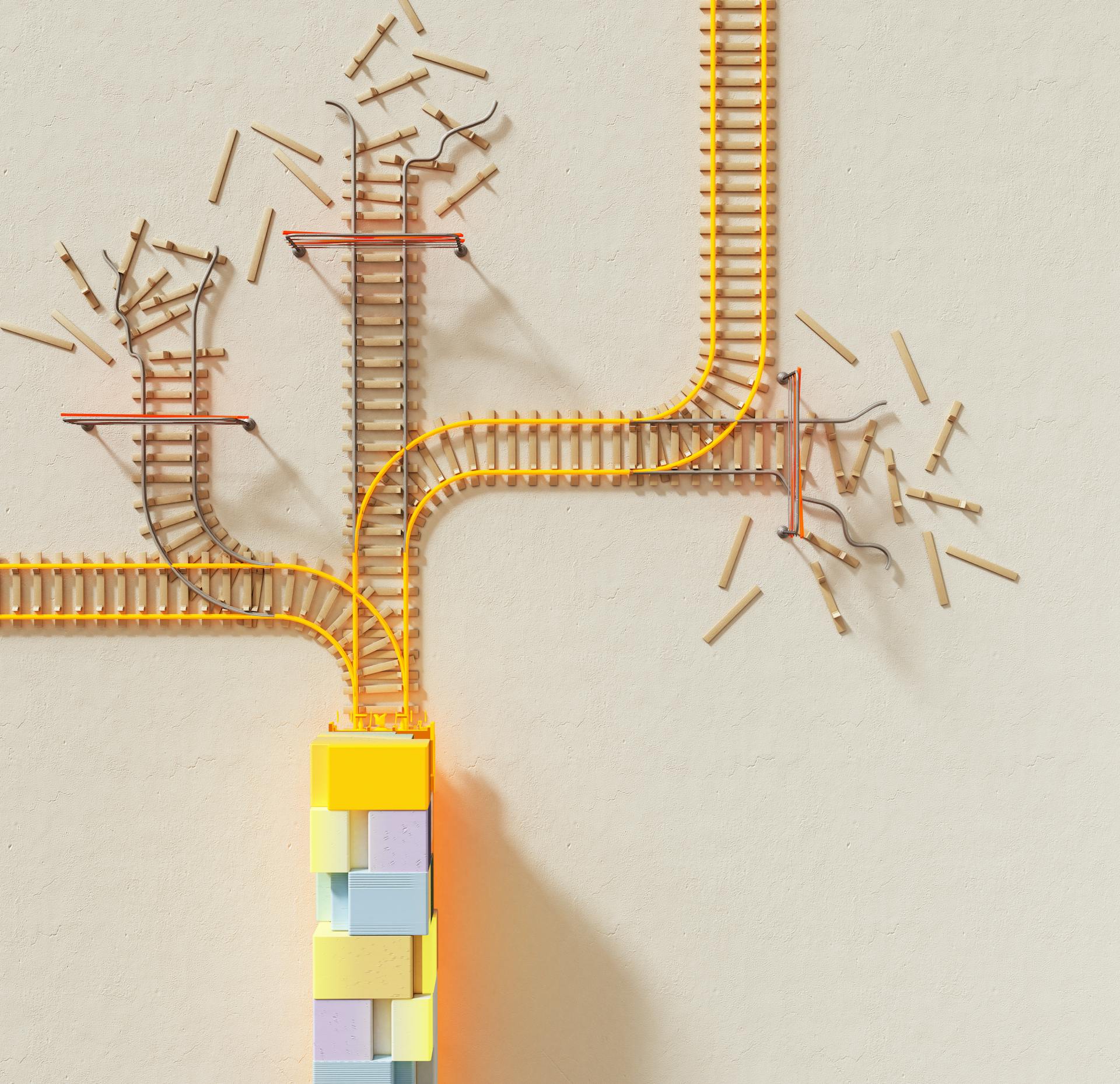
Machine learning is revolutionizing the field of computer security by providing a comprehensive approach to detecting and preventing cyber threats.
The use of machine learning algorithms can analyze vast amounts of data to identify patterns and anomalies that may indicate a security breach.
This approach is particularly effective in detecting zero-day attacks, which are attacks that use previously unknown vulnerabilities.
As a result, many organizations are turning to machine learning to augment their traditional security measures.
Machine learning can also help identify and prioritize potential security threats, allowing security teams to focus on the most critical issues first.
See what others are reading: Machine Learning Facial Recognition Security and Surveillance Systems
What Is Machine Learning in Security?
Machine learning (ML) is a subset of AI that empowers computers to execute tasks without explicit programming. It's built upon algorithms and statistical models designed to recognize patterns and relationships in data, iteratively improving performance.
ML encompasses various techniques, including supervised learning, unsupervised learning, and reinforcement learning. Supervised learning trains models on labeled datasets, while unsupervised learning infers patterns from unlabeled data. Reinforcement learning enables agents to make sequential decisions by interacting with the environment and receiving feedback.
