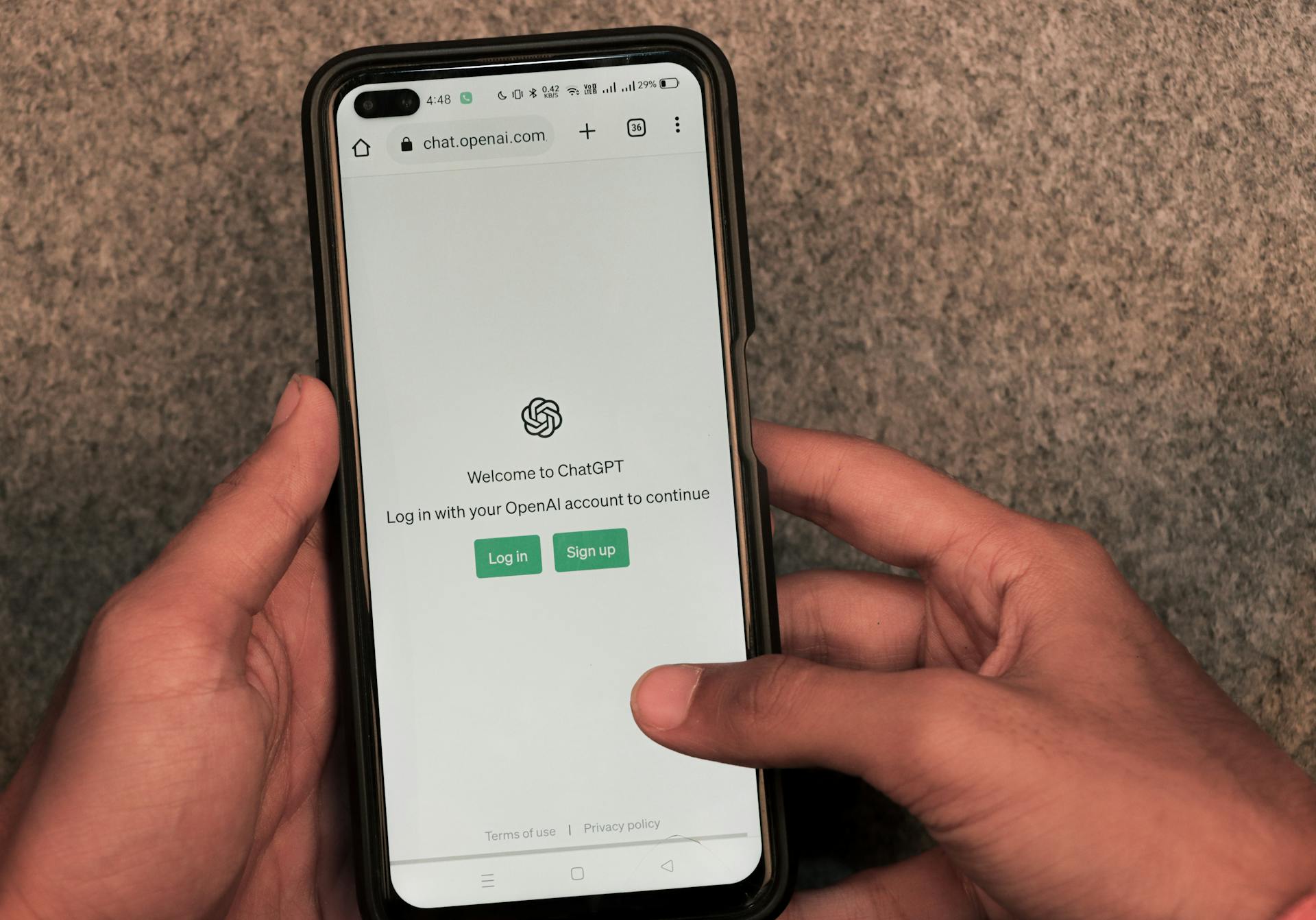
The total cost of ownership for AI software solutions can be a significant investment, with some solutions costing upwards of $100,000 per year.
This cost can be broken down into several components, including the initial purchase or subscription fee, maintenance and support costs, and the cost of training and implementing the solution.
The initial purchase or subscription fee can vary widely, from a few thousand dollars to tens of thousands of dollars, depending on the complexity of the solution and the size of the organization.
Maintenance and support costs can also add up quickly, with some solutions requiring ongoing software updates and technical support.
The cost of training and implementing the solution can also be significant, with some solutions requiring specialized training and consulting services.
