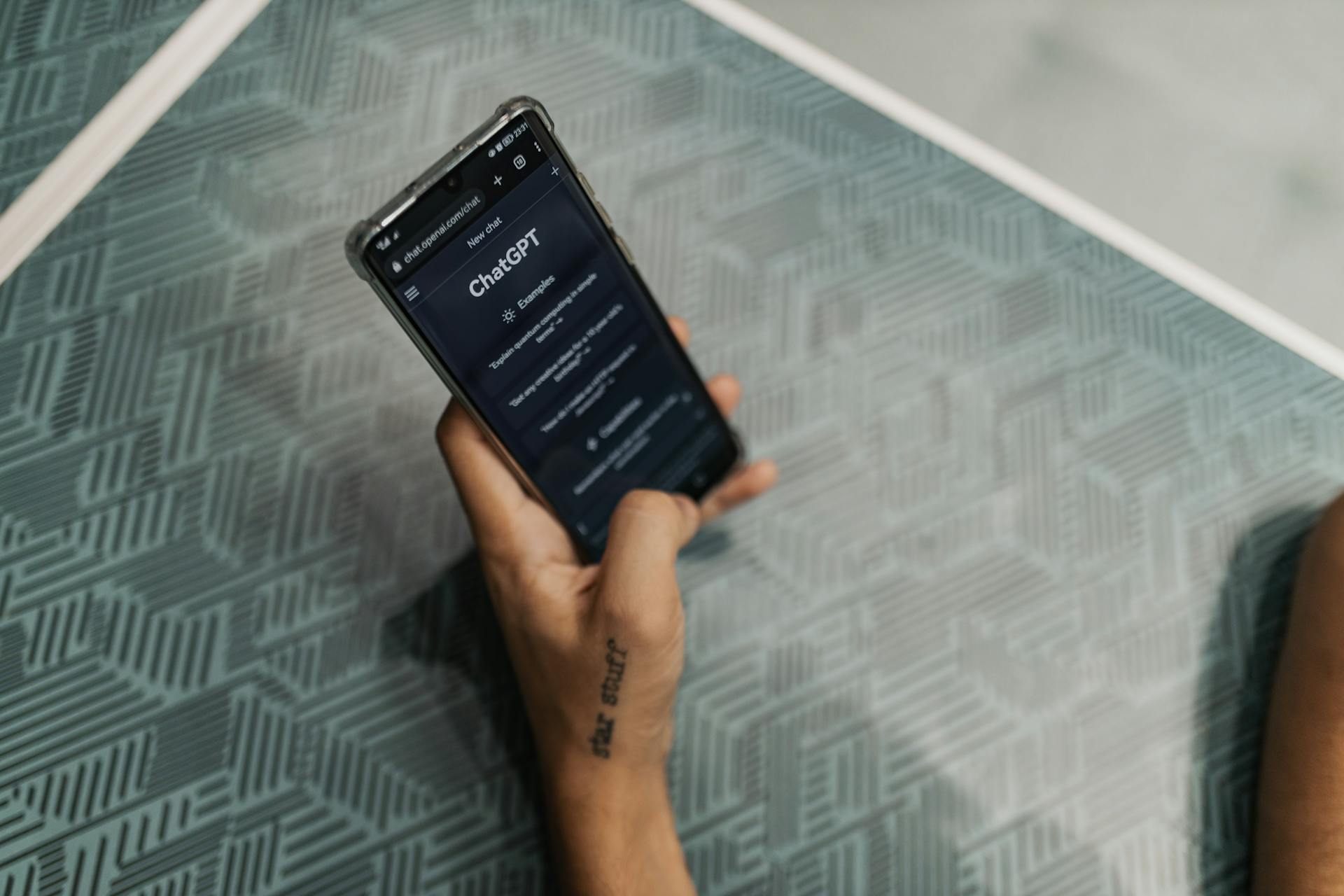
Generative AI chips are revolutionizing the tech industry with their ability to unlock efficiency and innovation. They're designed to handle complex tasks with ease, freeing up computing resources for more important tasks.
These chips are specifically engineered to optimize generative AI models, allowing them to process large amounts of data quickly and accurately. As a result, they're being used in a wide range of applications, from image and video generation to natural language processing.
