can enhance customer engagement and drive sales. For instance, generative AI can be used to create targeted recommendations, personalized product suggestions, and engaging social media content.
Generative AI can also help businesses innovate by generating creative content and ideas. This can lead to the development of new products and services, such as designing new drug molecules or creating innovative advertising campaigns.
To ensure a positive ROI, it's essential to prioritize the right use case and develop a scoring matrix to weigh factors such as potential revenue impact, cost reduction opportunities, and technical complexity.
A different take: Generative Ai Product Prototype
The financial aspect of developing generative AI applications can be significant, with costs varying widely depending on the approach taken. However, initial expenses typically cover data acquisition, computing resources, expert personnel, and integration into existing systems.
Here are some estimated costs for developing generative AI applications:
By tracking ROI through metrics like enhanced operational efficiency, cost savings, revenue growth, and improved customer interactions, businesses can gauge the financial viability and strategic success of their generative AI projects.
Getting Started and Implementation
Developing a well-thought-out strategy is crucial for successfully integrating generative AI into your business. This involves starting with small pilot projects to test how generative AI performs in specific areas of your business.
To begin, consider implementing pilot programs to gauge the effectiveness of generative AI in various sectors of your business. This will help you identify areas where generative AI can be most beneficial and allow you to refine your approach accordingly.
Related reading: Generative Ai for Business Leaders
Collaborative learning is also essential in building a collective understanding of generative AI across departments. By promoting teamwork, you can foster a culture of innovation and experimentation that drives the adoption of generative AI.
Iterative development is key to continually improving the AI systems based on feedback and changing business needs. This approach enables you to refine your generative AI models and adapt to new challenges as they arise.
A scalable infrastructure is necessary to meet the growing demands of AI as it becomes more embedded in your operations. Investing in such infrastructure will ensure that your business can handle the increased computational requirements of generative AI.
Here are the key steps to get started:
- Pilot programs
- Collaborative learning
- Iterative development
- Scalable infrastructure
Implementation and Integration
To successfully integrate generative AI into your business, it's essential to involve developers early in the planning process. They can help ensure a smooth adoption process by planning how the chosen model integrates with your existing systems and workflows.
Developers take the lead in integrating models into existing business applications, creating APIs or interfaces that allow seamless communication between the foundation model and the application. They also handle aspects like data preprocessing, output formatting, and scalability.
A well-thought-out strategy is crucial for successful integration. This includes pilot programs to test how generative AI performs in specific areas of your business, collaborative learning to build a collective understanding of generative AI, iterative development to continually improve the AI systems, and scalable infrastructure to meet AI's growing demands.
Here are the key steps to develop an implementation plan:
- Pilot programs: Begin with small pilot projects to test how generative AI performs in specific areas of your business.
- Collaborative learning: Promote teamwork across departments to build a collective understanding of generative AI.
- Iterative development: Use an iterative approach to continually improve the AI systems based on feedback and changing business needs.
- Scalable infrastructure: Invest in scalable infrastructure to meet AI's growing demands as it becomes more embedded in your operations.
Data privacy and security should be a top priority when integrating generative AI, including encryption and access controls to meet regulatory standards.
Scaling and Governance
As your generative AI project grows, it's essential to scale its impact and capabilities. This means expanding its reach and influence to achieve more significant business goals.
Scaling up requires careful planning and execution, but it's a crucial step in realizing the full potential of your gen AI project. In fact, as your project matures, it's time to expand its impact and capabilities.
For more insights, see: Generative Ai for Project Management
Maintaining strong governance practices is vital as you scale up your gen AI capabilities. This ensures that your expanding capabilities remain ethical, compliant, and aligned with your business objectives. Tools like watsonx.governance can help you achieve this.
By prioritizing governance, you can prevent potential issues and ensure a smooth scaling process.
A fresh viewpoint: Generative Ai Governance
Best Practices and Considerations
Implementing generative AI in your business requires careful planning to ensure a smooth adoption process. Three crucial aspects to consider include thoughtful planning, strategic decision-making, and careful planning.
To ensure a successful generative AI adoption, focus on the top 4 best practices: Setting up ethics boards or committees to oversee and guide the ethical implications of GenAI applications.Making sure your AI decision-making processes are transparent.Staying updated on legal and regulatory requirements related to AI.Establishing systems to continuously monitor generative AI business applications.
By implementing these best practices, you'll be well on your way to navigating the complex landscape of generative AI in business.
Discover more: Use Generative Ai
Key Limitations
Generative AI models often have difficulty adapting to specific business needs, especially when it comes to understanding industry-specific jargon.
This limitation can lead to significant challenges in implementing AI initiatives that directly support broader business aims.
Companies may need to invest in data curation and extensive model fine-tuning to enhance the adaptability of generative AI models to different business contexts.
Substantial customization is often required, which can be time-consuming and costly, necessitating collaboration with AI experts and focusing on domain-specific training data.
