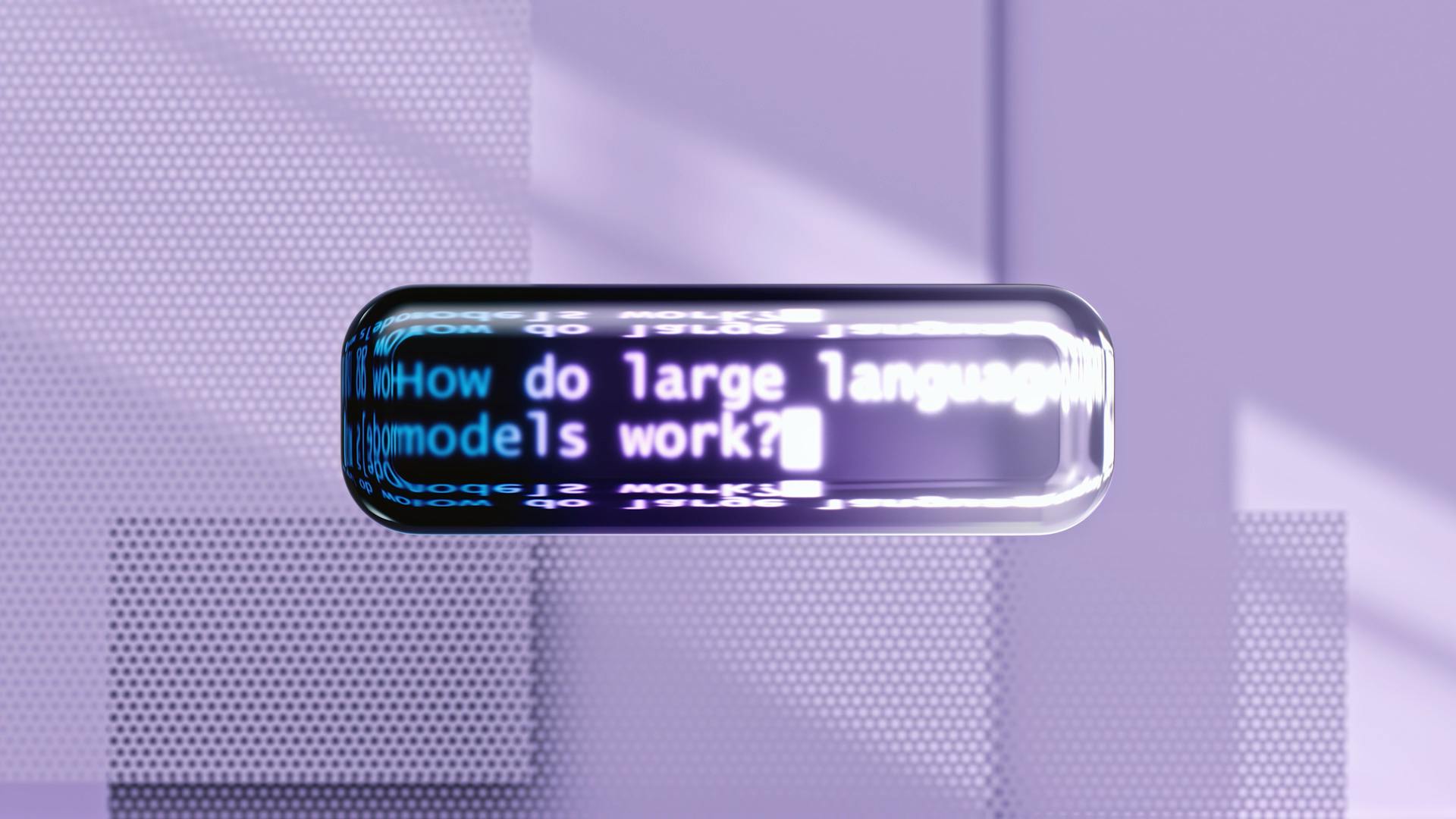
The generative AI landscape is vast and rapidly evolving. Generative AI models are designed to produce new, original content such as images, music, and text.
These models are trained on vast amounts of data, which enables them to learn patterns and relationships that humans may not have noticed. This training process can be time-consuming and computationally expensive.
Generative AI has many applications, including art, design, and even healthcare. In the field of art, generative AI can be used to create new and innovative pieces of art.
Generative AI models can be broadly classified into two categories: adversarial and non-adversarial. Adversarial models, such as Generative Adversarial Networks (GANs), use a competitive process to generate new content, while non-adversarial models use a single process to generate content.