One of the key benefits of generative AI chips is their ability to reduce the computational power required to run AI models. This is especially important for applications that require real-time processing, such as video games and virtual reality experiences.
Readers also liked: Foundations and Applications of Generative Ai
Generative AI Chip Components
Servers will employ high-performance GPUs or specialized AI chips, such as ASICs, to efficiently handle gen AI workloads through parallel processing.
Training workloads are expected to be executed using a CPU+GPU combination, with two CPUs and eight GPUs for compute, which is the prevailing high-performance gen AI server architecture.
A transition to system-in-a-package design for GPUs and AI accelerators is also expected, with both architectures expected to coexist.
Inference workloads will favor specialized hardware due to lower cost, higher energy efficiency, and faster or better performance for highly specialized tasks.
In 2030, more inference-specific AI servers are expected to use a combination of CPUs and several purpose-built AI accelerators that use ASICs.
Industry Trends and Players
Generative AI chips are being developed by major tech companies such as Google, Microsoft, and NVIDIA, who are investing heavily in the technology.
These companies are racing to create the first commercial-grade generative AI chips, with Google announcing its first chip, the Tensor Processing Unit (TPU), in 2016.
You might enjoy: Companies Using Generative Ai
Data Center Infrastructure and Hardware Trends
In the next decade, data center infrastructure and hardware trends will undergo significant changes to accommodate the increasing demand for computational power in gen AI workloads.
Semiconductor leaders will need to adapt to changes in underlying hardware and infrastructure, mainly to data center infrastructure, servers, and semiconductor chips.
Broaden your view: Generative Ai Contact Center
Servers will employ high-performance graphics processing units (GPUs) or specialized AI chips, such as application-specific integrated circuits (ASICs), to efficiently handle gen AI workloads through parallel processing.
Today, infrastructure for gen AI training and inference is expected to bifurcate as inference's compute demand becomes more specific to the use case and requires much lower cost to be economical.
Training server architecture is expected to be similar to today's high-performance cluster architectures in which all servers in a data center are connected to high-bandwidth, low-latency connectivity.
In 2030, most training workloads are expected to be executed using a CPU+GPU combination, with two central processing units (CPUs) and eight GPUs for compute.
A transition to system-in-a-package design for GPUs and AI accelerators is also expected, with both architectures expected to coexist.
Inference workloads will shift to mostly inference, favoring specialized hardware due to lower cost, higher energy efficiency, and faster or better performance for highly specialized tasks.
By 2030, more inference-specific AI servers are expected to use a combination of CPUs and several purpose-built AI accelerators that use ASICs.
Broaden your view: Ai Training Devices
NVIDIA
NVIDIA is currently the leading provider of AI chips, having previously made a name for itself with GPUs.
The company developed dedicated AI chips like the Tensor Core GPUs and the NVIDIA A100, considered the most powerful AI chip in the world at the time of this writing.
The A100 features Tensor Cores optimized for deep learning matrix arithmetic and has a large, high-bandwidth memory.
NVIDIA's AI chips also support CUDA, a parallel computing platform and API model, making them versatile for various AI and machine learning applications.
B2B Use Case Archetypes
B2B use cases for gen AI applications can be broadly categorized into six archetypes. These archetypes are based on the type of tasks the applications perform, such as coding, content generation, customer engagement, innovation, and concision.
The six B2B use case archetypes are:
- Coding and software development apps that interpret and generate code
- Creative content–generation apps that write documents and communication
- Customer engagement apps that cover automated customer service for outreach, inquiry, and data collection
- Innovation apps that generate product and materials for R&D processes
- Simple concision apps that summarize and extract insights using structured data sets
- Complex concision apps that summarize and extract insights using an unstructured or large data set
Each of these archetypes has its own unique characteristics and requirements. For instance, coding and software development apps require a significant amount of compute power to interpret and generate code.
Technologies and Innovations
Artificial intelligence (AI) chips are transforming the way we process data, making them highly efficient for machine learning workloads.
GPUs were once considered the go-to choice for AI workloads, but they're no longer enough for those on the cutting edge of AI development. Traditional CPUs are also being replaced by more specialized hardware.
