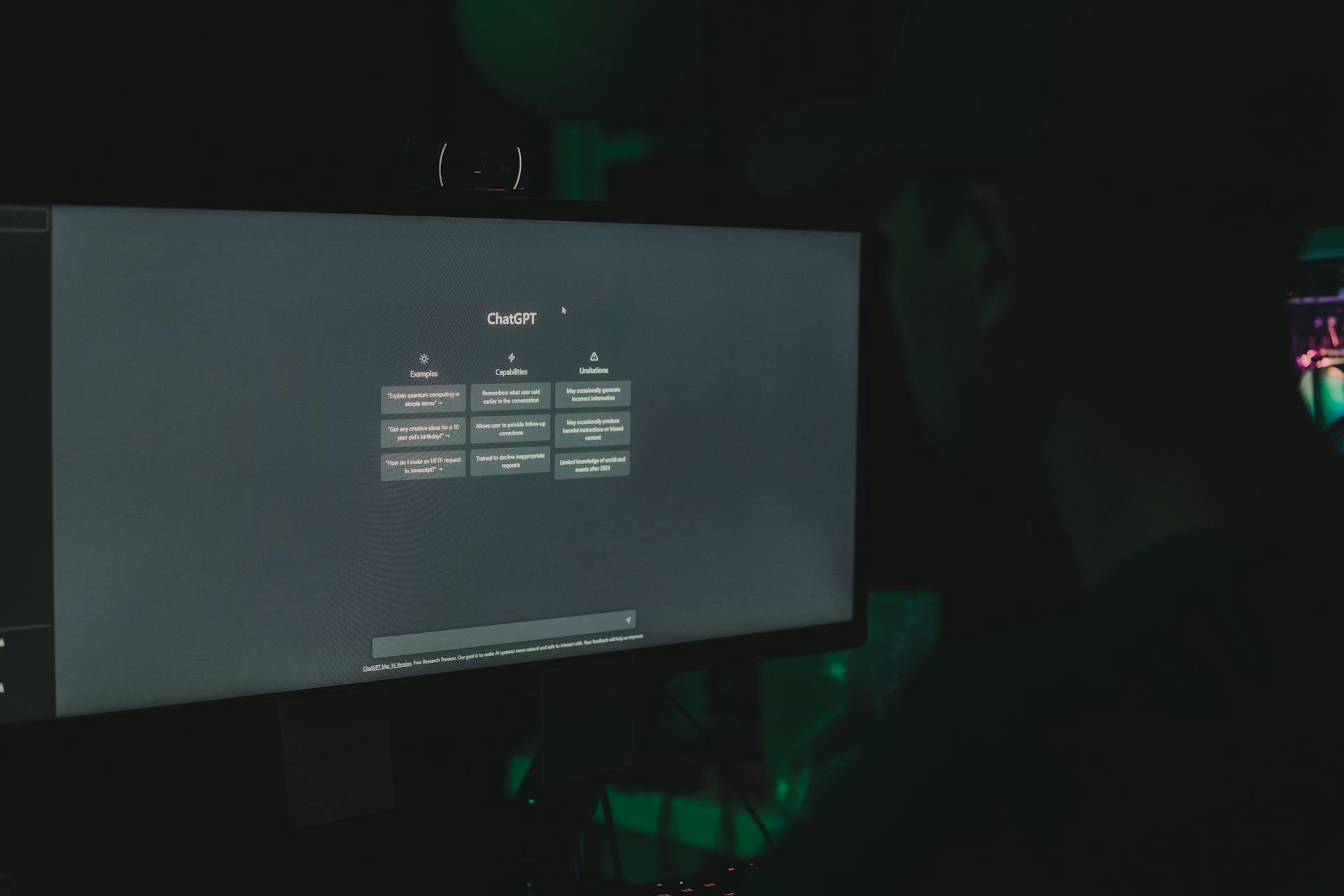
ChatGPT Generative AI is a type of artificial intelligence that can generate human-like text based on the input it receives.
It's a massive language model that uses a vast dataset to learn patterns and relationships in language, allowing it to produce coherent and context-specific text.
