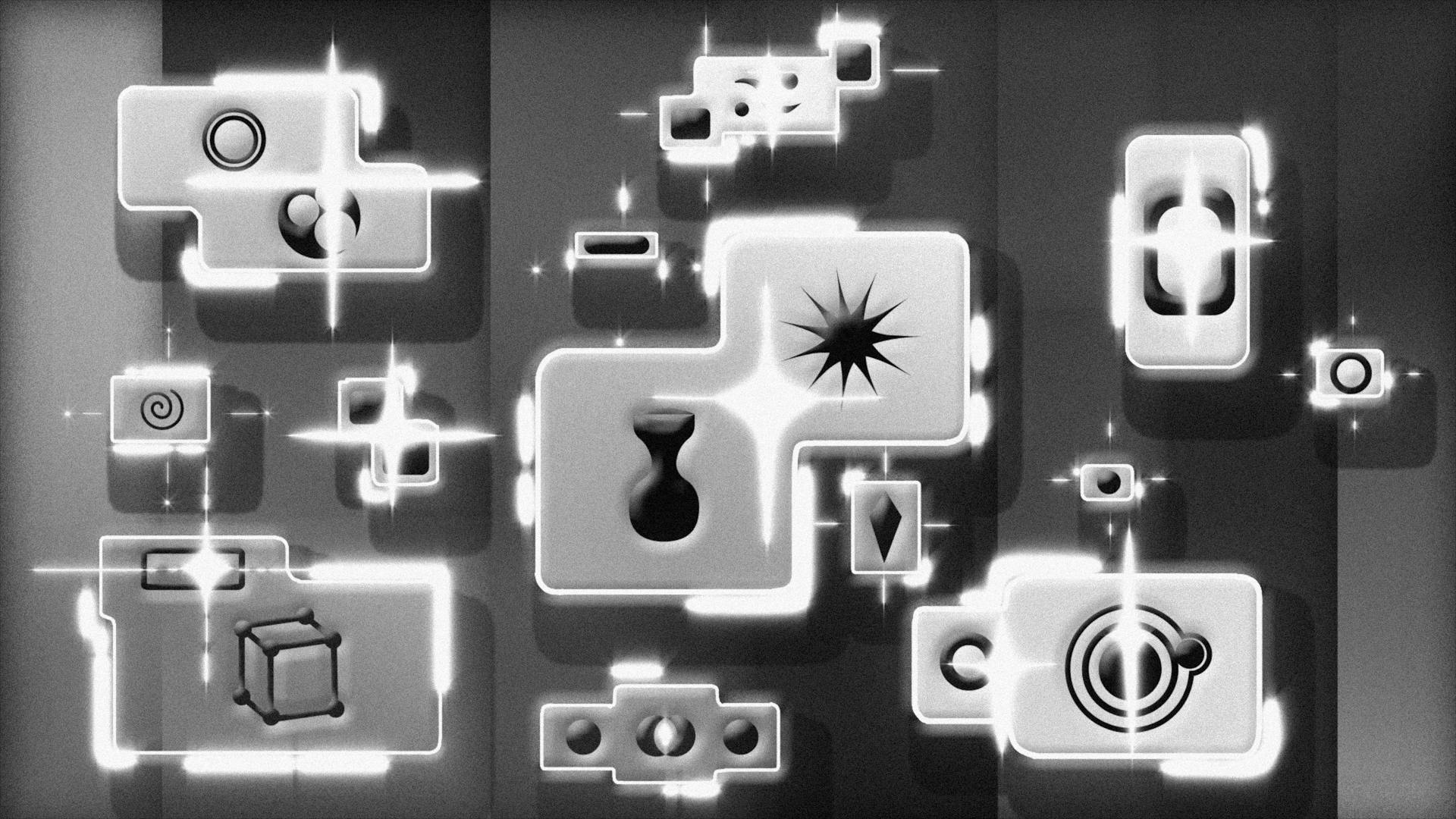
Generative AI is being touted as a revolutionary technology, but is it living up to the hype? According to a recent study, 70% of companies are already using or planning to use generative AI in the next two years.