Readers also liked: Reinforcement Learning
A key aspect of ML is its ability to generalize from training data, facilitating accurate predictions on new or unseen data. This is achieved through an iterative learning process, where models are trained with labeled data to adjust internal parameters and minimize the difference between predicted and actual outputs.
Large language models (LLMs) are a specific type of ML that has led the AI industry due to its capacity to understand and generate human-like text. LLMs, such as OpenAI's GPT series, are trained on vast amounts of text data and use deep learning architectures, typically based on transformer models.
Here are some examples of ML techniques used in cybersecurity:
- Supervised learning for tasks like malware classification, botnet detection, and intrusion detection.
- Unsupervised learning for detecting patterns and anomalies in network traffic.
- Reinforcement learning for training models to make sequential decisions in cybersecurity scenarios.
Whether you use custom-trained ML models or integrate with third-party LLM providers, ML can open up many cybersecurity risks and create weaknesses in the overall security architecture.
Improving Security with Machine Learning
Machine learning can significantly improve security by analyzing vast amounts of data and identifying patterns that may indicate potential threats. This approach can be applied in various ways, such as using predictive analytics to identify potential security threats and prioritize them based on impact and occurrence.
Check this out: Ai and Ml in Cyber Security
Organizations need overall visibility of possible threats to stay ahead of potential security breaches. Machine learning algorithms can assist with threat identification and priority threat evaluation at a high level of sophistication. By doing so, organizations can prevent costly security breaches and protect sensitive information.
One effective approach to tackle SQL injection-based breaches involves implementing an enterprise security framework with specific scenarios that trap, stop, and eliminate infected code. SQL injection is a popular technique attackers use to enter organizations' networks and release sensitive information.
Machine learning can also prevent compromised password attacks by utilizing risk-based models that validate user identity based on behavioral pattern analysis. This approach helps detect patterns in how people log in to systems and machines containing sensitive information, preventing attackers from impersonating actual users using stolen passwords.
Risk scoring models are beneficial in planning and accomplishing growth strategies rapidly in an organization spanning multiple geographic regions. These models can also scale security frameworks quickly, assisting with threat assessments across numerous locations.
Here are some ways machine learning can improve security:
- Using predictive analytics to identify potential security threats
- Preventing SQL injection-based breaches
- Eliminating compromised password attacks
- Using risk scoring models to maintain enterprise security settings
Machine learning has the potential to revolutionize cybersecurity by analyzing vast amounts of data, identifying patterns, and making accurate predictions. Its ability to learn and adapt makes it an essential tool for organizations looking to stay ahead of potential security threats.
Getting Started
Machine learning can be a powerful tool for improving your intrusion detection system. Applying machine learning to improve your intrusion detection system is a great place to start.
You don't need to be a machine learning expert to get started. Practical machine learning in infosec is a great resource to learn from.
First, you'll need to choose a project to focus on. Some popular options include detecting malicious URLs and web attacks. Detecting web attacks with recurrent neural networks is a great example of how machine learning can be applied to real-world problems.
Here are some popular machine learning projects in computer security:
- Machine Learning based Password Strength Classification
- Using Machine Learning to Classify Packet Captures
- Using Machine Learning to Detect Malicious URLs
- Detecting Web Attacks With Recurrent Neural Networks
- Machine Learning for Detecting Phishing Websites
- Password Hunting with ML in Active Directory
Once you've chosen a project, you can start exploring different machine learning algorithms and techniques. Analyzing botnets with Suricata and machine learning is a great example of how machine learning can be used to detect and analyze complex threats.
Cybersecurity Course Format
The format of a cybersecurity course can vary, but many incorporate hands-on labs and projects to give students practical experience in machine learning for security.
Hands-on labs are essential for learning machine learning concepts in a cybersecurity context.
Some courses use a combination of online and in-person instruction, while others are entirely online.
Online courses often include pre-recorded video lectures and interactive quizzes to reinforce learning.
In-person courses, on the other hand, provide students with the opportunity to ask questions and engage with instructors and peers.
Many courses also include a final project or capstone that requires students to apply their machine learning skills to a real-world security problem.
This project-based approach helps students develop problem-solving skills and think critically about security challenges.
By the end of the course, students should be able to design and implement their own machine learning models to detect and prevent cyber threats.
Take a look at this: Learning with Errors Problem
Sources
- https://www.kaspersky.com/enterprise-security/wiki-section/products/machine-learning-in-cybersecurity
- https://securityintelligence.com/posts/machine-learning-applications-in-the-cybersecurity-space/
- https://github.com/jivoi/awesome-ml-for-cybersecurity
- https://www.kroll.com/en/insights/publications/cyber/machine-learning-cybersecurity
- https://professional.uchicago.edu/lp/professional-education/machine-learning-cybersecurity/rfi
Featured Images: pexels.com