Expand your knowledge: Ai and Ml Solutions
Cost Factors
The cost of AI software can be a daunting topic, but breaking it down into its core components can make it more manageable. The primary cost factors in an AI project include necessary hardware, licensing fees, costs of data collection and preparation, wages for skilled AI engineers, and cybersecurity measures.
Related reading: How Much Does Claude 3 Cost
One of the biggest expenses is the cost of data collection and preparation, which can be a significant undertaking. The cost of data maintenance is also a factor to consider, including hardware maintenance, software maintenance, data maintenance, and expertise.
The type of AI solution chosen can also impact the cost, with custom-built solutions requiring more effort to design, develop, and maintain. The cost of implementing AI can range from a basic version to a more advanced, feature-rich option, with the cost increasing relative to the range of features and capabilities provided.
The cost of AI software development can vary widely, with custom AI solutions costing anywhere from $6000 to over $500,000. Third-party AI software, on the other hand, can cost anywhere from $40,000 per year to as little as $0.
Here are the key factors that impact the cost of AI:
- Custom-built AI solutions require more effort to design, develop, and maintain.
- The type of AI solution chosen can impact the cost.
- The cost of implementing AI can range from a basic version to a more advanced, feature-rich option.
- The cost of AI software development can vary widely.
Ongoing support and training expenses can also add up, with costs ranging from $2000 to $4500, depending on the scope of the project. The cost of AI maintenance can be broken down into four main factors: hardware maintenance, software maintenance, data maintenance, and expertise.
Pricing Models
Gen AI tools charge enterprises based on the number of characters or tokens in input or output text.
Character-based billing is used by some solutions, such as Gen AI tools driven by Google's Vertex AI, which bill users based on the number of characters in the input and output text.
This includes letters, numbers, spaces, and punctuation marks as a character. For example, the generative AI pricing for the PaLM 2 for Text model supported by Vertex starts from $0.0005 per 1,000 characters for input and output text.
Token-based billing is used by more advanced Gen AI tools, which break down text into tokens instead of characters. A token can be a punctuation mark, a word, or part of a word.
OpenAI defines a token as a group of approximately four characters. For example, the words "brought" and "flowers" in the sentence "Tom has brought Jill flowers." would thus consist of eight tokens.
Worth a look: Huggingface Vertex Ai
The cost of such generative AI solutions largely depends on your chosen language model. OpenAI's GPT-4 Turbo charges $0.01 per 1,000 tokens for input text and $0.03 per 1,000 tokens for output text.
Here's a comparison of the pricing models:
Gen AI services for visual content creation charge users per generated image, with fees tied to image size and quality.
Intriguing read: Ai Image Analysis Software
Project Considerations
AI project costs can be influenced by various factors, including the type of project, company size, and complexity of the AI solution. To give you a better idea, let's break down the key considerations.
The type of AI development project is a crucial factor in determining the cost. Training a large language model from scratch can be a costly endeavor, requiring a large amount of data, computational power, and time. Fine-tuning or adapting an existing language model can be much less expensive and yield better results.
The level of customization required also impacts the cost. Custom-built AI solutions require more effort to design, develop, and maintain. This can lead to higher costs compared to using off-the-shelf AI tools.
Explore further: Energy Based Model
Here are some factors to consider before investing in AI:
- What level of intelligence does your AI need? Basic automation tools are less costly than more advanced systems.
- What is the quantity and quality of data required to train your AI? Data collection, storage, and management is one of the most significant costs associated with any AI development project.
- What is your desired level of accuracy? Achieving 95% accuracy is fairly simple, but training and fine-tuning a model to reach higher levels of accuracy requires much more time, effort, and money.
- How complex is your AI implementation? Training an AI model to do one thing well is less costly than a solution that needs to be more flexible and integrate with more business functions.
Project Complexity
Project complexity is a significant factor in determining the cost of an AI project. It can range from simple chatbots that automate customer interactions to more complex systems that analyze language and image recognition.
Building a chatbot typically involves integrating existing natural language processing models and frameworks, which can significantly reduce development time and costs. For example, Google Dialogflow offers a free standard plan for small and medium-sized businesses, and the Enterprise edition costs $0.0002 per request.
More complex AI systems, on the other hand, require more resources and expertise. They may involve data collection and labeling large datasets for training AI models, which can be costly. It may involve purchasing proprietary datasets, hiring data annotators, or investing in tools for data labeling automation.
The costs associated with data collection and labeling can be substantial, with estimates ranging from $10,000 to $50,000 or more, depending on the complexity of the project. Additionally, ensuring data quality and diversity adds to the expense, as it may require extensive validation and verification processes.
