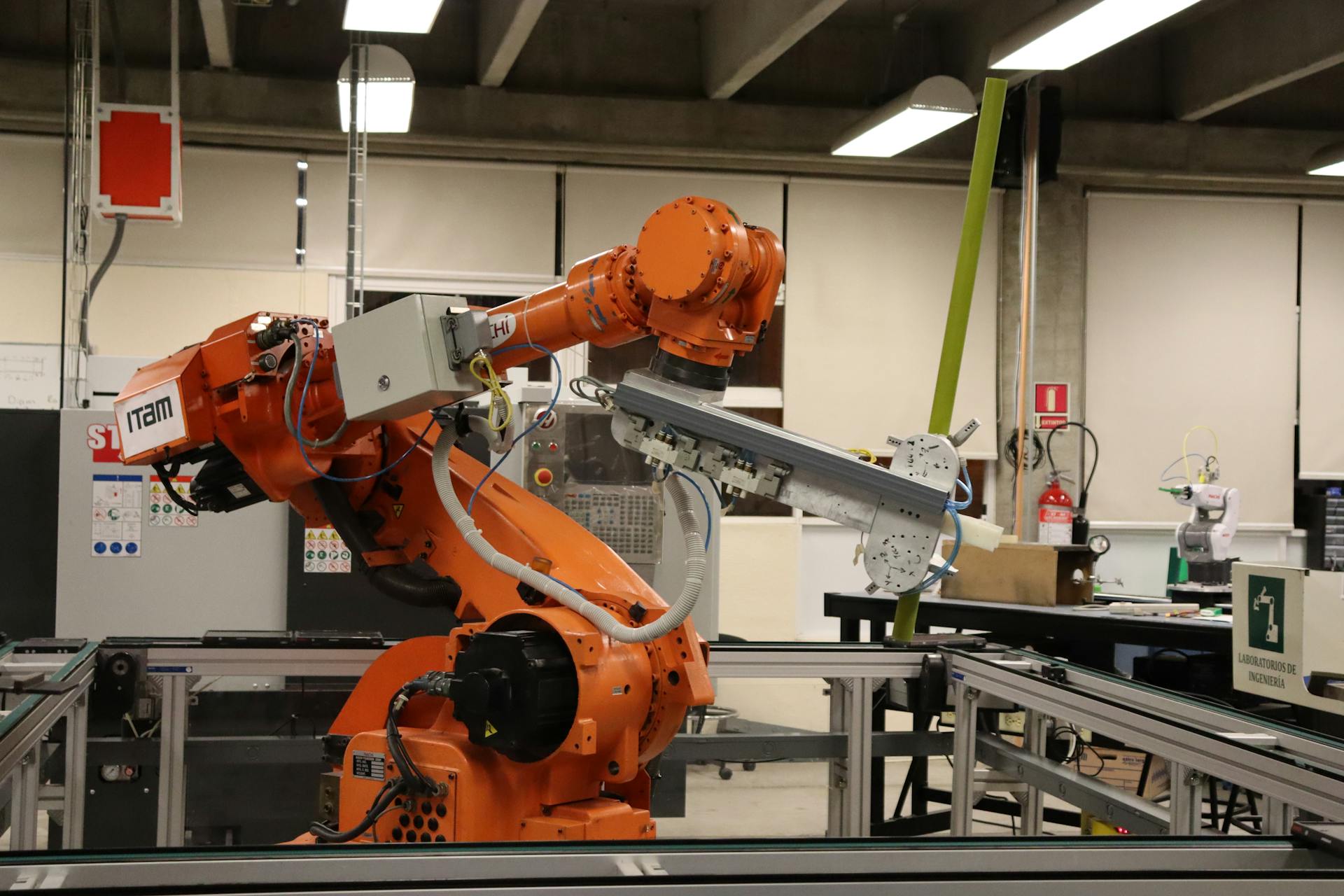
Human in the Loop RPA is a game-changer for businesses, allowing them to automate repetitive tasks while still maintaining the human touch.
By integrating human judgment with robotic process automation (RPA), organizations can improve accuracy and efficiency.
This hybrid approach enables employees to focus on high-value tasks, freeing up time for more strategic work.
According to research, Human in the Loop RPA can increase productivity by up to 30% and reduce errors by 25%.
Suggestion: Generative Ai vs Rpa
What Is Human in the Loop RPA?
Human in the loop RPA is a system that allows humans to interact with it, providing direct feedback to improve predictions. This interaction is key to refining outcomes.
The concept of HITL (Human-in-the-Loop) is defined as systems that allow humans to give direct feedback to a model for predictions below a certain level of confidence. This threshold can be set based on the acceptable level of error in the system.
To be "in the loop" means actively participating and having a direct influence on the decision-making process. This implies that humans are integrated into the system, contributing their expertise and judgment to improve outcomes.
