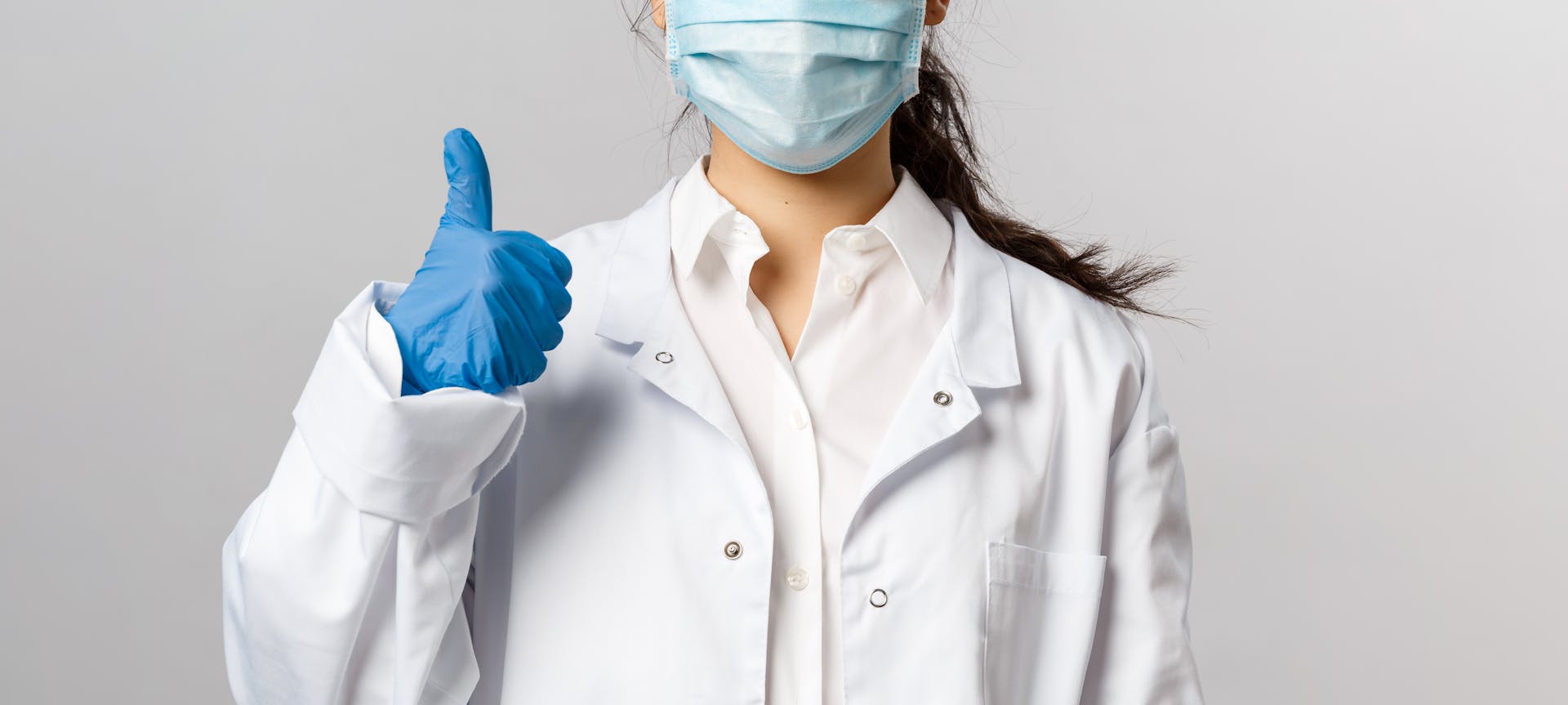
The integration of AI in healthcare has brought about numerous benefits, such as improved diagnosis accuracy and enhanced patient outcomes. This is particularly evident in the use of AI-powered diagnostic tools, which have been shown to detect diseases more accurately than human clinicians in some cases.
However, with the increased use of AI in healthcare comes a multitude of challenges, including the risk of bias in AI decision-making. For instance, AI algorithms can perpetuate existing biases if they are trained on biased data, leading to discriminatory outcomes.
The use of AI in healthcare also raises concerns about patient autonomy and informed consent. As AI systems become more autonomous, there is a growing need for clear guidelines on how to obtain informed consent from patients.
The development of AI in healthcare requires a multidisciplinary approach, involving clinicians, ethicists, and engineers working together to address these challenges and ensure that AI systems are developed with the needs of patients in mind.
Broaden your view: Ai Ml in Healthcare
Bias
Bias is a significant concern in AI healthcare, as AI algorithms can reproduce bias present in their training data.
AI hallucinations occur when generative AI models provide fabricated data outputs that appear authentic, often due to biased or inaccurate data points.
This can lead to poorer results for patients of color, who may be misdiagnosed or left undiagnosed when diagnostic AIs are made on data from white people.
To mitigate bias, teams must prioritize diversity and inclusion in clinical trials to collect various perspectives for the AI model to analyze.
Human oversight is crucial, as experts must critically analyze and cross-check data outputs against expert publications to ensure accuracy and fairness.
Ongoing audits can also detect and monitor bias vulnerabilities in the AI model's design, helping to prevent these issues from arising in the first place.
Here's an interesting read: Ai Training Datasets
Transparency and Accountability
Transparency and accountability are crucial aspects of AI in healthcare. AI systems often operate in a "black box", where their decision-making processes are not easily understood. This lack of transparency can make it difficult to determine who is responsible when errors occur.