The choice of model depends on the specific application and the desired outcome.
On a similar theme: Travel Industry and the Use of Generative Ai
What is Generative AI?
Generative AI is a type of AI that uses algorithms to generate new content, such as images, music, or text, based on a set of inputs or prompts.
These algorithms can learn patterns and relationships from existing data, allowing them to create something entirely new. Generative AI is often used in applications such as art, music, and writing.
The first generative AI models were developed in the 1960s, but it wasn't until the rise of deep learning in the 2010s that generative AI started to gain traction.
Applications and Use Cases
Generative AI has numerous applications across various industries, including information technology, marketing and sales, customer support, and product development. These applications can increase productivity and economic efficiency.
In the near future, the most promising domains for generative AI applications are IT, marketing and sales, customer support, and product development. IT teams can gain from automated documentation and code, while customer service can utilize virtual assistants and personalized chatbots to answer natural language requests and customer inquiries.
Generative AI is also being used in gaming to create new levels, maps, dialogue, and storylines. For example, a game might use a Gen-AI model to create a new, unique level for a player to explore each time they play.
Check this out: Use Generative Ai
Here are some examples of generative AI applications:
- Text: Generative AI can produce human-like text based on specific prompts, such as OpenAI's GPT (GPT-3.5 and GPT-4) and Google's PaLM 2.
- Images: These models can generate images ranging from realistic human faces and artistic creations to photorealistic scenes, all based on textual descriptions, such as OpenAI's DALL-E 2.
- Videos: Although currently limited to short clips, generative AI can create videos from textual descriptions, such as Kaiber and Runway.
- Audio: Generative AI is capable of creating audio content in the form of music and speech, such as Google's MusicLM and OpenAI's MuseNet.
Generative AI can also be used in various other areas, including cybersecurity, IT operations, and customer service. It can create synthetic training data for cybersecurity systems, automate tasks like network anomaly detection and incident response, and provide personalized support to customers.
Broaden your view: Generative Ai Cybersecurity
Examples of Generative AI
Generative AI has a wide range of applications across various industries. It can be used to create new art and music, generate new levels or maps in games, and even create new virtual environments. Generative AI can also be used to create new content in various forms, such as text, images, videos, audio, software code, and design.
One of the most prominent examples of generative AI is OpenAI's GPT, which can produce human-like text based on specific prompts. Other examples include Google's PaLM 2, Adobe Firefly, Midjourney, and Stable Diffusion, which can generate images ranging from realistic human faces to photorealistic scenes.
Curious to learn more? Check out: How Multimodal Used in Generative Ai
Generative AI can also be used to create videos from textual descriptions, and early platforms in text-to-video generation include Kaiber, Runway, Genmo, and Pika Labs. In the field of music generation, models such as Google's MusicLM, OpenAI's MuseNet, and Meta's AudioCraft are widely utilized.
Here are some examples of generative AI applications across different categories:
- Text: OpenAI's GPT, Google's PaLM 2, and ChatGPT
- Images: OpenAI's DALL-E 2, Adobe Firefly, Midjourney, and Stable Diffusion
- Videos: Kaiber, Runway, Genmo, and Pika Labs
- Audio: Google's MusicLM, OpenAI's MuseNet, and Meta's AudioCraft
- Software Code: GitHub Copilot and Amazon CodeWhisperer
- Design: NVIDIA's GET3D, DreamFusion, and RoomGPT
These are just a few examples of the many applications of generative AI. As the technology continues to evolve, we can expect to see even more innovative uses of generative AI in the future.
Supply Chain
Generative AI can analyze historical sales data to help businesses make more informed decisions about their supply chain operations.
By leveraging generative AI, companies can optimize various stages of the supply chain, such as demand forecasting and supply chain optimization.
Generative AI can also automate clerical work, freeing up employees to focus on more strategic and high-value tasks.
With generative AI, businesses can predict operational results and factor in tariffs into operational costs, giving them a more accurate picture of their financial situation.
This can lead to significant cost savings and improved efficiency in the supply chain.
Here's an interesting read: Generative Ai in the Supply Chain
Market and Industry Impact
The generative AI market is projected to grow at a staggering 47.5% CAGR, increasing from $43.87 billion in 2023 to $667.96 billion by 2030. This rapid growth is driven by advancements in technologies like super-resolution, text-to-image generation, and text-to-video conversion.
Boston Consulting Group estimates that the generative AI market size will reach $60 billion by 2025 and double to $120 billion by 2027, representing a 66% compound annual growth rate (CAGR) from 2022 to 2027. This significant increase will make up 30% of the total AI market by 2025.
