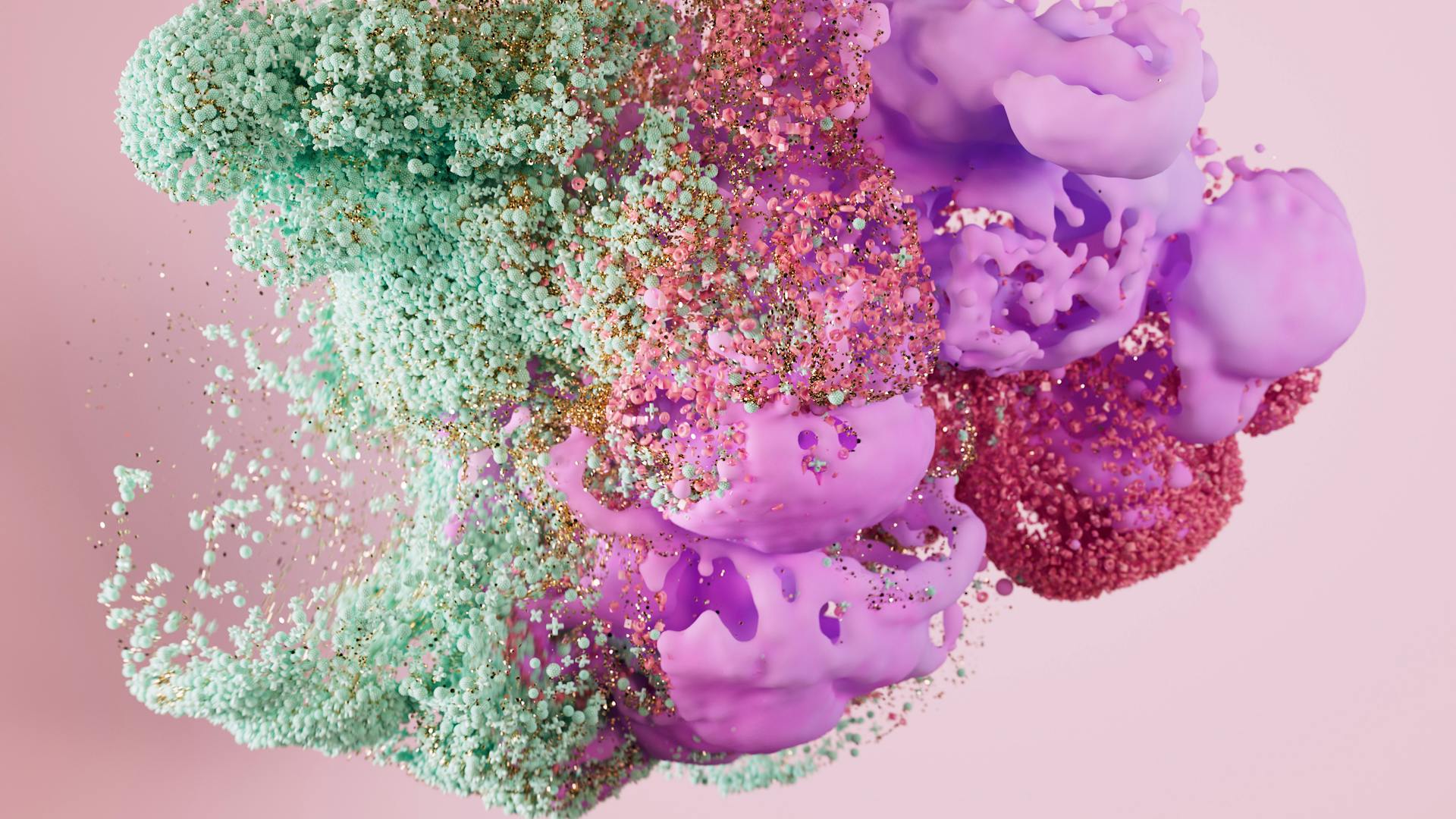
Generative AI in insurance has the potential to revolutionize the industry by automating routine tasks and improving customer experiences.
Generative AI can automate tasks such as data entry, claims processing, and policy administration, freeing up human resources for more complex and high-value tasks.
According to a report, AI-driven automation can reduce claims processing time by up to 75%.
With generative AI, insurance companies can also create personalized customer experiences by generating tailored policy quotes and recommendations.
A study found that 80% of customers are more likely to purchase a policy from an insurer that offers personalized recommendations.
Expand your knowledge: Generative Ai Customer Experience
What is Generative AI in Insurance?
Generative AI in insurance is a game-changer that enables machines to generate new content, data, or information similar to that produced by humans. Unlike traditional AI systems, generative AI uses advanced algorithms and deep learning models to create original and dynamic outputs.