Machine learning algorithms require massive amounts of computing power, which is where AI chips come in – they're designed from the ground up to perform AI tasks more efficiently than traditional CPUs or GPUs can do.
Here are some of the key technologies driving the evolution of AI chip technology:
- Graphical Processing Units (GPUs)
- Central Processing Units (CPUs)
- Specialized processors
Logic Chips
Logic chips are essential for artificial intelligence, and their demand is expected to skyrocket by 2030.
By that year, the majority of general AI compute demand will come from inference workloads, which require specific types of servers.
Currently, CPU+GPU servers are the most widely available and used for both inference and training workloads.
However, AI accelerators with ASIC chips are expected to take over in 2030, performing optimally in specific AI tasks.
In 2030, McKinsey estimates that 15 million logic wafers will be needed for non-gen AI applications.
Of these, 7 million will be produced using technology nodes of more than three nanometers, and 8 million will use nodes equal to or less than three nanometers.
Gen AI demand will require an additional 1.2 to 3.6 million wafers using nodes equal to or less than three nanometers.
Unfortunately, there will be a small supply shortage, with only 0.5 million wafers available for gen AI demand.
This shortage is due to the fact that the available free capacity will not be enough to meet the demand for nodes equal to or less than three nanometers.
As a result, a potential supply gap of 1 to 4 million wafers will need to be addressed by 2030.
Suggestion: Generative Ai in the Supply Chain
DDR and HBM
Gen AI servers use two types of DRAM: HBM, attached to the GPU or AI accelerators, and DDR RAM, attached to the CPU. HBM has higher bandwidth but requires more silicon for the same amount of data.
The industry faces a memory wall problem, where memory capacity and bandwidth are the bottleneck for system-level compute performance. This is a major challenge to hardware and software design.
HBM has a higher bandwidth compared to DDR RAM, but its higher silicon requirement limits its adoption. This is especially true when considering the high cost of SRAM, which is being tested in various chips to increase near-compute memory.
AI accelerators are generally lighter in memory compared to CPU+GPU architecture. This could lead to a slower growth in memory demand as more AI applications are deployed.
By 2030, DRAM demand from gen AI applications could be as low as five million wafers or as high as 13 million wafers, depending on the scenario. This translates to a range of four to 12 dedicated fabs.
Broaden your view: Foundations and Applications of Generative Ai Grants
Pursuing Innovation in Semiconductors
Investing in innovation across the semiconductor value chain is crucial to capture the value of generative AI. Large amounts of global investment are needed to reduce costs, optimize compute efficiency, and increase capacities to meet demand.
Examples of innovative technologies include new algorithm designs to reduce computational requirements, such as the invention of different transformer models. These models decrease computational demands and are a new approach to designing algorithms.
New chip architectures can achieve higher performance using the same area of silicon, and several start-ups have already developed such an architecture. Increased memory density of chips can also increase their storage capacity, for example, by using data compression similar to Linux's zram but implemented on the chip.
Improved high-speed networks between servers can provide faster access to the memory of other servers, reducing the need for storing local duplicates of data. Optimized software or compilers can also improve system-level infrastructure compute efficiency.
To spur innovation, it's necessary to combine new requirements with high levels of investment. This includes increasing demand for power semiconductors, as gen AI servers consume higher amounts of energy. Optical components, such as those used in communications, are also expected to transition to optical technologies over time.
Here are some examples of innovative technologies and their potential impact:
- New algorithm designs to reduce computational requirements
- New chip architectures to achieve higher performance using the same area of silicon
- Increased memory density of chips to increase their storage capacity
- Improved high-speed networks between servers
- Optimized software or compilers to improve system-level infrastructure compute efficiency
By investing in innovation and combining new requirements with high levels of investment, we can capture the value of generative AI and drive growth in the semiconductor industry.
Benefits and Challenges
Generative AI chips present a unique combination of benefits and challenges that are worth exploring.
The benefits of generative AI chips are numerous, including their ability to accelerate the development and implementation of AI and data science projects. They can process complex tasks at incredible speeds, making them a game-changer for industries that rely heavily on AI.