Generative AI could contribute between $2.6 trillion to $4.4 trillion to the GDP in advanced economies, amounting to 4% to 7% of the overall GDP, according to McKinsey's estimates.
A unique perspective: Telltale Words Identify Generative Ai Text
Industry Function Impact
The impact of generative AI on different industry functions is significant. Generative AI can transform customer service by improving productivity and providing personalized support.
Customers can quickly get relevant information in their preferred language through conversational search. Automate responses and summaries, empowering agents to provide better support.
AI can generate content and suggestions for customer service tools. Call centre optimization is another area where AI can make a difference, analyzing data and providing insights to improve performance.
AI considers customer history to provide tailored information in their preferred format. This can lead to improved customer satisfaction and loyalty.
Here are some of the key industry functions impacted by generative AI:
The impact of generative AI on different industry functions will vary depending on the degree of automation and the kind of work that other functions are performing.
Risk and Legal
Generative AI can help businesses remain compliant with regulations by using it for regulatory monitoring.
Compliance and regulatory monitoring are just one of the many potential legal use cases for generative AI, alongside contract analysis and negotiation, document drafting and review, due diligence, intellectual property management, legal research, and legal chatbots.
Automating document drafting can improve the efficiency of legal work, allowing lawyers to focus on higher-value tasks.
You might enjoy: Free Generative Ai Application for Document Extraction
Contract analysis and negotiation can also be improved with generative AI, helping businesses navigate complex agreements and identify potential risks.
By protecting intellectual property, generative AI can help businesses safeguard their creative and innovative work.
Conducting legal research more efficiently is another benefit of generative AI, allowing lawyers to access a vast amount of information quickly and accurately.
Essential legal guidance can be provided to clients through legal chatbots, offering support and answers to common questions.
If this caught your attention, see: Legal Implications of Ai
Talent and Organization
Generative AI is transforming talent management by allowing for accurate evaluation and prediction of employee performance.
This means that HR teams can rely on data-driven insights to make informed decisions about employee development and job assignments.
With personalized training programs, employees can receive tailored support to help them grow in their roles.
Generative AI also helps hiring managers by providing data-driven job requirements and assistance with the recruitment process.
By leveraging these capabilities, organizations can create more effective job designs and HR practices that drive business success.
Consider reading: Synthetic Data Generative Ai
Technology and Infrastructure
The technology and infrastructure behind generative AI are crucial for its development and deployment. The infrastructure layer of generative AI encompasses semiconductors, networking, storage, databases, and cloud services.
Cloud platforms have become the go-to solution for businesses to access computational power and manage expenses quickly. Most of the work to create, fine-tune, and operate large AI models occurs in the cloud due to the cost and scarcity of GPUs and TPUs. Cloud service providers like AWS, Microsoft Azure, and Google Cloud offer computing resources, networking, storage, databases, and various other services that enable the training and deployment of complex generative AI models.
Cloud service providers have developed platform services to allow companies to access necessary foundation models and train and customize their own models for specific applications. Examples of these platform services include Azure OpenAI Service, Amazon Bedrock, and Google Cloud's Vertex AI. These platforms offer a range of tools and services for building, training, and deploying generative AI models.
Check this out: Google Cloud Skills Boost Generative Ai
Here are some examples of cloud services offered by leading providers:
- AWS: Amazon offers EC2 P5 instances, which are powered by the NVIDIA H100 Tensor Core GPU, delivering up to 20 exaFLOPS of compute performance
- Microsoft Azure: They offer the NVIDIA A10G v5 instances, which are powered by the NVIDIA A10G Tensor Core GPU, providing up to 250 teraFLOPS of compute performance
- Google Cloud: Through G2 virtual machines, they offer NVIDIA’s L4 Tensor Core GPU, capable of up to 242 teraFLOPS of compute performance
Storage plays a vital role in the training and inference phases of generative AI models, enabling the retention of vast amounts of training data, model parameters, and intermediate computations.
Curious to learn more? Check out: Learning Generative Ai
How They Work in Practice
Generative AI models learn from a large dataset of examples and use that knowledge to generate new data that is similar to the examples in the training dataset.
These models are typically trained using a type of machine learning algorithm known as a generative model, which can include generative adversarial networks (GANs), variational autoencoders (VAEs), and autoregressive models.
For instance, a generative model trained on a dataset of images of faces might learn the general structure and appearance of faces, then use that knowledge to generate new, previously unseen faces that look realistic and plausible.
