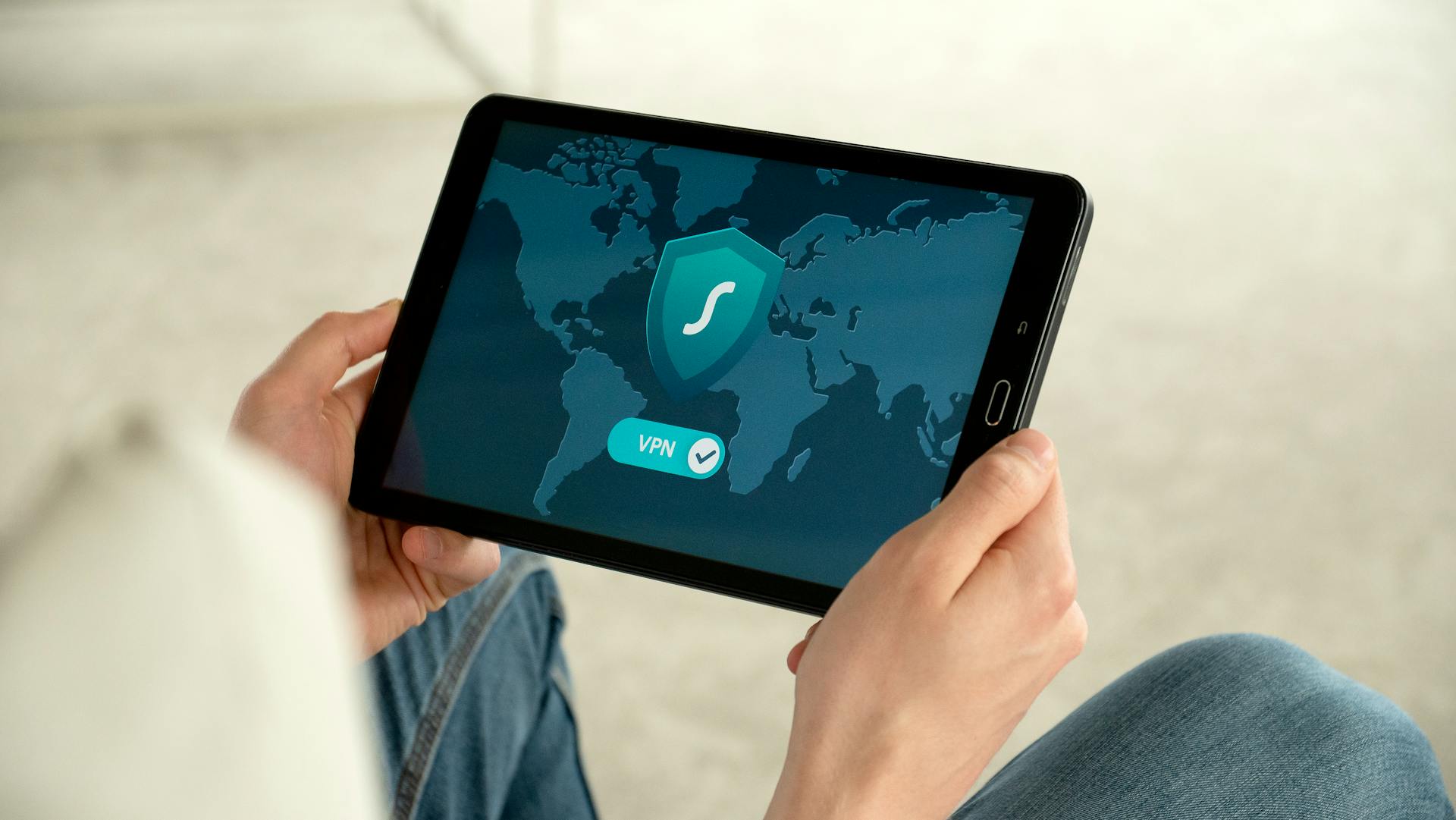
As a privacy expert, navigating the complexities of AI and ML can be daunting. Machine learning algorithms can collect and analyze vast amounts of personal data, often without users' knowledge or consent.
The EU's General Data Protection Regulation (GDPR) requires organizations to obtain explicit consent from users before collecting and processing their personal data, including biometric data used in facial recognition technology.
AI systems can be trained on biased data, leading to discriminatory outcomes. For example, a study found that a facial recognition system trained on a dataset of mostly white faces struggled to recognize darker-skinned individuals.
To mitigate these risks, experts recommend implementing transparency and accountability measures, such as data audits and algorithmic impact assessments.
Additional reading: Ai Personal Computer
The Impact of AI and ML
Machine learning (ML) plays a crucial role in AI, and it's essential to understand its impact on consumer privacy. AI and data privacy are intrinsically connected because of ML.
ML uses vast quantities of data to learn and develop its own logic. This data, or big data, is characterized by the three Vs: volume, variety, and velocity.