The model is trained on a large corpus of text data, which enables it to understand the nuances of language and generate text that is often indistinguishable from human-written content.
This technology has the potential to revolutionize the way we interact with machines and access information.
Related reading: Velocity Model Prediciton Using Generative Ai
What is ChatGPT?
ChatGPT is a technology that can create new and original content like art, music, and writing.
It's a type of generative AI that uses large language models to produce novel content based on input prompts.
ChatGPT is trained using large datasets and deep-learning algorithms that learn the underlying structures and patterns present in the data.
This allows it to generate unique and creative results, often producing new and original content that mimics human creativity.
Expand your knowledge: Generative Ai for Content Creation
Generative AI models like ChatGPT are capable of producing a wide range of outputs, including images, video, code, music, design, translation, question answering, and text.
By leveraging the power of artificial intelligence, ChatGPT is able to produce novel content based on what it has learned from existing examples on the internet.
How It Works
Generative AI models learn from data to create new content, discovering patterns and relationships in the data they're trained on.
They use neural networks inspired by the human brain to learn these patterns, and can then generate new data that aligns with what they've learned.
Generative AI models can refine their outputs through iterative training processes, analyzing the relationships within given data and adjusting their parameters to minimize the difference between desired and generated outputs.
These models can create content across a dynamic range of domains, leveraging advanced techniques like generative adversarial networks (GANs), large language models, variational autoencoder models (VAEs), and transformers.
Expand your knowledge: Generative Ai Content
From a user perspective, generative AI often starts with an initial prompt to guide content generation, followed by an iterative back-and-forth process exploring and refining variations.
Generative AI uses a computing process known as deep learning to analyze patterns in large sets of data and replicate those patterns to create new data that mimics human-generated data.
It employs neural networks, a type of machine learning process loosely inspired by the way the human brain processes, interprets, and learns from information over time.
Text-based generative AI models rely heavily on natural language processing (NLP) and are trained on huge amounts of text, such as all of Wikipedia.
Through this training, they learn how words relate to each other, grammar rules, and writing styles found in the data, enabling them to perform tasks like predicting the next word in a sentence and creating writing samples.
The success of a language model depends on its training data, with more data and varied data leading to better performance in creating clear, relevant, and correct text.
Generative AI employs various models to enable the creation of new and original content, including generative adversarial networks (GANs), variational autoencoders (VAEs), and large language models (LLMs).
Transformer-based models, like ChatGPT-4, have made significant advancements in generative AI possible, enabling the technology to train with billions of pages of data and text without requiring labeled data in advance.
For your interest: Generative Ai with Large Language Models
Types of ChatGPT Models
ChatGPT models are trained on vast volumes of textual data, leveraging transformer architectures to understand and generate coherent text based on given prompts.
They use knowledge from textbooks and websites to social media posts to generate human-like responses, making them ideal for language-related applications, from chatbots and content creation to translation.
Generative AI models like ChatGPT employ various types, including Generative Adversarial Networks (GANs), Variational Autoencoders (VAEs), and Large Language Models (LLMs).
GANs consist of a generator and a discriminator, with the generator producing synthetic data and the discriminator evaluating its authenticity.
VAEs are neural networks that learn to encode and decode data, enabling the generation of new data by sampling points in the latent space.
ChatGPT models are based on transformer architectures, which process data by making a token from given prompts to discover relationships between them.
This allows them to predict what word comes next in a sequence of words, simulating human speech and enabling realistic conversations and question answering.
Broaden your view: Generative Ai Human Creativity and Art Google Scholar
The advancements of large language models, with billions or possibly trillions of parameters, have created the next era in generative AI that we see today.
These models can be trained with billions of pages of data and text without labeling the data in advance, resulting in more depth and understanding of the data.
Benefits and Risks
Generative AI can significantly impact a company's bottom line by automating tasks and focusing employees' time on strategic objectives, resulting in lower labor costs and greater operational efficiency.
Manual oversight and scrutiny of generative AI models are highly important to ensure accuracy and quality.
Efficiency gains from generative AI can free up resources for more important tasks, providing additional insights into business processes.
