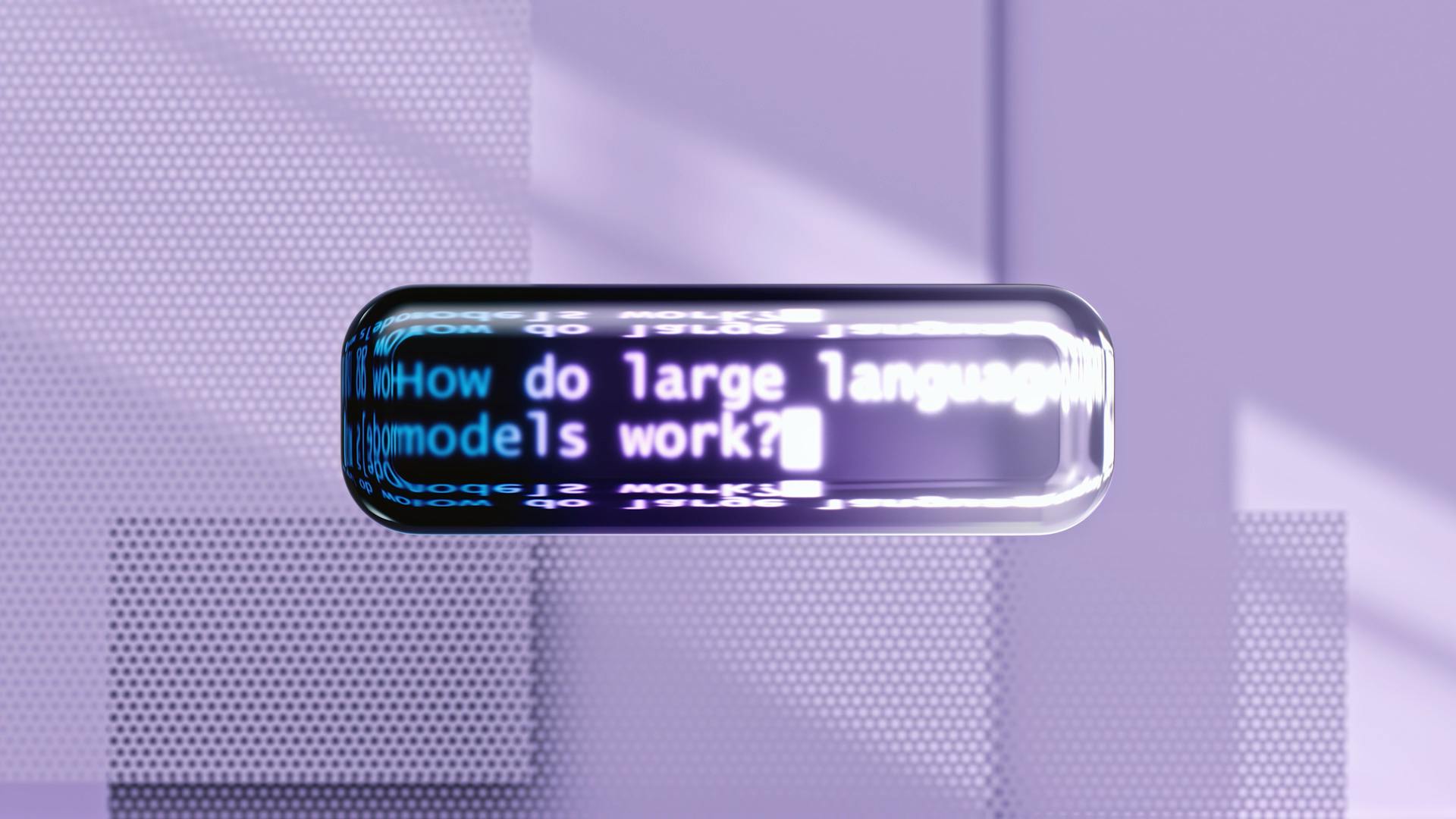
As we navigate the rapidly evolving landscape of generative AI, it's essential to acknowledge the potential risks that come with its increased use. Deepfakes, for instance, can be created with alarming accuracy, raising concerns about their potential misuse.
The consequences of deepfakes can be severe, including the spread of misinformation and the erosion of trust in institutions. In fact, a study found that 70% of people can't tell a deepfake from a real video.
Generative AI can also perpetuate existing biases, as algorithms often learn from and reflect the data they're trained on. This can result in AI systems that discriminate against certain groups, exacerbating social inequalities.
For your interest: The Economic Potential of Generative Ai
Risks and Concerns
Generative AI raises several concerns that must be addressed to ensure its safe and responsible use. Bias and stereotype amplification are significant risks, as AI models can perpetuate existing biases present in the training data, exacerbating societal inequalities and discrimination.
One major concern is the potential for generating fake or misleading content, which can be used to spread disinformation or deceive people. This can be particularly challenging for detecting and verifying digital media.
Generative AI also poses risks related to data privacy, including the potential for data privacy violations and the embedding of personally identifiable information (PII) in language models. Companies must ensure that PII isn't embedded in the language models and that it's easy to remove PII from these models in compliance with privacy laws.