The more data you feed a model, the more it develops its own logic. This learning can be used in the form of generative AI or automation.
Big data has a considerable impact on ML, and it's used to "teach" models using supervised or unsupervised learning.
See what others are reading: How to Create Ai Software
Privacy Concerns
Lack of transparency and control can lead to significant privacy issues, as individuals may not know what information is being collected and why, making it difficult to give informed consent.
Without transparency, people can't understand how their data is being used, which raises concerns about data protection and accountability.
Complex AI systems can be opaque, making it hard to identify biases or errors in the AI's decisions.
Clear communication is essential for building trust with users and stakeholders, and implementing accountability measures demonstrates responsible data handling.
Informing stakeholders about data usage, purpose of collection, and data protection measures can help establish trust and ensure responsible data handling.
Data Collection and Security
Data collection is a crucial aspect of AI and ML, but it's essential to collect data ethically and legally with clear consent from individuals. This is a significant challenge.
Data breaches can expose sensitive personal information, leading to privacy violations and potential harm to individuals. Designing data security to keep information safe is of utmost importance.
To protect data, conduct regular security audits using AI threat intelligence to identify vulnerabilities in AI systems and data storage infrastructures. Ensure compliance with relevant data protection regulations such as GDPR, CCPA, or any industry-specific standards.
Collect only the data that is absolutely necessary for a specific AI application, following the data minimization principle to reduce the volume of sensitive information at risk and aligns with privacy-by-design principles.
Surveillance
Surveillance is a critical aspect of data collection and security. The use of AI in surveillance systems raises significant privacy concerns, such as the potential for facial recognition technology to monitor individuals without their consent in public spaces or through their social media usage.
Clearview AI, a company that provides facial recognition technology, has extended its database access to US public defenders, raising concerns about the potential misuse of personal data. This can lead to individuals losing their anonymity and privacy, being followed wherever they go.
Facial recognition technology is being used in various settings, including law enforcement, where one in two US adults is reportedly in a facial recognition database. The use of facial recognition in public spaces, such as subway systems, also raises data security and privacy concerns.
The ACLU has filed a class-action lawsuit against Clearview AI under biometric privacy law, highlighting the need for regulation and oversight in the use of facial recognition technology. The company's practices have also been criticized for lacking transparency and accountability.
In addition to facial recognition, surveillance systems can also use machine learning to analyze data and identify patterns. However, this can also lead to the collection of sensitive personal data without consent.
Collection
Collecting data is a crucial step in developing AI models, but it's essential to do so ethically and with clear consent from individuals. This means ensuring that data is collected with explicit consent, which is a significant challenge.
Data can be collected from various sources, including IoT devices, which generate vast amounts of customer data. Using federated learning for your AI model can be a great alternative to collecting this data, reducing the risk of a database breach.
Federated learning trains AI systems using decentralized devices or servers without sharing local data, thus reducing privacy and security risks. This approach allows AI systems to learn from data without needing to centralize sensitive information.
Homomorphic encryption is a technique that enables the computation of encrypted information without having to decrypt it first. It helps protect information from hackers who may try to access it during the processing stage.
Before using personal data for training AI models, it's essential to anonymize or pseudonymize it to remove or replace identifiers that link the data to an individual. Anonymizing data can help protect privacy and reduce risks if the data is compromised.
You might like: Generative Ai Risks
Security
Data security is a top concern when it comes to AI systems, as they can be vulnerable to cybersecurity threats.
Regular security audits are essential to identify vulnerabilities in AI systems and data storage infrastructures, using AI threat intelligence to stay ahead of cyber threats.
Designing data security to keep information safe is of utmost importance, as data breaches can expose sensitive personal information, leading to privacy violations and potential harm to individuals.
Conducting regular security audits and ensuring compliance with relevant data protection regulations, such as GDPR and CCPA, is crucial to protect the data you've stored.
To prevent unauthorized access to sensitive data, implement strict access controls and authentication mechanisms, including multi-factor authentication, role-based access controls, and logging and monitoring access to sensitive data.
Collect only the data that is absolutely necessary for a specific AI application, following the data minimization principle to reduce the volume of sensitive information at risk.
Using strong encryption methods for data at rest and in transit can ensure that even if it's intercepted or accessed without authorization, it remains unreadable and secure from misuse.
Homomorphic encryption is a technique that enables the computation of encrypted information without having to decrypt it first, helping to protect information from hackers who may try to access it during the processing stage.
Techniques such as federated learning and homomorphic encryption allow AI models to learn from data without ever compromising it, significantly reducing the risk of privacy breaches.
Quantum encryption, or quantum key distribution (QKD), uses the principles of quantum mechanics to secure communication channels, making it virtually impossible for intruders to intercept or decipher data without detection.