One of the main challenges organizations face when adopting generative AI chips is the complexity of their development and implementation. The unique set of challenges involved can be daunting, but with the right approach, the benefits can far outweigh the difficulties.
The development and implementation of generative AI chips require specialized expertise, which can be a major hurdle for some organizations. However, with the right training and resources, businesses can overcome this challenge and unlock the full potential of these powerful chips.
Readers also liked: What Makes Generative Ai Unique
Efficiency
AI chips are a game-changer when it comes to efficiency. They're designed specifically for AI and machine learning workloads, making them significantly more efficient than traditional CPUs.
Traditional CPUs are not designed to handle parallel processing requirements of AI workloads, which can lead to slower processing times and less accurate results. This is why AI chips can perform tasks faster and with greater accuracy.
AI chips can handle larger and more complex workloads at a lower cost, making them a more cost-effective option for businesses and organizations. This is a huge advantage for companies looking to integrate AI technology into their systems.
By using AI chips, businesses can reduce their energy consumption and lower their costs. This is because AI chips are designed to be more energy-efficient than traditional CPUs.
The increased efficiency of AI chips can also lead to significant cost savings for businesses and organizations. This is a major benefit for companies looking to integrate AI technology into their systems.
Expand your knowledge: Why Is Controlling the Output of Generative Ai
Challenges of Adoption
Implementing AI chips within an organization's existing technology infrastructure is a significant challenge. This complexity extends not just to hardware integration but also to software and algorithm development, as AI chips typically require specialized programming models and tools.
For another approach, see: Ai Computer Chips
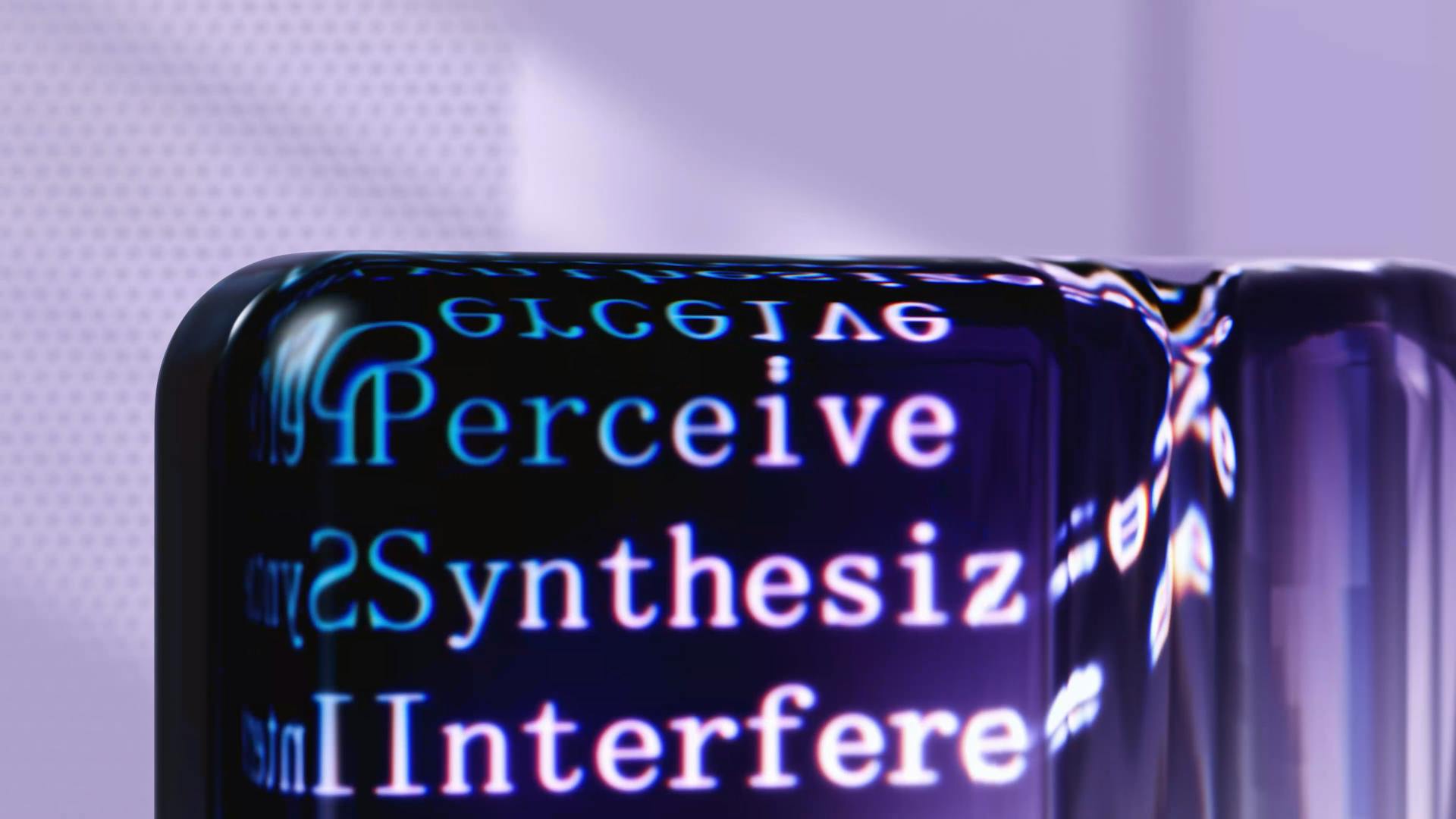
Organizations must either invest in training their existing workforce or recruit new talent with the necessary expertise. The skill set required to effectively implement and optimize AI chip-based systems is still relatively rare.
A redesign or substantial adaptation of existing systems is often necessary to accommodate AI chips. This can be a costly and time-consuming process, especially for smaller organizations or those new to the field of AI.
The need for specialized knowledge can create barriers to entry for organizations that don't have the resources or expertise to implement AI chip-based systems.
For your interest: How Are Modern Generative Ai Systems Improving User Interaction
Obsolescence Risk