The reality is that generative AI is still a developing field, and its limitations are often glossed over in the excitement. For example, a study found that 60% of AI-generated content is of "low quality" and requires significant human editing.
Despite these limitations, generative AI has shown promise in certain areas, such as content creation and data analysis. For instance, a company used generative AI to create a dataset of 10 million images, which was then used to train a model that could generate new images.
You might like: Chatgpt Openai's Generative Ai Chatbot Can Be Used for
Separating Hype from Progress
Separating hype from progress is crucial when evaluating AI-powered drug discovery companies. According to a recent study, entire data rooms with timelines and explanations are available for review and benchmarking, making it easier for analysts and journalists to do their research.
To avoid the financial hype, it's essential to focus on measurable results and experimental validation. Companies should receive funding gradually, based on the milestones they achieve, rather than relying on promises and big names.
Here are some simple questions to help evaluate AI-powered drug discovery companies and estimate their hype-to-results ratios:
- How many of your internally discovered drugs are in Phase I, Phase II, and Phase III?
- Did any of your pharma partnerships progress into Phase I, II, and III, and how long did it take?
- How many preclinical candidates (PCCs) did you nominate in a single year and in total?
- How long, on average, does it take you to nominate a PCC, and how much does it cost?
- What was the level of novelty of the targets and what is the level of novelty of the molecules?
- How long, on average, does it take you to go into clinical trials with an AI-discovered target?
- Did you in-license any of your drugs from other companies, repurpose them, or discover them internally?
- How many pharma companies use your AI software to discover drugs, and how many users do you have? How many renew every year?
- What was your revenue last year, and what was the revenue growth year over year?
- How much money have you raised, in how many rounds, and what is the valuation of your last round?
Is Generative AI Overhyped?
Generative AI is having a marked, measurable impact on industries and disciplines, but it's essential to separate hype from progress. IBM distinguished engineer Chris Hay believes we're in the corrective phase after a period of rampant enthusiasm.
The media often conflates generative AI with narrower applications like LLM-powered chatbots, which might not be equipped to solve every problem that enterprises face. This conflation can lead to exaggerated expectations and disappointment.
A list of simple questions can help evaluate the hype-to-results ratio of AI-powered companies:
- How many of your internally discovered drugs are in Phase I, Phase II, and Phase III?
- Did any of your pharma partnerships progress into Phase I, II, and III, and how long did it take?
- How many preclinical candidates (PCCs) did you nominate in a single year and in total?
- How long, on average, does it take you to nominate a PCC, and how much does it cost?
- What was the level of novelty of the targets and what is the level of novelty of the molecules?
- How long, on average, does it take you to go into clinical trials with an AI-discovered target?
- Did you in-license any of your drugs from other companies, repurpose them, or discover them internally?
- How many pharma companies use your AI software to discover drugs, and how many users do you have? How many renew every year?
- What was your revenue last year, and what was the revenue growth year over year?
- How much money have you raised, in how many rounds, and what is the valuation of your last round?
Unfortunately, most journalists, analysts, and investors rarely ask these questions, even though the answers can be summarized in one small table or figure.