It plays a crucial role in redefining various aspects of the insurance industry, from customer interactions to risk assessment and fraud detection. Generative AI introduces a new paradigm in the insurance landscape, offering unparalleled opportunities for innovation and growth.
You might like: New Generative Ai
Generative AI's ability to create original content opens doors to novel applications pertinent to the insurance industry. This includes predictive modeling, which enables the creation of risk scenarios that empower insurers to formulate preemptive strategies for proactive risk management.
Generative AI's capability to create personalized content enables insurers to offer tailor-made insurance policies and experiences, fostering stronger relationships with customers.
You might enjoy: Generative Ai Content Creation
Benefits and Use Cases
Generative AI in insurance is revolutionizing the way companies operate, interact with customers, and manage risks. The technology has a wide range of use cases, offering innovative solutions to longstanding challenges and driving disruptive changes.
Four main objectives have emerged among insurers exploring generative AI: summarizing policies, documents, and other unstructured content; synthesizing summarizations to create new content; answering questions based on learned information; and translating between natural languages and computer code.
Streamlined claims processing is one of the use cases gaining traction across insurance subsectors, enhancing workforce productivity, cost savings, and efficiencies. Loss prevention and control is another use case, enabling workforce productivity and generating new revenue streams.
For your interest: Google Announces New Generative Ai Search Capabilities for Doctors
Generative AI has a wide range of use cases in the insurance industry, including product personalization, agent assistance, optimized underwriting and pricing, customized group plans, and improved member engagement.
Here are some key benefits of implementing generative AI in the insurance industry:
- Streamlined claims processing to enhance workforce productivity, enable cost savings and efficiencies
- Loss prevention and control to enhance workforce productivity and generate new revenue streams
- Product personalization to generate new revenue streams
- Agent assistance to enhance workforce productivity
- Optimized underwriting and pricing to enhance workforce productivity and enable cost savings and efficiencies
- Customized group plans to generate new revenue streams and enhance workforce productivity
- Improved member engagement to generate new revenue streams and enhance workforce productivity
Key Features and Technologies
Generative AI in insurance is all about creating systems that can enhance decision-making and automate routine tasks. These systems integrate data aggregation technologies to compile and analyze information from diverse sources.
One key feature of generative AI in insurance is its ability to personalize customer interactions. Machine learning algorithms are employed to tailor insurance policies to individual client profiles, ensuring that each client's unique needs and risk factors are considered.
Generative AI plays a pivotal role in transforming tasks like claim processing and policy documentation. It's also used to improve accuracy in risk assessments and optimize operational efficiency.
The comprehensive data foundation supported by data aggregation technologies allows for predictive analytics capabilities. This enables the forecasting of risks and claims trends that inform strategic decisions.
Machine learning algorithms are used to tailor insurance policies to individual client profiles, ensuring that each client's unique needs and risk factors are considered. This is a major area of focus for generative AI in insurance.
Worth a look: Chatgpt Openai's Generative Ai Chatbot Can Be Used for
Implementation and Challenges
Implementing generative AI in insurance requires a strategic and phased approach. This involves defining specific objectives, such as risk assessment, customer profiling, or automated underwriting.
To achieve these objectives, insurers need to ensure they have high-quality and diverse datasets available, which are essential for training generative AI models. Generative Adversarial Networks (GANs), Variational Autoencoders (VAEs), or other relevant models may be suitable for different applications.
Implementing generative AI also requires integrating it seamlessly into existing insurance systems and workflows, which may involve collaboration with IT teams. Strong security protocols must be established to protect sensitive data, and compliance with data privacy regulations must be ensured.
A feedback loop should be established to facilitate ongoing enhancements and improvements, collecting input from users and stakeholders to pinpoint areas for improvement. Insurers must also scale implementation gradually, beginning with pilot projects or specific use cases to assess the impact of generative AI.
Here are the key steps to implementing generative AI in insurance:
- Define objectives: Identify specific use cases and objectives for implementing generative AI.
- Data preparation: Ensure high-quality and diverse datasets are available.
- Select appropriate models: Choose generative AI models based on the use case.
- Training and validation: Train the chosen model using historical data and validate its performance.
- Integration with existing systems: Integrate generative AI seamlessly into existing insurance systems and workflows.
- Security and privacy considerations: Establish strong security protocols to protect sensitive data and ensure compliance with data privacy regulations.
- Continuous monitoring and improvement: Implement mechanisms for ongoing monitoring of generative AI models and regularly update and retrain them.
- Collaboration with domain experts: Work closely with insurance domain experts to interpret model outputs and validate the relevance of generated content.