Suggestion: Can Generative Ai Replace Humans
In practice, HITL systems require humans to determine what level of confidence is acceptable for the process. This can be a low threshold, allowing more wrong predictions to "slip through", or a high threshold, requiring more manual intervention.
Here are some key aspects of HITL systems:
- Allow humans to give direct feedback to a model for predictions below a certain level of confidence.
- Require humans to determine the acceptable level of confidence for the process.
- Integrate humans into the system to contribute expertise and judgment to improve outcomes.
What Are the Benefits of?
Human input enhances the system's ability to handle ambiguous or evolving situations, as seen in a 2018 Stanford study where a Human-in-the-Loop AI model worked better than AI on its own or human doctors on their own.
Humans can provide real-time adjustments and insights, making the system more adaptable, which is beneficial in areas like healthcare where systems can't always be automated.
Involving human judgment clarifies decision-making processes, increasing transparency in AI systems. This is especially important in areas where AI decision-making can be opaque.
Human oversight helps address ethical concerns and biases in AI decision-making, which is crucial in areas like product recommendations where customers' preferences are taken into account.
Here are some of the key benefits of human-in-the-loop machine learning:
- Improved Decision-Making: Human input enhances the system's ability to handle ambiguous or evolving situations.
- Adaptability: Humans can provide real-time adjustments and insights, making the system more adaptable.
- Ethical Considerations: Human oversight helps address ethical concerns and biases in AI decision-making.
- Complex Problem Solving: Humans contribute problem-solving skills to handle unique or novel situations.
By blending the best of manual and automated systems, human-in-the-loop approaches allow for human intervention when beneficial, resulting in speed and efficiency gains in certain scenarios.
Improving Accuracy and Reliability
Human-in-the-loop RPA can significantly improve the accuracy of rare datasets, especially in languages spoken by only a few thousand people. By involving human judgment, accuracy is improved while maintaining human-level standards of work.
A Human-In-The-Loop (HITL) approach is particularly useful in industries where precision is crucial, such as manufacturing critical parts for vehicles or airplanes. In these situations, human monitoring is necessary to ensure human-level precision is always achieved.
Human review of machine learning workflows can also improve the quality of models by identifying errors made by the models, assessing training data, and reducing data bias. This leads to more accurate predictions and improved reliability.
The benefits of a HITL model include enhanced transparency, increased trust, speed and efficiency, problem-solving superiority, and greater flexibility. These advantages can be seen in various industries, including healthcare, where a HITL AI model has been shown to work better than AI alone or human doctors alone.
Human-in-the-loop systems can also rectify errors in machine learning, making them more accurate and reliable. This is particularly important in applications such as autonomous driving, medical diagnosis, and object recognition, where accuracy is critical.
Here's an interesting read: Bootstrap Method Machine Learning
Improved Reliability and Accuracy
Human-centered AI offers a more dependable solution compared to relying solely on algorithms. This is because human expertise can validate data and results, improving the overall reliability and accuracy of AI solutions.
By interjecting critical information into the process, humans can provide continual feedback during the training process, resulting in ML models that make more accurate predictions. This is especially important in situations where a single mistake can have serious consequences.
In fact, a 2018 Stanford study found that a Human-in-the-Loop AI model works better than AI on its own or human doctors on their own. This highlights the importance of combining human expertise with machine learning.
Human-in-the-Loop (HITL) systems can improve accuracy by rectifying errors made by machines. This is particularly useful in situations where there is a lack of data, as HITL can help ensure the accuracy of rare data sets.
Here are some benefits of a HITL model:
- Enhanced Transparency: Involving human judgment clarifies decision-making processes.
- Increased Trust: Human participation builds confidence in automated systems.
- Speed and Efficiency: Humans can make rapid decisions, outpacing full automation in certain scenarios.
- Problem-Solving Superiority: People apply real-world knowledge to issues beyond AI’s current capabilities.
- Greater Flexibility: The HITL approach blends the best of manual and automated systems, allowing human intervention when beneficial.
What's the Difference Between and ?
The difference between human-in-the-loop and human-out-of-the-loop systems is a crucial one when it comes to improving accuracy and reliability. Human-in-the-loop systems benefit from human expertise, providing input and guidance to machine learning algorithms.
In contrast, human-out-of-the-loop systems operate independently without human intervention. They rely solely on pre-programmed rules and algorithms, lacking the adaptability and nuanced understanding that human input provides.
Human-in-the-loop systems are a collaborative effort between humans and machines, resulting in more informed decisions. This approach is especially valuable in situations where complex judgment is required.
Human-out-of-the-loop systems, on the other hand, can be prone to errors due to their reliance on pre-programmed rules. This can lead to oversimplification of complex issues, resulting in suboptimal outcomes.