Token Subsidies
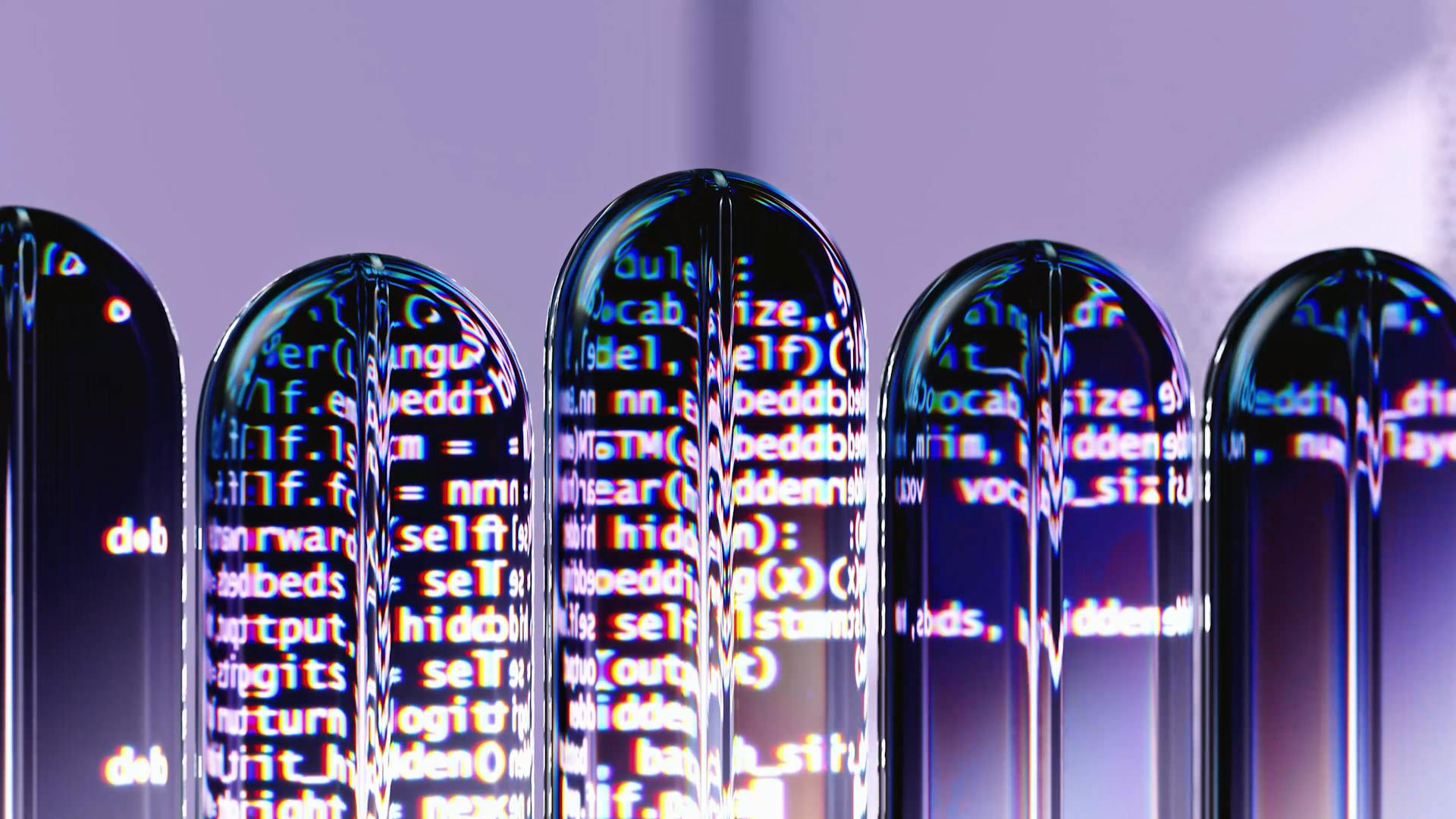
Token subsidies are a crucial aspect of the AI ecosystem, and they're not as well-understood as you'd think.
Tokens are the building blocks of AI responses, and they're broken up from your query. You're charged a fraction of a cent for each token in both the request and the response.
A significant portion of the hundreds of billions of dollars invested into the gen AI ecosystem goes directly toward keeping these costs down. This is done to proliferate adoption, making AI more accessible to everyone.
For example, ChatGPT generates about $400,000 in revenue every day, but the cost to operate the system requires an additional $700,000 in investment subsidy. This is called "Loss Leader Pricing", a common strategy in the tech industry.