For your interest: Data Labeling Companies
Here's a rough estimate of the costs associated with different levels of project complexity:
Keep in mind that these estimates are rough and can vary widely depending on the specific requirements of the project. However, they give you an idea of the scale of costs associated with different levels of project complexity.
Level of Expertise
As you start planning your AI project, it's essential to consider the level of expertise required to bring it to life. Implementing AI solutions can be a costly affair, especially if you need to hire a team of data scientists.
The complexity of your project will greatly impact the level of expertise needed. For instance, building an AI system that analyzes language and image recognition requires more resources and expertise than a chatbot that automates customer interactions.
You may need to hire a team of data scientists or work with a third-party consultant, which can come at a high cost. Depending on your company's needs, the cost of expertise can be a significant factor to consider.
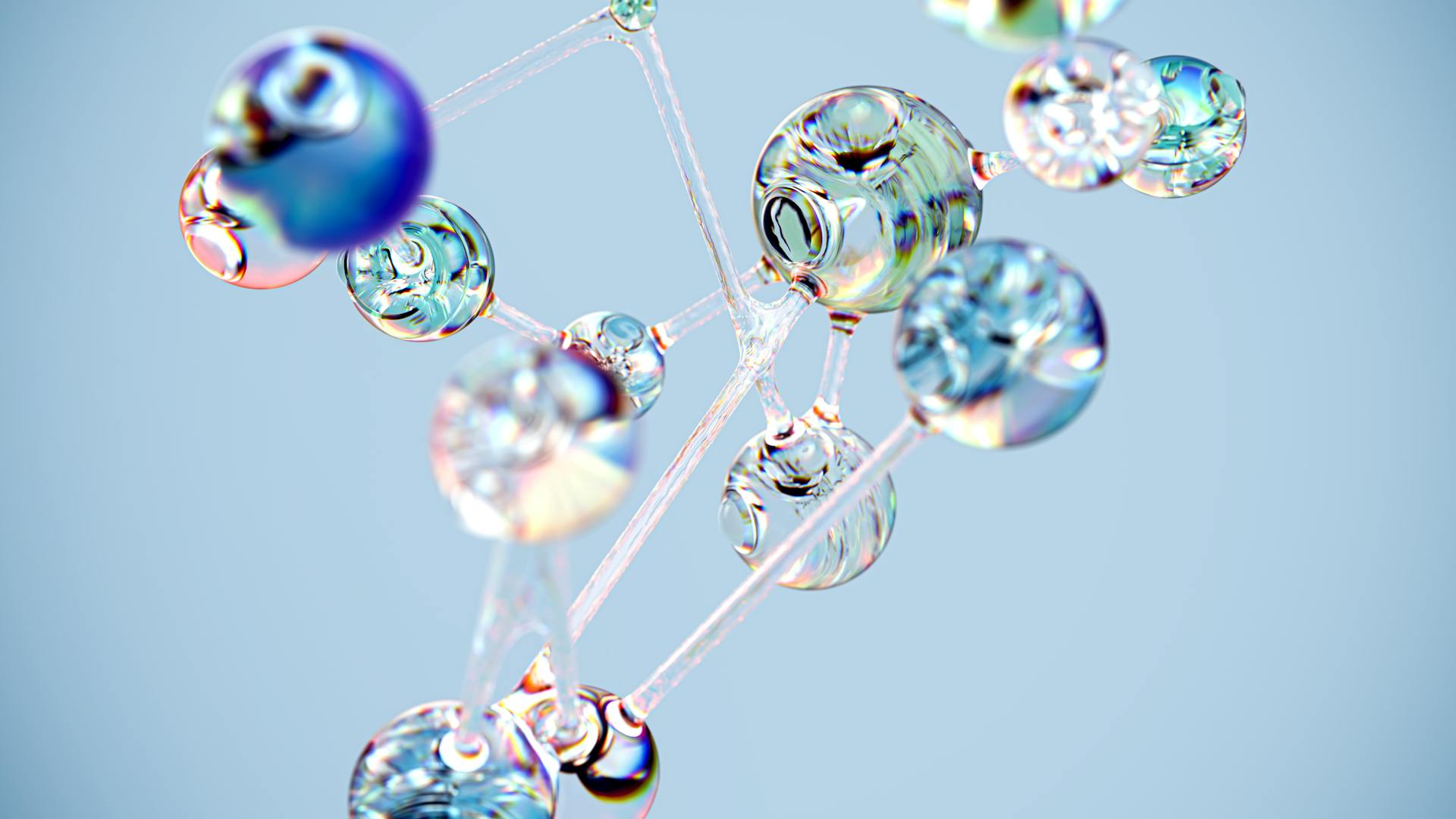
Here are some key factors to consider when evaluating the level of expertise needed for your project:
- Do you have in-house data scientists or will you need to hire them?