The rapid pace of AI technology advancement can be both exciting and intimidating. AI chip technology is constantly evolving, leading to a continuous cycle of innovation and new product development.
Organizations investing in AI chip technology face the challenge of their hardware becoming outdated relatively quickly. This is because newer, more efficient chips are constantly being released.
The risk of obsolescence can lead to hesitancy in investment, particularly for organizations with limited budgets. Managing costs while staying at the forefront of technology is a delicate balance.
Careful strategic planning and consideration of long-term technological trends are essential to mitigate this risk.
Suggestion: Roundhill Generative Ai & Technology Etf
Leading Manufacturers and Customers
Nvidia's top customers include Google, Microsoft, and Amazon, which together make up over 40% of Nvidia's revenue. These tech giants are building their own processors for internal use, posing a challenge to Nvidia's dominance.
Amazon has introduced its own AI-oriented chips, Inferentia, which is now on its second version. Google, on the other hand, has been using Tensor Processing Units (TPUs) since 2015 to train and deploy AI models.
Google's commitment to its own silicon is evident, with the company announcing the sixth version of its chip, Trillium, which is used to develop its models, including Gemini and Imagen.
A unique perspective: Generative Ai Explained Nvidia
AMD
AMD has made a significant move into the AI space with its Radeon Instinct GPUs, which are tailored for machine learning and AI workloads.
These GPUs feature advanced memory technologies and high throughput, making them suitable for both training and inference phases.
AMD provides ROCm, or Radeon Open Compute Platform, which enables easier integration with various AI frameworks.
ROCm allows developers to write code once and run it on multiple architectures, making it a powerful tool for AI development.
The Radeon Instinct GPUs are a testament to AMD's ability to adapt and innovate in the rapidly evolving field of AI.
Suggestion: Amd Ai Training
Nvidia's Top Customers
Nvidia's top customers are some of its biggest competitors in the market. Cloud providers like Google, Microsoft, and Amazon are building their own processors for internal use, which can be a challenge for Nvidia.
Amazon introduced its own AI-oriented chips, Inferentia, in 2018, and has since released a second version. Inferentia chips are now available for rent through Amazon Web Services, which markets them as more cost-efficient than Nvidia's.
Google is perhaps the cloud provider most committed to its own silicon, using Tensor Processing Units (TPUs) since 2015 to train and deploy AI models. The company has developed several versions of its chips, including the sixth version, Trillium.