This means that companies like Google, OpenAI, Microsoft, and Elon Musk are essentially subsidizing their AI operations to make them more affordable. But what happens when they decide to start making a profit?
For more insights, see: What Is a Token in Generative Ai
The Consequences of Hype
The hype surrounding generative AI can lead to a lack of critical evaluation, causing companies to receive funding based on promises rather than measurable results and experimental validation. This can result in a waste of resources and a distorted view of the technology's potential.
Companies in the AI-powered drug discovery space are often evaluated based on their big names and funding rounds, rather than their actual progress. However, a list of simple questions can help estimate a company's hype-to-results ratio.
Here are some key questions to ask:
- How many of your internally discovered drugs are in Phase I, Phase II, and Phase III?
- Did any of your pharma partnerships progress into Phase I, II, and III, and how long did it take?
- How many preclinical candidates (PCCs) did you nominate in a single year and in total?
- How long, on average, does it take you to nominate a PCC, and how much does it cost?
- What was the level of novelty of the targets and what is the level of novelty of the molecules?
- How long, on average, does it take you to go into clinical trials with an AI-discovered target?
- Did you in-license any of your drugs from other companies, repurpose them, or discover them internally?
- How many pharma companies use your AI software to discover drugs, and how many users do you have? How many renew every year?
- What was your revenue last year, and what was the revenue growth year over year?
- How much money have you raised, in how many rounds, and what is the valuation of your last round?
The media often conflates generative AI with a narrower application of LLM-powered chatbots, which might indeed not be equipped to solve every problem that enterprises face. This can lead to a distorted view of the technology's potential.
The hype surrounding generative AI can also lead to a lack of focus on the actual problems that need to be solved. Chris Hay, an IBM distinguished engineer, argues that AI needs to be part of a broader strategy to solve business problems.
Explore further: Can Generative Ai Solve Computer Science
Beyond the Hype Cycle
To navigate the hype cycle, it's essential to separate fact from fiction. IBM distinguished engineer Chris Hay believes we're in the corrective phase, where the initial enthusiasm is being tempered with a more measured approach.
Journalists, analysts, and investors can ask specific questions to evaluate AI-powered companies. For example, how many internally discovered drugs are in Phase I, Phase II, and Phase III? Did any pharma partnerships progress into Phase I, II, and III, and how long did it take? Answering these questions can help assess the hype-to-results ratio.
Here are some simple questions to ask AI-powered companies:
- How many of your internally discovered drugs are in Phase I, Phase II, and Phase III?
- Did any of your pharma partnerships progress into Phase I, II, and III, and how long did it take?
- How many preclinical candidates (PCCs) did you nominate in a single year and in total?
- How long, on average, does it take you to nominate a PCC, and how much does it cost?
- What was the level of novelty of the targets and what is the level of novelty of the molecules?
- How long, on average, does it take you to go into clinical trials with an AI-discovered target?
- Did you in-license any of your drugs from other companies, repurpose them, or discover them internally?
- How many pharma companies use your AI software to discover drugs, and how many users do you have? How many renew every year?
- What was your revenue last year, and what was the revenue growth year over year?
- How much money have you raised, in how many rounds, and what is the valuation of your last round?
By asking these questions, you can get a clearer understanding of a company's progress and achievements, rather than just relying on promises and big names.
AI-Powered Drug Discovery Benchmarks
In 2020, Insilico Medicine released the AI software suite, Pharma.AI, which is now comprised of Biology42, Chemistry42, and Medicine42.
Ten of the top 20 pharma companies use elements of this software, generating millions in recurrent software revenue.
You might enjoy: Generative Ai for Software Development
It takes Insilico Medicine 11-12 months to reach the PCC stage on average.
The company has nominated 18 PCCs from January 2021 to May 2024, with seven proceeding to human clinical trials and two progressing into Phase II.