Generative AI, despite its remarkable capabilities, is also packed with major limitations that businesses should be mindful of.
Key Considerations
As you consider implementing generative AI in your business, it's essential to think about the key considerations that will impact its success.
Maintaining strong governance practices is crucial as you scale your gen AI capabilities. Tools like watsonx.governance can help ensure that your expanding gen AI capabilities remain ethical, compliant, and aligned with your business objectives.
Implementing generative AI demands thoughtful planning, and three crucial aspects to ensure a smooth adoption process are worth noting.
Developing an implementation plan is vital, and starting with small pilot projects to test generative AI's performance in specific areas of your business is a great idea.
To ensure a successful generative AI adoption, it's crucial to navigate the ethical and legal landscape of AI use. This includes setting up ethics boards, making AI decision-making processes transparent, staying updated on legal and regulatory requirements, and continuously monitoring generative AI business applications.
Here are some key considerations to keep in mind:
- Pilot programs can help test generative AI's performance in specific areas of your business.
- Ethics boards can oversee and guide the ethical implications of GenAI applications.
- Transparency practices are essential to ensure stakeholders understand how AI is being used.
- Regulatory adherence is crucial to ensure compliance with industry standards.
- Scalable infrastructure is necessary to meet AI's growing demands as it becomes more embedded in your operations.
Technical Considerations
Conducting a thorough technical feasibility assessment is crucial before integrating generative AI into existing systems. This evaluation will determine the complexity of the integration and whether custom model development is necessary.
You'll need to consider whether pre-trained models can be utilized, which can save time and resources. This is especially important for businesses with limited AI expertise.
Determining the computational requirements for different use cases is also essential, as it will help you plan for the necessary infrastructure and resources.
Technical Feasibility Assessment
Evaluating the complexity of integrating generative AI into existing systems is crucial for successful adoption. This involves determining whether custom model development is necessary or if pre-trained models can be utilized.
To assess the technical feasibility of generative AI, you need to consider the computational requirements for different use cases. This will help you understand the resources needed to implement and maintain the technology.
A thorough technical feasibility assessment will also help you evaluate the complexity of integrating generative AI into your existing systems. This includes assessing the data landscape and evaluating data quality.
Bringing in data engineers to assess data quality and set up data preparation processes is essential for successful gen AI implementation. They will use their expertise to evaluate data quality and establish robust data preparation processes.
Custom model development may be necessary if pre-trained models are not suitable for your specific use case. This will require significant resources and expertise, so it's essential to carefully evaluate the need for custom development.
You might like: Generative Ai Healthcare Use Cases
Technical Assistance
Technical assistance is a crucial aspect of leveraging AI technology. Voice synthesis is a key area where AI can greatly enhance user experiences in voice-activated devices and applications.
With AI-powered voice synthesis, businesses can create realistic human-like voices for voice assistants. This technology has the potential to benefit various industries, including audiobook narration.
Audiobook narration is another area where AI can make a significant impact. Generative AI can convert text into natural-sounding audio for producing audiobooks, podcasts, and voiceovers.
Publishing organizations can utilize this technology for audiobook production, expanding their content offerings for readers.
For more insights, see: Roundhill Generative Ai & Technology Etf
Deployment and Maintenance
Deploying generative AI models marks the transition from development to real-world application.
You can deploy generative AI models through APIs that enable seamless integration with your business applications and processes. This allows developers to focus on other aspects of the project.
To simplify the deployment process, tools like Fixie are available. They make it easier to build, share, and deploy AI applications that can integrate into your business processes seamlessly.
Monitoring and maintaining generative AI models is crucial to ensure they perform effectively. Developers rely on a suite of evaluation tools to track model performance in real time.
For prompt engineering, experimentation, and observability, developers leverage no-code and low-code tooling alongside tracking tools and platforms like WhyLabs’ LangKit.
You might like: Foundations and Applications of Generative Ai
Security and Compliance
Security and compliance are crucial when implementing generative AI in your business. Incorporating strong security measures protects sensitive data used by generative AI models.
Compliance with data privacy regulations is essential, especially for industries with strict requirements. This ensures that your business is not only secure but also law-abiding.
A well-structured generative AI tech stack is necessary for data-driven decision-making, automation, and innovation. This enables your business to stay competitive and improve efficiency.
Generative AI development companies have emerged as significant players, catalyzing the adoption of this innovative technology. They serve as both the architects and enablers of a future where businesses can leverage the potential of generative AI.
As these companies continue to innovate and refine generative AI solutions, they empower organizations to thrive in the digital age and lead the way.
Consider reading: How Are Companies Using Generative Ai
Sources
- https://appinventiv.com/blog/generative-ai-for-business/
- https://professional.uchicago.edu/find-your-fit/courses/generative-ai-business
- https://www.ibm.com/blog/step-by-step-guide-generative-ai-for-your-business/
- https://www.neurond.com/blog/generative-ai-in-business
- https://www.leewayhertz.com/generative-ai-in-business/
Featured Images: pexels.com