You might like: Why Is Controlling the Output of Generative Ai Systems Important
Benefits of
Generative AI can help automate specific tasks, focusing employees' time and energy on more important strategic objectives.
Automating tasks can result in lower labor costs and greater operational efficiency.
Manual oversight and scrutiny of generative AI models remain highly important to ensure accuracy and quality.
Generative AI tools can help professionals and content creators with idea creation, content planning and scheduling, search engine optimization, marketing, audience engagement, research, and editing.
Risks of Use

Using generative AI can have serious consequences, such as the loss of human jobs as AI takes over tasks that were previously done by humans.
Gartner analyst's predictions suggest that generative AI will significantly impact culture and society, and one of the risks is the spread of misinformation and propaganda through AI-generated content.
Generative AI can be used to create fake news, deepfakes, and other forms of manipulated media that can be difficult to distinguish from real information.
This can lead to a breakdown in trust in institutions and the media, and can even contribute to the erosion of democracy.
The risk of job displacement is a significant concern, as AI takes over tasks that were previously done by humans, potentially leading to widespread unemployment.
As AI becomes more advanced, it's likely that we'll see a shift in the way we work and live, and it's essential to be aware of the potential risks and consequences of this shift.
Readers also liked: Generative Ai Risks
Limitations and Future
Generative AI is still in its early stages, and its limitations are being explored. The technology is rapidly evolving, with companies like OpenAI pushing the envelope with higher-parameter models and photorealistic AI video.
As of 2024, OpenAI released its OpenAI o1 model, which prioritizes complex coding and math processes over speed. This is a significant development in the field of generative AI.
The future of generative AI holds much promise, with the potential for natural language commands to control systems. However, this also raises concerns about the risks and opportunities that come with such technology.
Explore further: Roundhill Generative Ai & Technology Etf
Limitations of
Generative AI has a lot of potential, but it also has some limits. These models depend on the data they learn from, which can be biased or incomplete. This can lead to wrong or biased results.
There's a risk of using this technology in harmful ways, like creating misleading content. This raises ethical issues that need to be addressed.
To fix these problems, we need to think carefully about the ethical effects of generative AI. We need to create strong rules for using data and keep researching ways to reduce bias.
If we use generative AI responsibly, we can make the most of what it can do while managing its risks. Just like with any new technology, we should be careful but hopeful.
Future of
Generative AI is poised to play a central role in various industries, with many organizations already establishing guidelines for its acceptable use.
As more businesses embrace digitization and automation, generative AI will continue to shape the chips market, according to Deloitte's 2024 Tech Predictions.
The capabilities of generative AI have already proven valuable in areas such as content creation, software development, medicine, productivity, and business transformation.
Companies are experimenting with generative AI tools, but leaders need to keep tabs on regulations, use cases, and potential risks.
Generative AI companies are pushing the envelope by creating higher-parameter models and photorealistic AI video.
The future of generative AI might bring the use of natural language commands to both find information and command the system.
OpenAI released its OpenAI o1 model in 2024, which trades speed for complex coding and math processes.
Agentic AI, where teams of generative AI "agents" work together to solve multi-step, multivariable problems, is often cited as the future of the technology.
Curious to learn more? Check out: Generative Ai and the Future of Work in America
Applications and Examples
Generative AI is making waves in various industries, and its applications are vast. Google has integrated generative AI into Search with AI Overviews.
Artists are using generative AI to create new art styles and forms of creative writing. DALL-E 3, an AI model by OpenAI, can create images and artwork from text prompts.
Designers are taking advantage of generative AI to look at many design options and create special user interfaces. Apple released Apple Intelligence, a mix of proprietary AI models and OpenAI technology, in iOS 18, iPadOS 18, and macOS Sequoia.
Here's an interesting read: Create with Confidence Using Generative Ai
Generative AI is also being used in music, with musicians trying AI-generated tunes, rhythms, and harmonies. GitHub Copilot, an AI-powered coding tool, suggests code completions within development environments.
Here are some examples of generative AI models and products:
- GPT-4: OpenAI's flagship generative AI model comes in a variety of sizes.