Generative models are used in a variety of applications, including image generation, natural language processing, and music generation.
A fresh viewpoint: Geophysics Velocity Model Prediciton Using Generative Ai
Here are some common types of generative models and their applications:
Generative models are particularly useful for tasks where it is difficult or expensive to generate new data manually, such as creating new designs for products or generating realistic-sounding speech.
Databases
Databases play a vital role in generative AI, particularly non-relational (NoSQL) types, which facilitate the efficient storage and retrieval of large, unstructured datasets required to train complex models like Transformers.
These databases are highly performant and scalable, as seen in the use of Azure Cosmos DB by OpenAI for dynamically scaling the ChatGPT service.
Efficient data storage and retrieval are crucial for generative AI, and databases like Azure Cosmos DB help bridge this gap.
Azure Cosmos DB is a NoSQL database within Azure that is capable of handling large amounts of data and providing high performance.
Non-relational databases like Azure Cosmos DB are well-suited for generative AI because they can handle the large amounts of unstructured data required to train complex models.
See what others are reading: Generative Ai for Data Analytics
In particular, Azure Cosmos DB is designed to handle high volumes of data and provide low latency, making it an ideal choice for generative AI applications.
Here are some key features of non-relational databases like Azure Cosmos DB:
- High performance: Azure Cosmos DB can handle high volumes of data and provide low latency
- Scalability: Azure Cosmos DB can scale dynamically to handle changing workloads
- Flexibility: Azure Cosmos DB can handle a wide range of data formats and structures
Overall, non-relational databases like Azure Cosmos DB are a critical component of the infrastructure layer of generative AI.
Cloud Platforms for Model Fine-Tuning
Cloud platforms have revolutionized the way we fine-tune AI models, making it easier and more accessible than ever before. Cloud service providers like Azure, Amazon, and Google have developed platform services that allow companies to access foundation models and train and customize their own models for specific applications.
Azure OpenAI Service is a cloud-based service that offers access to OpenAI's foundation models, enabling users to create applications within the Azure portal. This includes the GPT family of LLMs for text generation and Codex for code generation.
Amazon Bedrock is another platform that supports building and scaling generative AI applications using foundation models like Anthropic's Claude, Stability AI's Stable Diffusion, and Amazon Titan.
Recommended read: Foundations and Applications of Generative Ai Grants
Google Cloud's Vertex AI is a managed ML platform that offers tools and services for building, training, and deploying generative AI models, including PaLM for text generation and Imagen for image generation.
Here are some key features of these cloud platforms:
These cloud platforms have made it possible for companies to access the necessary tools and resources to fine-tune their AI models, without having to invest billions of dollars and years of effort in developing these models from scratch.
Computer Hardware
Computer hardware plays a vital role in generative AI systems, which require massive quantities of data to generate content. This is a task that conventional computer hardware is incapable of handling.
To process the vast amount of data across billions of parameters simultaneously, extensive clusters of GPUs or TPUs equipped with specialized accelerator chips are required. The chip design market is largely dominated by NVIDIA and Google, while TSMC is responsible for producing nearly all accelerator chips.
New entrants into the market encounter significant initial expenses for research and development, while traditional hardware designers must acquire specialized expertise, knowledge, and computational capabilities to cater to the generative AI industry. This can be a significant barrier to entry.
Key examples of hardware accelerators used in generative AI include:
These hardware accelerators are particularly well-suited for tasks that can be broken down into smaller, parallel tasks, such as those found in generative AI.
vs
In the generative AI landscape, two approaches are gaining traction: model-based and data-based methods. Model-based methods rely on pre-defined models to generate content, whereas data-based methods use large datasets to learn patterns and generate new content.
The model-based approach is often more efficient and scalable, but can struggle with creativity and nuance. Data-based methods, on the other hand, can produce more diverse and realistic results, but require massive amounts of data to train.
One key difference between the two approaches is their reliance on human input. Model-based methods often require explicit programming and fine-tuning, whereas data-based methods can learn from large datasets with minimal human intervention.
The choice between model-based and data-based methods ultimately depends on the specific use case and desired outcome.
A different take: Generative Ai Human Creativity and Art Google Scholar
Future and Emerging Trends
The future of generative AI is looking incredibly exciting! With advancements in Explainable AI (XAI), users will have a greater understanding of how generative models arrive at their outputs, fostering trust and wider adoption of AI-generated content.
Easier-to-use generative AI tools are making the technology more accessible to a wider range of developers, even those without extensive AI expertise. This democratization of AI development is further accelerating innovation and the integration of generative AI into various IT applications.