Some of the specific risks associated with generative AI include biased data and/or outputs, model inversion/membership inference, and DoS attacks. These risks are not entirely new, but rather adaptations of existing issues in data management and system integrity.
Unknowns
Generative AI raises concerns about misuse and errors. Along with the benefits, there are limitations where legal frameworks have not caught up with technological developments.
The OECD works with governments to enable policies that ensure the ethical and responsible use of generative AI. This is crucial to mitigate the risks and ensure the technology benefits society.
Fake and misleading content is a significant concern. Generative AI can be used to create realistic-looking but entirely fabricated images or videos, which can be used to spread disinformation or deceive people.
Here's an interesting read: How Multimodal Used in Generative Ai
The potential for generating fake or misleading content poses challenges for the detection and verification of digital media. This is a major concern that must be considered carefully.
Risks stemming from prompt injection are a novel concern. This threat is unique to generative AI and directly exploits the non-deterministic nature and interactive, generative capabilities of AI models.
Prompt injection introduces entirely new vectors for attack that traditional security measures may not address. This represents a significant security concern.
In the near term, generative AI can exacerbate challenges as synthetic content with varying quality and accuracy proliferates in digital spaces. This can lead to a vicious cycle of synthetic content being used to train subsequent generative AI models.
Over the longer term, emergent behaviors such as increased agency, power-seeking, and pursuing hidden sub-goals to achieve a core objective might not align with human values and intent.
Expand your knowledge: Generative Ai Prompt Examples
Bias, Stereotype Amplification and Privacy Concerns
Bias, stereotype amplification, and privacy concerns are significant risks associated with generative AI. These risks can have far-reaching consequences, including perpetuating and amplifying societal inequalities and discrimination.
Generative AI models can inadvertently produce biased outputs if the training data contains biases, such as racial or gender stereotypes. For example, a language model trained on text data may reproduce personal details or confidential information, raising privacy concerns.
Bias can be found in data used for training LLMs outside the control of companies that use these language models for specific applications. Companies working on AI must have diverse leaders and subject matter experts to help identify unconscious bias in data and models.
Generative AI applications can exacerbate data and privacy risks, as they often store input information indefinitely and use it to train other models. This can contravene privacy regulations that restrict secondary uses of personal data.
Some of the key risks related to bias and stereotype amplification include:
- Model/data poisoning: This refers to the manipulation of training data or model behavior, which can lead to biased or inaccurate outputs.
- Bias in data and/or outputs: This can result in perpetuating and amplifying societal inequalities and discrimination.
- Model inversion/membership inference: This is a technique used to extract sensitive details from aggregated or anonymized data outputs, potentially leading to privacy concerns.
- DoS attacks: While not unique to GenAI, these attacks can still pose a threat to the integrity of AI systems.
To mitigate these risks, it's essential to have an effective AI governance strategy in place. This should involve data scientists, engineers, diversity and inclusion specialists, user experience designers, functional leaders, and product managers working together to ensure responsible AI development and deployment.
Intellectual Property and Liability
Generative AI raises intellectual property rights issues, particularly concerning unlicensed content in training data, potential copyright, patent, and trademark infringement of AI creations, and ownership of AI-generated works.
Several lawsuits were filed in the US against companies that allegedly trained their models on copyrighted data without authorisation, making decisions that will set legal precedents and impact the generative AI industry.
Companies must validate outputs from models until legal precedents provide clarity around IP and copyright challenges, as reputational and financial risks could be massive if one company's product is based on another company's intellectual property.
This could be a major concern for companies like banks and pharmaceutical companies, where a single mistake could have severe consequences.
If this caught your attention, see: Generative Ai Has an Intellectual Property Problem
Intellectual Property and Liability
Generative AI raises complex intellectual property rights issues, particularly concerning unlicensed content in training data.
In the US, several lawsuits have been filed against companies that allegedly trained their models on copyrighted data without authorization, which could set legal precedents impacting the industry.
Companies must validate outputs from generative AI models until legal precedents provide clarity around IP and copyright challenges.
Reputational and financial risks can be massive if a company's product is based on another company's intellectual property.
Lawsuits in the US have highlighted the issue of training ML models on copyrighted material without permission, which could have significant implications for the industry.
Here are some key risks to consider:
- Copyright infringement of AI creations
- Ownership of AI-generated works
- Unlicensed content in training data
These risks can be mitigated by validating outputs from generative AI models and seeking clarity on IP and copyright challenges through legal precedents.
Fair Approach
The FAIR-AIR approach is a detailed methodology for quantifying generative AI risks. It covers five vectors of GenAI risks, emphasizing the importance of understanding and managing Shadow AI, foundational large language model (LLM) development, hosting, third-party management, and adversarial threats.
This approach takes a strategic, financial perspective on risk, aligning with broader organizational and cybersecurity risk management practices. It's a framework that helps companies understand and manage the risks associated with using generative AI.
For your interest: Generative Ai and Knowledge Management
The FAIR philosophy is about conceiving risk as an uncertain event, the probability and consequences of which need to be measured. Risk is defined as the probability of a loss relative to an asset.
