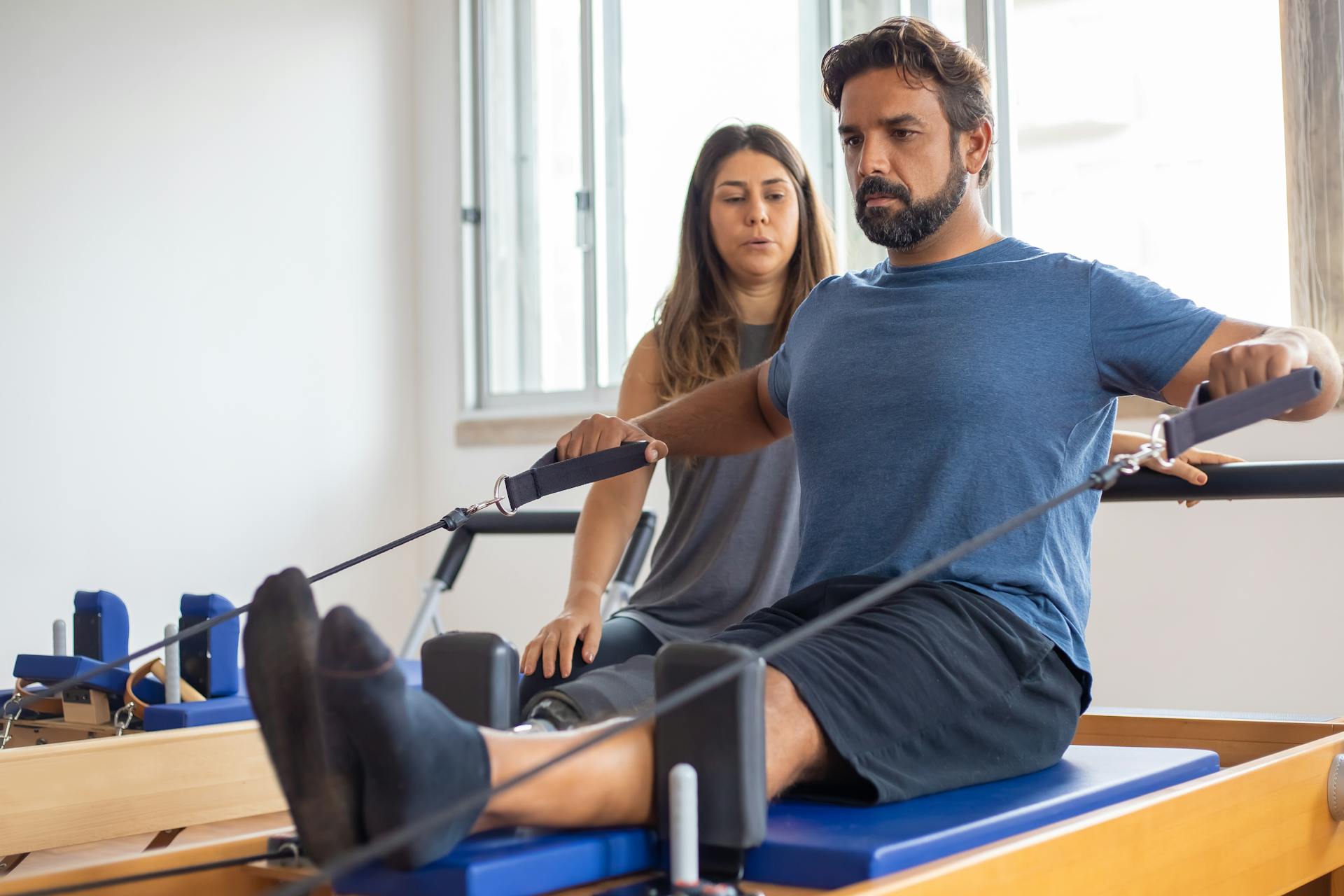
The Human-in-the-Loop revolution is transforming machine learning outcomes in profound ways. By integrating human judgment and oversight into the AI development process, organizations can significantly improve the accuracy and reliability of their models.
Machine learning models can be notoriously prone to errors and biases, with a study revealing that up to 60% of AI decisions are incorrect. Human-in-the-Loop helps mitigate these issues by allowing humans to review and correct model outputs.
By leveraging human expertise and feedback, organizations can reduce the risk of model failure and improve overall performance. For instance, a company using Human-in-the-Loop to optimize its image classification model saw a 25% reduction in errors.
Human-in-the-Loop also enables organizations to address complex tasks that are difficult or impossible for AI to accomplish alone. By combining human and machine intelligence, organizations can tackle challenges that were previously insurmountable.
Recommended read: Learning with Errors
What Is It?
Human-in-the-loop is a concept that refers to the involvement of human experts in the machine learning process. These experts can include data annotators, QAs, data scientists, ML engineers, product and project managers, data analysts, and domain experts.
The human-in-the-loop approach provides valuable feedback that helps applications using artificial intelligence improve faster and more effectively. This is because human experts can investigate market needs, evaluate the appropriateness of a model, and establish ownership of a product.