On a similar theme: Human-in-the-loop
Hand in Hand
Human in the loop RPA is all about collaboration between humans and machines. It's a continuous feedback loop where humans and machines work together to achieve better results.
Machine learning algorithms can fail to understand improper formatting of sentences, making human inputs essential for analyzing data and providing the best results. This is especially true when it comes to understanding the subtleties of human language and emotions.
Human-in-the-Loop (HITL) systems are beneficial in processing every question, ensuring that machine learning algorithms are tuned accurately and customers receive thoughtful responses from AI chatbots. This is achieved through constant human intervention, which reviews and corrects the language used by the AI.
Here are some key benefits of HITL systems:
The beauty of HITL systems is that they don't require you to be a computer scientist to use them. With automation software built around the concept of human in the loop, you can expect near-perfect performance from the start and reasonable results.
Challenges and Considerations
Implementing a human in the loop RPA requires careful consideration of several challenges. One of the main hurdles is getting a dataset with enough training data.
To overcome this, you need to find people who can operate effectively in the environment. Integrating AI into existing workflows can also be a significant challenge.
Designers should consider human cognitive constraints and inherent biases to build more intuitive and responsive automation tools. This involves recognizing humans as active agents rather than mere recipients of machine outputs.
Challenges When Implementing
Implementing a human in the loop is a complex process, and one of the biggest challenges is getting a dataset with enough training data to make AI work effectively.
Having a robust dataset is crucial for AI to learn and adapt to new situations. Unfortunately, gathering sufficient data can be a time-consuming and resource-intensive task.
In many cases, finding people who can operate effectively in the environment where AI is being implemented can be a significant challenge. This can be due to a lack of technical expertise or the need for specialized skills.
Integrating AI into existing workflows can also be a major hurdle, requiring significant changes to existing processes and systems. This can be a daunting task, especially for organizations with legacy systems.
Design Considerations
Designing automation tools with human needs in mind is crucial. This means recognizing humans as active agents rather than mere recipients of machine outputs.
To build effective automation tools, designers should consider human cognitive constraints and inherent biases. This will help create tools that are more intuitive and responsive.
Consider reading: Ai in Software Test Automation
A human-centric approach involves understanding how humans process information and make decisions. It's essential to design systems that work in harmony with human capabilities.
An effective Human-in-the-Loop system allows multiple people to have input on a process at any given time. The person providing feedback is likely to be the one responsible for making the final decision.
Designers should prioritize flexibility and adaptability in their systems to accommodate human input and feedback. This will enable humans to make informed decisions and take ownership of the process.
If this caught your attention, see: Automated Decisions
Future Directions and Scalability
Human in the loop RPA systems can be challenging to scale up, but there are ways to make them more efficient. One approach is to use an interpretable machine learning model, which can provide a high-level summary of the data, making it easier for humans to handle large amounts of data.
To make human in the loop systems more scalable, online learning algorithms can be used. These algorithms allow models to adapt to new conditions and customers, enabling humans to improve the accuracy and reliability of machine learning models.
Data labeling is a crucial aspect of human in the loop systems, as it allows machines to understand data by creating a training set that the machine learning algorithm can learn from. This is particularly important in areas such as autonomous driving, medical diagnosis, and object recognition.
For your interest: Optimize Machine Learning Algorithm
Future Directions for Systems and ML
Human-in-the-loop systems are crucial for future applications, particularly in areas like autonomous driving, medical diagnosis, and object recognition, where unstructured data requires human input for proper labeling.
Effective human-in-the-loop systems involve data labeling, which is a challenging but essential task for machine learning algorithms to learn from representative examples.
The limitations of fully automated systems can be addressed by combining human intelligence with machine efficiency, creating a powerful synergy that can handle complex, dynamic, and context-dependent scenarios more effectively.
To establish trust in AI systems, we need to find ways to regulate robots and ensure their reliability, which is becoming increasingly important as our societies rely more on these technologies.
Human-in-the-loop computing is the future of machine learning because it allows machines to learn from humans, enabling them to understand and adapt to new situations more effectively.
A fresh viewpoint: What Is Human in the Loop
Scalability
Scalability is a crucial aspect of any human-in-the-loop system. It's often difficult and expensive to scale up a HITL system to handle large amounts of data, which can lead to decreased performance.
One way to make a HITL system more scalable is to use an interpretable machine learning model. This type of model provides a high-level summary of the data, making it easier for humans to handle large amounts of data.
Using an online learning algorithm is another approach to scalability. This type of algorithm allows models to adapt to new conditions and customers or end-users, improving the accuracy and reliability of machine learning models.
There is no one-size-fits-all answer to scalability, as it depends on the particulars of the situation. However, with proper design, a HITL system can be scaled up to meet the needs of an organization.
Sources
Featured Images: pexels.com