Google also uses Nvidia chips and offers them through its cloud, showing that the company isn't entirely cutting ties with its traditional supplier.
Readers also liked: Google Cloud Skills Boost Generative Ai
Apple and Qualcomm
Apple and Qualcomm are working together to make AI more efficient on devices. They're updating their chips to run AI more efficiently, which can have privacy and speed advantages.
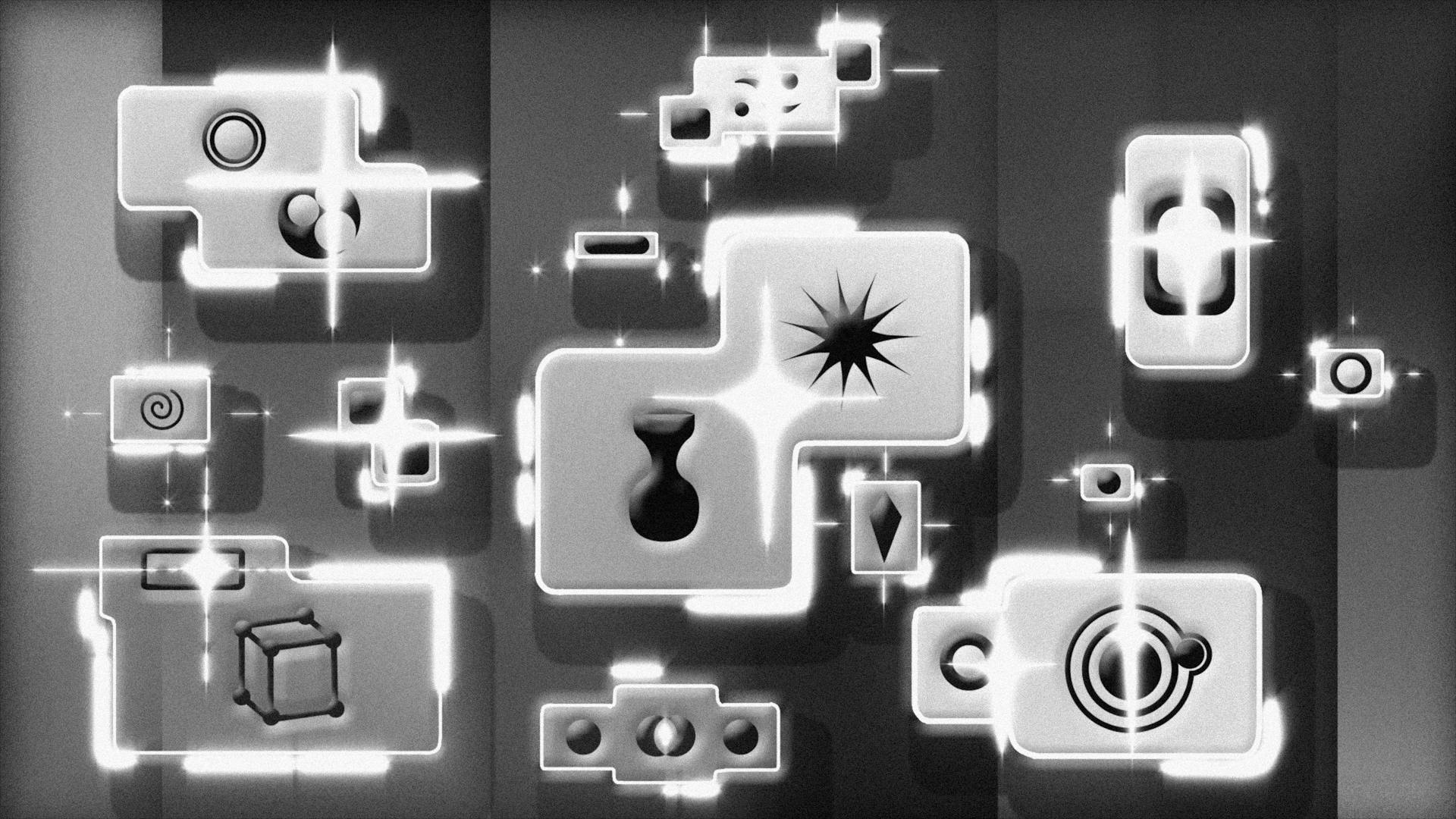
Big companies like Apple and Microsoft are developing "small models" that require less power and data and can run on a battery-powered device. These models are not as skilled as the latest version of ChatGPT, but they're useful for tasks like summarizing text or visual search.