Threats enter this definition as factors influencing the probability of a loss, and it's essential to distinguish between threats and risk scenarios. Threats are the initial exploits or entry points that threat actors use to compromise the system, while risk scenarios include the business impacts and losses caused by such exploits.
The FAIR approach helps companies understand the relationship between the methods threat actors might use and the types of harm or compromise they can lead to. It's a framework that encourages a strategic, financial perspective on risk, which is essential for managing GenAI risks.
Job Market and Workforce
Generative AI is likely to transform labour markets and jobs, but exactly how is still uncertain and being debated among experts. This transformation could lead to humans performing tasks more efficiently and generating new creative possibilities.
Some jobs might be automated or eliminated, but generative AI could also transform existing jobs, requiring a shift in required skills. Businesses will need to help employees develop generative AI skills such as prompt engineering.
The future of work itself is changing, and companies that invest in preparing their workforce for the new roles created by generative AI applications will be better equipped to minimize negative impacts and prepare for growth.
Additional reading: How Generative Ai Will Transform Knowledge Work
Workforce Roles and Morale
Generative AI is changing the face of work, and it's not just about automating tasks. It's about transforming jobs and requiring new skills.
Businesses are already investing in preparing employees for the new roles created by generative AI applications. This includes developing skills like prompt engineering.
The pace of worker displacement and replacement has accelerated with the innovations in generative AI technologies. The future of work itself is changing, and companies that adapt will be the ones to thrive.
Explore further: New Generative Ai
Ethical companies are investing in preparing certain parts of the workforce for the new roles created by generative AI. This will not only minimize the negative impacts but also prepare companies for growth.
The truly existential ethical challenge for adoption of generative AI is its impact on organizational design, work, and individual workers. This requires a shift in thinking and a willingness to adapt.
You might like: Generative Ai Company
Internal Audit Leaders
Internal audit leaders need to design and adopt new audit methodologies to assess the risks that generative AI systems pose. This is because auditing will be a key governance mechanism to confirm that AI systems are designed and deployed in line with a company's goals.
To create a risk-based audit plan specific to generative AI, internal audit must understand the problem the company is trying to solve using GenAI. This is an important starting point because it's difficult and ineffectual to assess the risks that generative AI systems pose independent of the context in which they are deployed.
Internal audit leaders must adopt new forms of supervision and new skill sets to effectively assess the risks of generative AI systems.
Related reading: Why Is Controlling the Output of Generative Ai
Security and Compliance
Security and Compliance is a crucial aspect of managing generative AI risks. The way organizations choose to develop and utilize AI systems plays a significant role in defining their safety and security.
Chief Information Security Officers (CISOs) need to be aware of the increased risk of sophisticated phishing attacks, where generative AI can create custom lures in chats, videos, or live-generated "deep fake" video or audio, impersonating someone familiar or in a position of authority.
A nimble, collaborative, regulatory-and-response approach is emerging with generative AI, requiring a major adjustment for compliance officers to keep up with new regulations and stronger enforcement of existing regulations that apply to generative AI.
Here's a summary comparison of existing frameworks that can help organizations navigate security and compliance:
OWASP Top 10 for LLMs
The OWASP Top 10 for LLMs is a must-know for anyone working with Large Language Models. It outlines major security vulnerabilities specific to LLMs, offering practical prevention strategies.
Consider reading: Generative Ai and Llms for Dummies
OWASP Top 10 for LLMs addresses threats that are unique to LLMs like prompt injection and training data poisoning. This is a major concern, as these vulnerabilities can have serious consequences.
OWASP Top 10 for LLMs emphasizes robust and new security practices. Its strength comes from its specificity to LLM security, providing actionable recommendations.
Here are some of the key takeaways from OWASP Top 10 for LLMs:
The OWASP Top 10 for LLMs is a valuable resource for anyone looking to improve the security of their LLMs. By understanding these vulnerabilities and taking steps to prevent them, you can help keep your users and data safe.
Explore further: Are Llms Generative Ai
Compliance
As generative AI emerges, compliance officers must adapt to new regulations and stronger enforcement of existing ones. This requires a nimble and collaborative approach.
To stay on top of regulatory changes, compliance officers need to keep up with the latest developments. This includes understanding the specific regulations that apply to generative AI.
Intriguing read: Generative Ai Regulation
A major adjustment for compliance officers is required, as generative AI demands a new level of regulatory awareness. This is an area where experience and knowledge are crucial.
Ultimately, the success of generative AI depends on the people using it. Compliance officers must empower their teams to critically evaluate the outputs of generative AI models.
Sources
- https://oecd.ai/en/genai/issues/risks-and-unknowns
- https://www.elastic.co/blog/fair-generative-ai-risks-frameworks
- https://www.techtarget.com/searchenterpriseai/tip/Generative-AI-ethics-8-biggest-concerns
- https://www.icaew.com/technical/technology/artificial-intelligence/generative-ai-guide/risks-and-limitations
- https://www.pwc.com/us/en/tech-effect/ai-analytics/managing-generative-ai-risks.html
Featured Images: pexels.com