Here are some key emerging trends that are expected to shape the future of generative AI:
- Explainable AI (XAI)
- Democratization of AI Development
These trends are driven by the rapid growth of the generative AI market, which is being fueled by advancements in technology and increasing demand across various sectors.
The Future Ahead?
Explainable AI (XAI) is on the rise, giving users a deeper understanding of how generative models work, which will boost trust and adoption of AI-generated content.
This is particularly exciting for developers, as it means they'll be able to create more transparent and reliable AI systems.
The development of easier-to-use generative AI tools is making the technology more accessible to a wider range of developers, even those without extensive AI expertise.
This democratization of AI development will accelerate innovation and the integration of generative AI into various IT applications.
Generative AI applications are transforming industries by enhancing user experiences, streamlining workflows, and providing valuable insights from complex datasets.
From gaming and entertainment to design and healthcare, the possibilities are endless.
Here are some key trends shaping the future of generative AI:
- Super-resolution, text-to-image generation, and text-to-video conversion are driving growth in various sectors.
- Prediction algorithms are highly effective in analyzing complex datasets, identifying patterns, and generating valuable predictions.
- Advanced generative models, including Deep Convolutional GANs (DCGANs) and StyleGANs, are generating high-quality and realistic images and videos.
The Future of Space Exploration
The Future of Space Exploration is a vast and complex topic, but one thing is certain: the challenges ahead for Gen-AI are numerous. Gen-AI models will face challenges such as improving the quality and diversity of their outputs, increasing speed, and making them more robust and reliable.
These models will also need to develop a better understanding of the underlying structure and context of the data they're working with, in order to produce more accurate and coherent outputs. This will be crucial in the education space, where students may use Gen-AI to aid in their academic work, raising questions about the authenticity of their assignments.
The risk of bias in the generated data is a significant concern, particularly if the training data is not diverse or representative enough. This could lead to the perpetuation of misinformation, making it even more challenging to identify accurate sources.
Here are some potential drawbacks of Gen-AI:
- The risk of bias in the generated data, if the training data is not diverse or representative enough.
- Concerns about the potential for generative AI to replace human labor in certain industries, leading to job loss.
- The potential for Gen-AI to be used for malicious purposes, such as creating fake news or impersonating individuals.
It's worth noting that Gen-AI has the potential to replace millions of jobs, from designers to producers to artists, but it's unlikely to completely eliminate the creative industry.
Frequently Asked Questions
What is the AI technology landscape in 2024?
The AI technology landscape in 2024 shows growing adoption and acceptance, with 90% of business leaders agreeing that AI enhances employee skills. Concerns about job displacement have eased, but more businesspeople are now using generative AI beyond IT.
Where do the OpenAI models fit into the AI landscape?
OpenAI models stand out in the AI landscape due to their vast and diverse training data, setting them apart from competitors. This unique advantage enables them to learn and evolve more effectively, making them a key player in the field.
Sources
- Generative AI: Reshaping the IT Landscape (sawyersolutionsllc.com)
- Generative AI Applications Landscape (xenonstack.com)
- RoomGPT (roomgpt.io)
- DreamFusion (dreamfusion3d.github.io)
- NVIDIA’s GET3D (nv-tlabs.github.io)
- Amazon CodeWhisperer (amazon.com)
- Tacotron (pytorch.org)
- ElevenLabs (elevenlabs.io)
- WaveNet (deepmind.com)
- MuseNet (openai.com)
- MusicLM (blog.google)
- Pika Labs (pika.art)
- Genmo (genmo.ai)
- Runway (runwayml.com)
- Kaiber (kaiber.ai)
- Stable Diffusion (stablediffusionweb.com)
- Midjourney (midjourney.com)
- Adobe Firefly (adobe.com)
- DALL-E 2 (openai.com)
- Bard (google.com)
- ChatGPT (openai.com)
- projected (bcg.com)
- Einstein GPT (www.salesforce.com)
- Microsoft 365 Copilot (microsoft.com)
- Med-PaLM 2 (research.google)
- BloombergGPT (bloomberg.com)
- Jasper AI (jasper.ai)
- Character.ai (character.ai)
- Open LLM Leaderboard (huggingface.co)
- Cerebras-GPT (cerebras.net)
- Stable Diffusion XL (stability.ai)
- Dolly 2.0 (databricks.com)
- Llama 2 (meta.com)
- Mapping the Generative AI landscape (antler.co)
- Ali (substack.com)
Featured Images: pexels.com