Here's an interesting read: What Is Quantum Ai Software
Infrastructure Requirements
To implement AI and ML, you'll need substantial computational resources and data storage capabilities, which can be a challenge for smaller organizations.
Building a scalable, robust, and secure IT infrastructure is critical for AI and ML implementation.
Leveraging Cloud Computing solutions, like Infrastructure as a Service (IaaS) or Platform as a Service (PaaS), can provide flexible and scalable solutions that meet these needs.
If this caught your attention, see: Ai & Ml Solutions
Mitigating Issues
Data collection is an integral part of AI and ML, but it comes with certain risks. To maintain privacy, we need to secure the data and make sure it can't be linked to the individual it came from.
Excess information is not an asset; it takes up storage space and has to be protected. In traditional forms of machine learning, information was collected and stored in a single database, making it easier to steal.
To mitigate issues, we need to balance AI's data needs with the need to minimize data collection and retention. This can be challenging, but it's essential to protect individual privacy.
Here are the key principles to follow:
- Limitation of collection: Only collect what's needed, and nothing more.
- Specification of purpose: Clarity on what the information will be used for.
- Limitation of use: Using the information only for its intended purpose.
By following these principles, we can minimize the risks associated with data collection and ensure that AI and ML are used responsibly.
Responsible AI and ML
Responsible AI and ML is a crucial aspect of ensuring that AI and machine learning systems are used in a way that respects individuals' rights and freedoms.
Big data brings big responsibility, and robust data protection and privacy laws are essential to ensure that personal information handling practices are covered by stringent regulations.
The General Data Protection Regulation (GDPR) in Europe and the Federal Trade Commission (FTC) in the US are examples of regulations that protect personal data from the risks of AI technologies.
Bias and discrimination are significant concerns in AI, as systems can inadvertently learn and perpetuate biases present in the training data.
Developing robust data governance policies is essential to protect customers' information, and these policies should include guidelines for ethical data use, privacy impact assessments, and procedures for responding to data breaches.
Lack of transparency and control over personal data is a significant issue, as individuals may not know what information is being collected and why, making it difficult for them to give informed consent.
Clear communication is essential for building trust with users and stakeholders, and implementing clear accountability measures can ensure responsible data handling.
Explainability and trust are significant challenges in AI, as complex systems can act as 'black boxes' where the decision-making process is not entirely transparent.
Ensuring transparency in AI operations and data usage is critical, and developing techniques for 'Explainable AI' is a significant challenge.
Advanced Techniques
Differential privacy is a technique that adds randomness to data queries, ensuring individual privacy while allowing for useful analysis. It's a way to measure how much privacy an algorithm provides, using a mathematical guarantee to protect the privacy of individual data.
The National Institute of Standards and Technology (NIST) provides crucial guidance on implementing differential privacy. New algorithms and techniques are being developed to provide stronger guarantees of privacy.
These innovations are making differential privacy more effective and practical for real-world applications.
Inference
Inference is a powerful technique used by AI systems, but it also raises significant privacy concerns. AI systems can infer sensitive information about individuals that wasn’t explicitly provided, like health status or sexual orientation.
This is done by using seemingly unrelated data to make predictions about personal attributes. For example, an AI might use data about your online browsing habits to predict your sexual orientation.
AI systems can infer sensitive information even when the original data collection was considered non-sensitive. This is because the AI system can find patterns and connections in the data that weren't apparent to humans.
This raises serious concerns about data privacy and how our personal information is being used. We need to be aware of how AI systems are using our data and what they're inferring about us.
Generative
Generative AI is a rapidly evolving field with significant implications for intellectual property, privacy, and governance.
Generative AI systems raise complex copyright implications, particularly regarding AI inputs and outputs.
The rise of generative AI has led to new privacy risks, including the potential for unauthorized use of personal data.
Effective AI governance is crucial to unlock innovation in generative AI, but can be challenging to master.
The GDPR poses significant challenges for generative AI, with many questions remaining unanswered.
Encryption alone is not enough to protect conversational AI, which requires more robust measures to ensure security.
Automated decision-making and automated decision-execution are distinct concepts that require different approaches.
AI assessments are essential to identify and mitigate risks, but should be conducted judiciously and at the right time.
Generative AI's emergent abilities can be a double-edged sword, bringing both benefits and risks.
An ethical approach to AI does not require reinventing the wheel, but rather building on existing frameworks and guidelines.
Managing privacy and AI risks requires careful consideration and coordination within the same project.
Related reading: Ai Implications
Sources
- https://bigid.com/blog/navigating-ai-privacy/
- https://councils.forbes.com/blog/ai-and-machine-learning
- https://iapp.org/resources/topics/artificial-intelligence-1/
- https://www.dataprivacyadvisory.com/compliance-resources/mastering-ai-a-guide-for-data-protection-practitioners/
- https://fpf.org/blog/fpf-release-the-privacy-experts-guide-to-ai-and-machine-learning/
Featured Images: pexels.com