In the narrow sense, human-in-the-loop refers to the involvement of specialists who help reduce errors and supervise the learning process of machines. However, in a broader sense, it can also refer to other experts involved in planning stages of model development.
You can use automation techniques to mark data pieces, but you still need a data labeler to assign meaning to these labels. This is because a sophisticated model will need at least some form of annotation from human experts.
Here are some examples of how human-in-the-loop benefits AI:
- AI chatbots improve conversations with the help of human agents in the back end.
- Machine learning algorithms are tuned accurately with the HITL concept.
- Customers don't receive curt responses from AI chatbots with Human-in-the-loop.
In essence, human-in-the-loop is about making the work of people easier by automating tedious tasks, while still relying on human experts to make creative decisions.
Benefits and Importance
Human-in-the-loop (HITL) is a game-changer in machine learning, allowing models to learn from human expertise and adapt to changing user preferences and real-world scenarios.
By incorporating human input and feedback, HITL enhances the accuracy and reliability of ML models, reducing the risk of biases and errors.
Human involvement in the ML process promotes fairness and equity in ML systems, making them more transparent and explainable.
The inclusion of human feedback and collaboration fosters trust among end-users, increasing their confidence in ML systems.
Here are the benefits of HITL in a nutshell:
With HITL, ML models can learn from human behavior and adapt to evolving real-world conditions, making them more effective and reliable.
By leveraging human expertise, HITL helps identify and correct biases, ensuring that ML systems are fair and equitable.
In the learning process, algorithms perform better with constant human intervention, known as HITL, which reviews and corrects the language and responses of AI systems.
Human inputs are required for analyzing the data and providing the best results, making HITL an essential component of ML development.
By working together, human-in-the-loop and machine learning can produce more accurate and reliable results, improving the overall performance of ML systems.
Collaborative Machine Learning
Collaborative machine learning is all about working together with humans to improve the accuracy and effectiveness of machine learning models. This approach is essential for navigating complex and nuanced tasks that require human judgment and contextual understanding.
Human inputs are required for analyzing data and providing the best results. Machines can learn almost everything, but providing accurate results is not possible without humans monitoring the information.
For more insights, see: Can Generative Ai Replace Humans
Incorporating human feedback into the machine learning pipeline allows models to adapt and evolve with changing user preferences and real-world scenarios. This is especially important in areas like chatbots, where machine learning reflects and updates the pattern of human conversations.
Machine learning algorithms can fail to understand the improper formatting of sentences, but HITL is beneficial in processing every question. By integrating the human element, we empower ML systems to navigate the complexities and nuances that often challenge purely algorithmic approaches.
Here are some key benefits of collaborative machine learning:
- The machine can make smart decisions with human understanding and inputs.
- HITL ensures the AI will connect with people in a natural way and understand what they are asking and respond accordingly.
- Machine learning algorithms are generally known as ‘Black boxes’, HITL rectifies the machine errors with human intelligence.
Improving Machine Learning Outcomes
Human-in-the-loop inputs and feedback are essential for improving machine-learning outcomes. This approach makes machine learning or computer vision models smarter with continuous human feedback and inputs.
With human-in-the-loop, machine learning algorithms are more effectively trained, tested, tuned, and validated. This leads to better results, improving accuracy and identifying objects in images or videos more confidently.
The human-in-the-loop approach is broader than active learning, encompassing everything from labeling datasets to providing continuous feedback to the algorithmic model. This makes it a more comprehensive solution for improving machine learning outcomes.
Here's an interesting read: Mlops Continuous Delivery and Automation Pipelines in Machine Learning
Human-in-the-loop chatbots rectify both human errors and machine errors by combining humans and machines. This results in better performance and more accurate answers.
Incorporating human domain experts into the machine learning process is crucial for designing a model that can give you a competitive advantage in the market. This is evident in the Nasdaq discovery tool that aimed to improve the management of trading activities.
Here are some benefits of human-in-the-loop for improving machine learning outcomes:
- Improves accuracy and identifies objects in images or videos more confidently
- Rectifies both human errors and machine errors by combining humans and machines
- Results in better performance and more accurate answers
- Requires human domain experts for designing a model that can give you a competitive advantage in the market
Implementation and Workflow
Human-in-the-loop technology is designed to work in harmony with human agents, recognizing that AI algorithms can struggle to respond to complex customer questions.
In difficult situations, human agents are essential to implement necessary actions.
Artificial intelligence can answer customer inquiries instantly, but human intervention is necessary to resolve more intricate issues.
Human-in-the-loop technology understands user intent and responds accordingly, making it a valuable tool for customer support.
Action Implementation
In difficult situations, humans are essential to implement necessary actions. This is because machine learning algorithms can struggle to respond to a customer's question.
Artificial intelligence can answer instantly, but human agents are needed to resolve complex issues. This highlights the importance of a seamless integration between AI and human agents.