Two programs, QPCTL and USP1, have been partnered or out-licensed at the Phase I and PCC stages, respectively, with an upfront payment of $80 million.
If this caught your attention, see: What Are the Two Main Types of Generative Ai Models
Beyond the Hype
The hype cycle is a real phenomenon, and it's easy to get caught up in the excitement. IBM distinguished engineer Chris Hay notes that generative AI is having a marked, measurable impact, but we're in the corrective phase now. This means we need to take a step back and look at the facts.
Most analysts and investors rarely ask the right questions to evaluate AI-powered drug discovery companies. A list of simple questions can help estimate their hype-to-results ratios. These questions include asking about the number of internally discovered drugs in Phase I, Phase II, and Phase III, as well as the level of novelty of the targets and molecules.
Expand your knowledge: Generative Ai Questions
The hype cycle can also lead to extremes in our expectations. Smolinksi argues that AI needs to be part of a broader strategy to solve business problems. It's usually not AI by itself that solves a problem, but rather multiple types of AI models applied in tandem.
To overcome limitations and work within them, engineers will get creative. For example, synthetic data can be created algorithmically to mimic real-world data, serving as an alternative or supplement to it. This can help address the data crisis in the short term.
Hay believes that today's LLMs are power-hungry, but current transformers are not the final architecture. Hybrid architectures that use multiple types of models are gaining traction, and engineers are finding value in other methods, such as quantization and fine-tuning.
Here are some ways to evaluate AI-powered drug discovery companies:
- How many internally discovered drugs are in Phase I, Phase II, and Phase III?
- Did any pharma partnerships progress into Phase I, II, and III, and how long did it take?
- How many preclinical candidates (PCCs) were nominated in a single year and in total?
- How long does it take to nominate a PCC, and how much does it cost?
- What is the level of novelty of the targets and molecules?
- How long does it take to go into clinical trials with an AI-discovered target?
- Were any drugs in-licensed from other companies, repurposed, or discovered internally?
- How many pharma companies use your AI software to discover drugs, and how many users do you have?
- What was your revenue last year, and what was the revenue growth year over year?
- How much money have you raised, in how many rounds, and what is the valuation of your last round?
Agentic Workflows
Agentic workflows are a multi-step approach to using LLMs and AI agents to perform tasks, where these agents act with a degree of independence and decision-making capability.
Take a look at this: Generative Ai Agents
These agents can be designed to handle specific tasks or areas of expertise, bringing in deep knowledge and experience that LLMs might lack. They can either draw on more specialized data or integrate domain-specific algorithms and models.
In an agentic workflow, an LLM processes an inquiry, categorizes the issue, and triggers specific agents to handle various tasks. For instance, one agent retrieves the customer's account details and verifies the information provided.
Agents can operate autonomously and proactively, in real time, by using simpler decision-making algorithms. They can also be designed to learn from and adapt to their environment.
Agents use reinforcement learning or feedback loops to improve performance over time, adjusting strategies based on the success or failure of previous tasks. This is in contrast to LLMs, which can struggle with tasks that require deep domain expertise.
Agentic workflows themselves generate new data, which can then be used for further training. This creates a continuous cycle of improvement and refinement.
You might enjoy: Large Language Model vs Generative Ai
Sources
- https://www.genengnews.com/topics/artificial-intelligence/is-generative-ai-in-drug-discovery-overhyped/
- https://www.ibm.com/blog/with-generative-ai-dont-believe-the-hype-or-the-anti-hype/
- https://www.forbes.com/sites/christianstadler/2024/09/06/the-generative-ai-hype-is-almost-over-whats-next/
- https://sloanreview.mit.edu/article/dont-get-distracted-by-the-hype-around-generative-ai/
- https://venturebeat.com/ai/why-we-need-to-check-the-gen-ai-hype-and-get-back-to-reality/
Featured Images: pexels.com