Qualcomm recently announced a PC chip that will allow laptops to run Microsoft AI services on the device. This chip is a significant step towards making AI more portable and accessible.
Apple and Qualcomm's collaboration on neural processors is a game-changer for AI on the go. These processors can run AI models on devices without needing a massive cluster of powerful GPUs.
Qualcomm has also invested in lower-power processors to run AI algorithms outside of a smartphone or laptop. This investment will likely lead to more innovative AI applications in the future.
Discover more: Power Bi Generative Ai
Business and Adoption
The adoption of generative AI chips presents a unique set of challenges, despite their benefits. Organizations must navigate complexities such as the economics of adoption, algorithm efficiency, and hardware advancements.
A fresh viewpoint: Generative Ai Adoption
McKinsey analysis estimates that by 2030, the total gen AI compute demand could reach 25x10 FLOPs, with 70 percent from B2C applications and 30 percent from B2B applications. This underscores the importance of developing sustainable business models for companies looking to leverage gen AI.
A base adoption scenario assumes steady technological advancements, favorable regulatory developments, and growing user acceptance, leading to an estimated 28 billion interactions in 2030. This is twice the forecast daily number of online search queries.
If this caught your attention, see: Use Generative Ai
Business Intelligence for Enterprises
Business Intelligence for Enterprises is all about using data to make informed decisions. It's a crucial aspect of business and adoption.
Enterprises can use Business Intelligence to analyze their data and gain insights that can help them improve their operations and make better decisions. This can be done through various tools and technologies such as data visualization, reporting, and predictive analytics.
Business Intelligence can help enterprises identify trends and patterns in their data that might not be immediately apparent. For example, a company might use Business Intelligence to analyze customer data and identify which products are most popular among certain demographics.
Readers also liked: Generative Ai in Business
By using Business Intelligence, enterprises can make data-driven decisions that can help them stay ahead of the competition. This can be especially important in today's fast-paced business environment where change is constant.
Business Intelligence can also help enterprises optimize their processes and improve efficiency. For instance, a company might use Business Intelligence to analyze its supply chain data and identify areas where it can reduce costs and improve delivery times.
Intriguing read: Generative Ai for Business Leaders
B2C Compute Demand Scenarios
By 2030, the expected average number of daily interactions per smartphone user is ten for basic consumer applications, such as creating an email draft.
McKinsey analysis estimates the number of interactions to be approximately twice the forecast daily number of online search queries (approximately 28 billion) in 2030.
The base B2C scenario assumes steady technological advancements, favorable regulatory developments, and continuously growing user acceptance.
In the conservative adoption scenario, cautious consumer adoption due to ongoing concerns related to data privacy, regulatory developments, and incremental technology improvements could lead to half the number of interactions of the base case.
The accelerated adoption scenario suggests a high degree of trust in the technology and widespread user acceptance, driven by attractive new business models, substantial technological advancements, and compelling user experiences, potentially leading to a 150% higher adoption rate of the base case.
Frequently Asked Questions
What is a gen AI chip?
A Gen AI chip is a specialized processor designed to accelerate the performance of generative artificial intelligence models, enabling faster and more efficient content creation. This chip technology is crucial for powering the next generation of AI applications.
What is the most powerful AI chip?
The NVIDIA Blackwell B200 is considered the world's most powerful AI chip, capable of revolutionizing various AI applications. Its groundbreaking GPU technology makes it a game-changer in the field of artificial intelligence.
Sources
- Gen AI is hitting limits but these new chipmakers can help - (globalventuring.com)
- Generative AI: The next S-curve for the semiconductor ... (mckinsey.com)
- raised (techcrunch.com)
- What Are AI Chips? Evolution, Benefits & Top Manufacturers (run.ai)
- "Generative AI Chips: Revolutionizing Innovation & Efficiecy (linkedin.com)
Featured Images: pexels.com