Human agents can understand the user intent and respond accordingly, as seen in situations where machine learning algorithms are unable to provide a solution. This collaboration between AI and humans is crucial for delivering excellent customer service.
By working together, AI and human agents can provide a more comprehensive and effective support experience for customers.
Workflow Drawbacks
Human-in-the-loop systems can be slow and cumbersome, which can hinder productivity.
Using HITL processes can lead to human errors going unnoticed, impacting a model's performance and outputs negatively.
Humans can't work as quickly as computer vision models, making it essential to balance human involvement with machine-based processes.
Once people are deeply involved in the training process, it can take more time than if humans weren't involved at all.
This highlights the need to strike a balance between human oversight and machine efficiency in workflow implementation.
Employment Opportunities
Human-in-the-loop is not just about augmenting the capabilities of machines, it's also about creating new job opportunities for humans. In fact, the Indian market has seen significant growth thanks to data annotators who are working alongside machines.
The jobs created by human-in-the-loop are not just any jobs, they're better, more interesting, and ambitious ones. This concept is a perfect example of how technology can be harnessed to create a brighter future for people.
In some cases, a human expert is placed outside the loop and serves as an arbitrator, ensuring that machine-made decisions are accurate and fair. This is especially important in situations where a machine makes a judgment error.
The Canadian visa application process is a great example of this, where human officers are still available to address any issues with machine-made decisions.
Suggestion: Automated Decisions
Machine Learning and AI
Machine learning and AI are powerful tools, but they can benefit from human input and feedback. This is where human-in-the-loop (HITL) comes in, allowing machines to learn from humans and improve their performance.
Machine learning models can fail to understand improper sentence formatting, but HITL is beneficial in processing every question. Machines can learn almost everything, but providing accurate results is not possible without humans monitoring the information.
Human inputs are required for analyzing data and providing the best results. AI chatbots continuously improve the conversation with the help of human agents in the back end. Machine learning algorithms are tuned accurately with the HITL concept.
The machine can make smart decisions with human understanding and inputs. HITL ensures the AI will connect with people in a natural way and understand what they are asking and respond accordingly. Machine learning algorithms are generally known as 'Black boxes', HITL rectifies the machine errors with human intelligence.
Here are some key benefits of HITL in machine learning and AI:
- Improves accuracy and identifies objects in images or videos more confidently
- Produces better results, thanks to human-in-the-loop feedback
- Rectifies errors in machine learning, making AI more effective
- Allows machines to learn from humans and improve their performance
In the medical field, a 2018 Stanford study found that AI models performed better with human-in-the-loop inputs and feedback compared to when an AI model worked unsupervised or when human data scientists worked on the same datasets without automated AI-based support.
Computer Vision and Models
Encord is a collaborative, active learning suite of solutions for computer vision that can also be used for human-in-the-loop (HITL) processes.
The platform provides AI-assisted labeling, model training, and diagnostics, making it easier to accelerate computer vision model training and development.
With Encord, you can use a wide range of sectors, including healthcare, manufacturing, utilities, and smart cities, to annotate human pose estimation videos and accelerate their computer vision model development.
Encord Active is an open-source computer vision toolkit, and an Annotator Training Module that helps teams implement human-in-the-loop iterative training processes.
Encord's active learning platform is a comprehensive AI-assisted platform for collaboratively annotating data, orchestrating active learning pipelines, fixing dataset errors, and diagnosing model errors & biases.
You can try Encord for free today to see its capabilities firsthand.
Encord Data Engine accelerates every step of taking your model into production by streamlining fragmented workflows, annotation tools, and Notebooks for building AI applications.
Here's an interesting read: Active Learning (machine Learning)
Active Learning and Compatibility
Active learning and human-in-the-loop are similar in many ways, and both play an important roles in training computer vision and other algorithmically-generated models.
They are compatible, and you can use both approaches in the same project. According to Example 7, "Active learning and human-in-the-loop are similar in many ways, and both play an important role in training computer vision and other algorithmically-generated models."
The main difference between active learning and human-in-the-loop is that the human-in-the-loop approach is broader, encompassing everything from active learning to labeling datasets and providing continuous feedback to the algorithmic model.
Here's a breakdown of the key differences:
By understanding the differences between active learning and human-in-the-loop, you can choose the approach that best suits your project's needs.
Frequently Asked Questions
What is an example of a human-on-the-loop?
A human-in-the-loop example is a system where a person reviews and approves or rejects decisions made by a machine learning algorithm. This can be as simple as a manager approving a hiring recommendation or a doctor reviewing a diagnosis suggested by AI.
Sources
- https://cloud.google.com/discover/human-in-the-loop
- https://hdsr.mitpress.mit.edu/pub/812vijgg
- https://www.ometrics.com/blog/e-commerce-chatbots/what-is-human-in-the-loophitl-in-ai-chatbots/
- https://labelyourdata.com/articles/human-in-the-loop-in-machine-learning
- https://encord.com/blog/human-in-the-loop-ai/
Featured Images: pexels.com