- ChatGPT: An AI language chatbot developed by OpenAI that can answer questions and generate human-like responses from text prompts.
- DALL-E 3: An AI model by OpenAI that can create images and artwork from text prompts.
- Google Gemini: Google's generative AI chatbot and rival to ChatGPT.
- Claude 3.5: Anthropic's AI model, Claude, offers a 200,000 token context window.
- Midjourney: A gen AI model that interprets text prompts to produce images and artwork.
- GitHub Copilot: An AI-powered coding tool that suggests code completions within development environments.
- Llama 3: Meta's open-source large language model that can be used to create conversational AI models.
- Grok: A generative AI venture by Elon Musk that debuted its first model, Grok, in November 2023.
Examples of
Generative AI has exploded in recent years, with tech giants like Google, Microsoft, and Meta launching their own tools to capitalize on the technology's rapid uptake. Google's AI Overviews integrate generative AI into Search, while Microsoft incorporates the Copilot AI into PCs.
Generative AI models like OpenAI's GPT-4 and DALL-E 3 can create text, images, and artwork from text prompts. ChatGPT, another OpenAI model, can answer questions and generate human-like responses from text prompts.
Google's Gemini, previously known as Bard, is a generative AI chatbot that can answer questions and generate text from prompts. It's trained on the PaLM large language model.
Some notable examples of generative AI models and products include:
- GPT-4: OpenAI's flagship generative AI model
- ChatGPT: An AI language chatbot developed by OpenAI
- DALL-E 3: An AI model by OpenAI that can create images and artwork from text prompts
- Google Gemini: Google's generative AI chatbot and rival to ChatGPT
- Claude 3.5: Anthropic's AI model that offers a 200,000 token context window
- Midjourney: A gen AI model that interprets text prompts to produce images and artwork
- GitHub Copilot: An AI-powered coding tool that suggests code completions
- Llama 3: Meta's open-source large language model that can be used to create conversational AI models
- Grok: A new generative AI venture from Elon Musk, with its first model debuting in November 2023
Generative AI can speed up the content generation process by automatically generating drafts, designs or compositions that professionals can refine and improve.
Transforming Art and Design
Artists are using AI to create new art styles and forms of creative writing, which can lead to innovative ideas and stunning visuals. Generative AI is changing the creative world by providing artists with new tools to explore their art.
Artists can work with AI to come up with unique music, including AI-generated tunes, rhythms, and harmonies. This expands what is possible in music.
Designers are taking advantage of generative AI to look at many design options and create special user interfaces. The fast ability to experiment and show ideas allows for more creativity.
Designers can use generative AI to improve product designs, leading to amazing new designs.
Discover more: Generative Ai for Music
Comparison and Overview
Generative AI is distinct from traditional AI, which often refers to machine learning that predicts outcomes or rearranges information. It's like the difference between a calculator and a creative artist - one just follows rules, while the other generates new content.
In traditional AI, systems are designed for specific tasks, making them examples of "narrow AI". This is in contrast to the concept of "general AI", which refers to computer systems that possess human-like intelligence and autonomy.
On a similar theme: How Are Modern Generative Ai Systems Improving User Interaction
Traditional vs.