In critical domains like healthcare, transparency is vital to understand how decisions are made and who bears responsibility for them. Clarifying accountability is particularly important when AI systems make errors or cause harm. This ensures that appropriate corrective actions can be taken.
Researchers are working to develop explainable AI, which helps characterize the model's fairness, accuracy, and potential bias. This approach can help combat the challenges of black box AI. Explainability is a key strategy to uphold data security and mitigate any bias in AI models.
Establishing accountability in AI-powered healthcare is not straightforward. It's often unclear whether errors occur due to the system designers, healthcare practitioners, or the AI system itself. This ambiguity can lead to confusion and difficulties in assigning responsibility.
To promote transparency and accountability, clinical and research teams can prioritize explainability when using AI models. This can be achieved by using simpler, interpretable AI models to explain the outputs of more complex systems. By doing so, healthcare providers and patients can understand the decision-making processes behind AI-driven diagnoses and treatments.
For your interest: Generative Ai in Healthcare Examples
AI Ethics
The use of AI in healthcare raises important questions about ethics. The Four core ethical concepts that apply to the relationship between artificial intelligence and healthcare are beneficence, non-maleficence, patient autonomy, and distributive justice.
Beneficence requires that AI does good to the patient, while non-maleficence means that AI should not be allowed to cause any harm. Patient autonomy values the rights of patients and their freedom to make decisions about their own care, while distributive justice ensures that every patient receives fair treatment.
Transparency is crucial in AI algorithms, which is why explainability is essential. This means training AI algorithms to be interpretable by healthcare providers and patients, and presenting their decision-making processes in a comprehensible manner.
The creation of ethical frameworks and guidelines is a crucial task in regulating the use of AI in healthcare. Such frameworks should be developed with the participation of various stakeholders, including patients, practitioners, developers of AI systems, and officials.
The pillars of ethical systems in AI healthcare include legal frameworks and regulation, self-regulation, and the establishment of regulatory authorities to oversee the use of AI. Regular checks and revisions of ethical policies are also necessary to keep up with advancements in technology.
If this caught your attention, see: Generative Ai Healthcare Use Cases
Data Protection and Privacy
Data protection and privacy are significant concerns in the use of AI in healthcare. Patient confidentiality and data protection are still issues, and any unauthorized attempt to gain access or cases where people's sensitive health information gets violated can prejudice individuals in severe ways.
Data storage and sharing are key areas of concern. Data storage refers to the ways and places in which patient data is managed, as well as the persons who can access it. Data sharing involves minimizing data leakage to unauthorized subjects and using it only for the designated purpose.
The General Data Protection Regulation (GDPR) was enacted by the European Union to protect personal data and activities of foreign communities and companies. This regulation requires data processors and controllers to protect the information of natural persons with sufficient protection.
In the United States, the Genetic Information Non-discrimination Acts (GINA) prohibits employers from making discriminatory decisions based on genetic health information. However, current laws are not enough to protect an individual's health data, and clinical data collected by robots can be hacked into and used for malicious purposes.
Some social networks gather and store large amounts of users' data, including mental health data, without their consent. This data can be used for marketing, advertising, and sales purposes. Additionally, some genetics testing and bioinformatics companies sell customer data to pharmaceutical and biotechnology companies without proper consent or monitoring.
To ensure data protection and privacy, patients must be informed about the use of AI in their healthcare and consent to its use. This includes understanding how AI will be used, its benefits, risks, and any potential implications for their care.
Here are some key data protection and privacy concerns in AI healthcare:
- Data Storage: The ways and places in which patient data is managed, as well as the persons who can access it.
- Data Sharing: Minimizing data leakage to unauthorized subjects and using it only for the designated purpose.
- Unauthorized access: Clinical data collected by robots can be hacked into and used for malicious purposes.
- Lack of consent: Some social networks gather and store large amounts of users' data, including mental health data, without their consent.
AI in Healthcare
AI in healthcare is a complex and rapidly evolving field. Transparency in AI algorithms is crucial for effective implementation in healthcare.
Explainability is key, as AI algorithms need to be trained to be interpretable by healthcare providers and patients. This means presenting their decision-making processes in a comprehensible manner.
Responsibility is also a significant concern, as there is currently an undefined framework on how to blame AI occurrences or mistakes.
This lack of accountability can lead to mistrust in AI-driven healthcare systems.
Sources
- five-year workplan (europa.eu)
- exploring benefits (fda.gov)
- cybersecurity measures (hitrustalliance.net)
- “AI hallucinations” (mit.edu)
- Ethical Considerations in AI-Assisted Healthcare (mednoting.com)
- Ethical concerns mount as AI takes bigger decision-making ... (harvard.edu)
- Several U.S. agencies recently issued warnings (cnbc.com)
- researchers are working to better develop explainable AI (towardsdatascience.com)
- https://www.theguardian.com/global-development/2020/dec/02/robodoc-how-india-robots-are-taking-on-covid-patient-care-mitra (theguardian.com)
- DOI (doi.org)
- Google Scholar (google.com)
- DOI (doi.org)
- https://www.ama-assn.org/delivering-care/ethics/informed-consent (ama-assn.org)
- Google Scholar (google.com)
- Google Scholar (google.com)
- https://www.reuters.com/article/us-health-coronavirus-italy-robots-idUSKBN21J67Y (reuters.com)
- Google Scholar (google.com)
- Google Scholar (google.com)
- Google Scholar (google.com)
- DOI (doi.org)
- DOI (doi.org)
- Google Scholar (google.com)
- DOI (doi.org)
Featured Images: pexels.com