- User training and acceptance: Train end-users to leverage generative AI outputs effectively and foster acceptance and understanding of the technology among stakeholders.
- Scale gradually: Begin with pilot projects or specific use cases to assess the impact of generative AI and gradually scale implementation based on successful outcomes and feedback.
- Feedback loop: Establish a feedback loop to facilitate ongoing enhancements and improvements.
Claims Processing
Claims processing is a crucial aspect of the insurance industry, and generative AI is revolutionizing the way claims are handled. Generative AI automates claims processing by extracting and validating data from claim documents, reducing manual efforts and processing time.
Automated claims processing ensures faster and more accurate claim settlements, improving customer satisfaction and operational efficiency. For example, property insurers can utilize generative AI to automatically process claims for damages caused by natural disasters.
The claims processing workflow involves several key steps, including claims reporting, assessment, investigation, decision making, and claims payment. Generative AI plays a crucial role in each of these steps, from automating data extraction to facilitating decision making.
Here's a breakdown of the key steps involved in claims processing and the role of generative AI:
Generative AI streamlines the entire claims settlement process, reducing turnaround time and minimizing errors. Faster and more accurate claims settlements lead to higher customer satisfaction and improved operational efficiency for insurers.
Claims management and fraud detection is another area where generative AI has taken a more important role. Generative AI helps to speed up the identification of fraud by checking past cases and incidents, removing the need for employees to check claims manually.
You might enjoy: Generative Ai Fraud Detection
Risk Modeling
Risk modeling with generative AI is a game-changer for insurers. It allows them to simulate various risk scenarios and predict potential future risks, helping them optimize risk management strategies and make informed decisions.
Generative AI models can forecast weather-related risks in different regions, enabling proactive measures to minimize losses. This is especially useful for property and casualty insurers.
Predictive analytics powered by generative AI provides valuable insights into emerging risks and market trends. By leveraging this technology, insurers can identify potential risks and adjust their strategies accordingly.
Here are some key benefits of using generative AI for risk modeling:
Autoregressive models are particularly well-suited for generating sequential data, such as time-series data on insurance premiums, claims, or customer interactions. These models can help insurers predict future trends, identify anomalies within the data, and make data-driven decisions for business strategies.
Implementing: Key Steps
Implementing generative AI in the insurance industry involves a strategic and phased approach. To get started, insurers should define specific objectives for implementing generative AI, such as risk assessment, customer profiling, or automated underwriting.
Before implementing generative AI, it's essential to ensure high-quality and diverse datasets are available. Generative AI models require robust training data to effectively learn and generate meaningful outputs.
To drive better business outcomes, insurers must effectively integrate generative AI into their existing technology infrastructure and processes. This involves establishing a multidisciplinary team of businesspeople, IT specialists, and data scientists to adapt generative AI solutions to the unique requirements of the organization.
Generative AI can automate claims processing by extracting and validating data from claim documents, reducing manual efforts and processing time. This can lead to faster and more accurate claim settlements, improving customer satisfaction and operational efficiency.
To implement generative AI, insurers should follow these key steps:
By following these key steps and considering the challenges and opportunities of implementing generative AI, insurers can unlock the full potential of this technology and drive innovation in the industry.
Industry Challenges
The biggest challenge in implementing a new system is the high cost of training and retraining employees to use it.
Legacy systems can be a major obstacle, making it difficult to integrate new technology with existing infrastructure.
One of the main reasons for system failure is poor planning and execution, which can lead to a lack of user adoption.
Many companies struggle to find the right talent to manage and maintain their new systems, which can be a significant challenge.
The average cost of a failed IT project can be as high as $1 million, making it crucial to get it right the first time.
A fresh viewpoint: Android 12 New Features
Frequently Asked Questions
What is the size of the generative AI in the insurance market?
The global generative AI in insurance market size was approximately $615 million in 2023, with a projected growth to over $14 billion by 2034.
What is an example of AI in insurance?
Swiss Re uses AI to streamline life insurance underwriting, improving risk assessments and pricing strategies. This example showcases AI's potential to enhance insurance processes and outcomes
Sources
- https://www.ey.com/en_us/insights/insurance/how-insurers-can-leverage-the-power-of-generative-ai
- https://www.deloitte.com/global/en/Industries/financial-services/perspectives/generative-ai-in-insurance.html
- https://www.leewayhertz.com/generative-ai-in-insurance/
- https://www.sas.com/sas/webinars/global-insurance-circle/unleash-the-power-of-generative-ai-in-insurance-us.html
- https://www.oliverwyman.com/our-expertise/insights/2023/nov/generative-ai-transform-insurance-asia.html
Featured Images: pexels.com