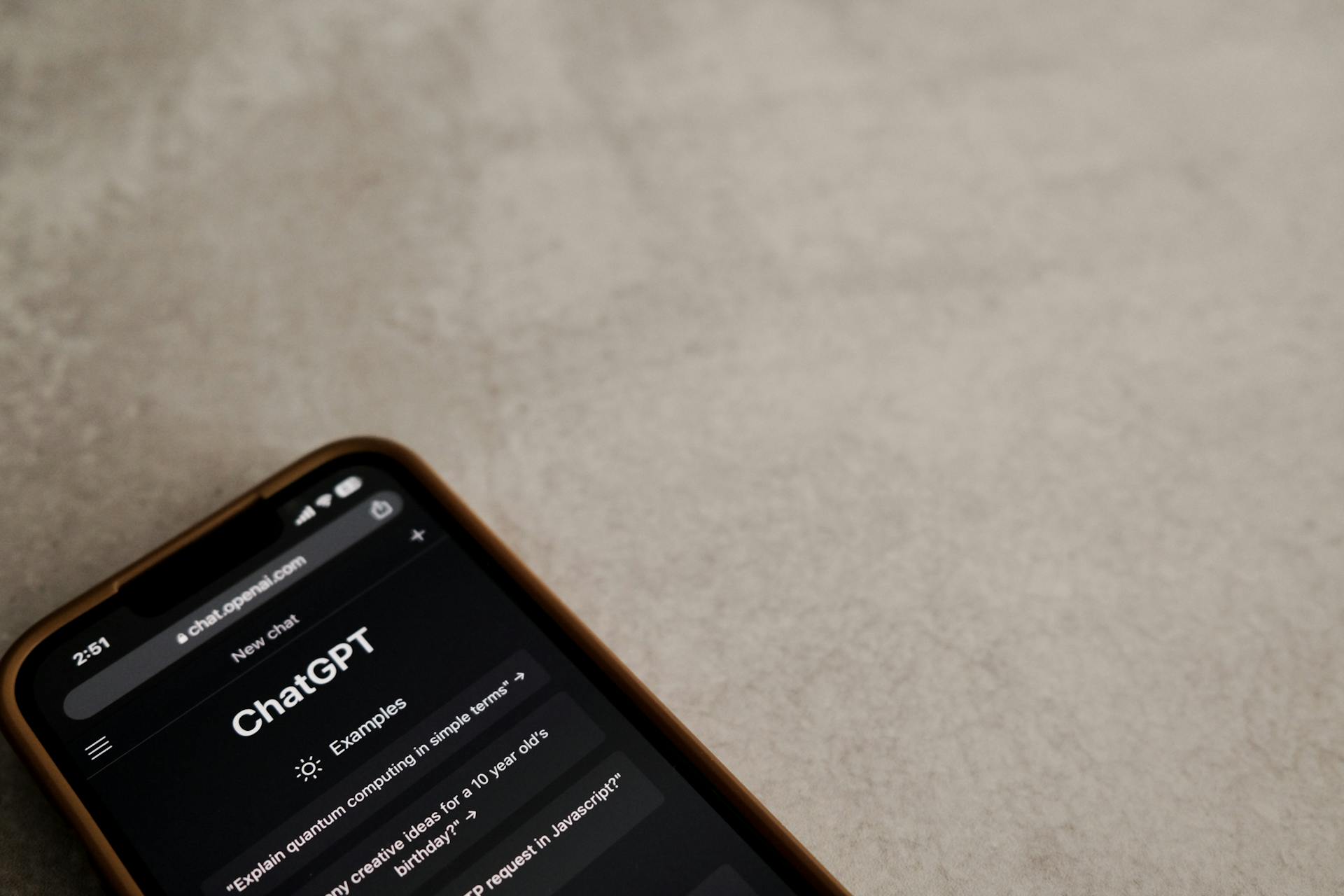
Traditional AI is essentially machine learning, which predicts outcomes or rearranges information.
In many cases, traditional AI refers to machine learning.
The term "traditional AI" is often used to describe machine learning, which is a specific type of AI.
Machine learning is a form of AI that's been around for a while, and it's used for a wide range of tasks.
Most current AI systems are examples of "narrow AI", which are designed for very specific tasks.
Traditional AI is different from generative AI, which generates new content based on training data not necessarily related to the input it receives.
Discover more: Gen Ai vs Ml
Google Gemini Overview
Google Gemini is a relatively new AI model that's worth learning about.
You can learn more about Gemini by reading TechRepublic's comprehensive Google Gemini cheat sheet.
Google Gemini is being compared to ChatGPT, with some wondering if it's better.
TechRepublic has written a comparison article, Google Gemini vs. ChatGPT: Is Gemini Better Than ChatGPT?.
Google Gemini is an AI model that's generating interest in the tech community.
You might enjoy: Geophysics Velocity Model Prediciton Using Generative Ai
Featured Images: pexels.com