- Will you need to work with a third-party consultant or partner?
- What is the expected ROI for the project, and will it justify the cost of expertise?
By carefully considering the level of expertise required for your AI project, you can avoid costly mistakes and ensure a successful outcome.
Factors to Consider Before Investing
Investing in a project can be a daunting task, especially when it comes to AI. The primary cost factors in an AI project include necessary hardware, licensing fees, data collection costs, and wages for skilled AI engineers.
The cost of AI software development increases with the range of features and capabilities provided. A basic version can cost less, while a more advanced, feature-rich option can be more expensive.
Before investing in AI, consider the AI solution, level of expertise, integration with existing systems, and expected ROI. This will help you determine if AI is the right fit for your project.
To mitigate costs, consider lean approaches and scalability in AI implementation. Choosing AI tools and frameworks with community support can save on development time and costs compared to custom-built alternatives.
Here are some key factors to consider before investing in AI:
- The AI solution
- Level of expertise
- Integration with existing systems
- Expected ROI
Additionally, consider outsourcing tasks like data labeling to specialized service providers to reduce overall development costs.
Maintenance
Maintenance is a crucial aspect of AI software costs. It's not just a one-time expense, but an ongoing cost that can add up quickly.
The McKinsey study found that foundational AI models can cost $1-4 million per year on model maintenance. This is a significant expense that businesses need to factor in.
Software updates, patches, and bug fixes are necessary to keep the system up-to-date and running smoothly. This is another critical factor in AI software costs.
To put it into perspective, off-the-shelf AI software can cost $200,000 per year to maintain. This is a substantial cost that businesses need to be aware of.
There are ways to reduce AI maintenance costs, but it requires careful planning and consideration. Here are a few strategies to consider:
- Outsourcing AI development can help reduce maintenance costs.
- Leveraging open-source AI technologies can also help reduce costs.
- Investing in cloud-based AI solutions can be another cost-effective option.
Development and Infrastructure
Development and infrastructure costs can add up quickly. Developing or adapting an AI model requires significant computational resources and infrastructure.
The initial investment for high-performance hardware such as GPUs, TPUs, or other specialized hardware can be substantial. Cloud platforms like AWS, Google Cloud, or Azure charge based on usage, which can add up quickly for large-scale projects.
Setting up and maintaining servers and network infrastructure incurs additional costs. Implementing robust security measures to protect data and infrastructure is essential, adding to overall costs.
Here is a breakdown of the infrastructure costs:
- Hardware: Initial investment for high-performance hardware such as GPUs, TPUs, or other specialized hardware
- Cloud Services: Usage-based charges for cloud platforms like AWS, Google Cloud, or Azure
- Servers and Networking: Costs associated with setting up and maintaining servers and network infrastructure
- Security: Costs associated with implementing robust security measures to protect data and infrastructure
- Electricity: Operational costs associated with running high-performance hardware for extended periods
Algorithm Accuracy
Achieving higher accuracy in AI algorithms often requires more sophisticated algorithms, extensive testing, and fine-tuning of models.
These processes demand additional resources, including specialized expertise, computational power, and time. This is because AI algorithms need to be trained and updated regularly to maintain high accuracy levels over time.
For instance, a customer support chatbot is expected to handle up to around 60% of routine tasks and simple user queries, which requires high fluency. However, for complex issues, a human specialist is always waiting on the other end of the line.
Developers may prioritize efficiency over precision if the AI's tasks are less critical or do not require great accuracy. This approach can reduce costs by simplifying algorithms and reducing the need for extensive testing and optimization.
Related reading: How to Use Ai in Software Testing
Application Development
Developing the application or platform is a crucial step in the AI app development process. This phase involves significant costs, including programming the backend and frontend, integrating your AI model, and hiring developers and UX/UI designers.
The costs associated with developing the application or platform can be substantial. According to the article, this phase will also require planning, wireframing, and prototyping.
Testing, QA, and maintenance stages are also part of the application development process. These stages are necessary to ensure your application is stable and functions as intended.
Working with a fully managed AI software development company can help simplify and streamline this process based on your needs and budget. This can help reduce costs and make the process more efficient.
Data Management
Data Management can be a daunting task, especially when working on AI projects. 80% of an AI project's time is spent on collecting, organizing, and labeling data.
Gathering large amounts of high-quality data can be a significant challenge, requiring substantial manual effort and cost. This is especially true for projects that are just starting out, with little to no existing data to draw from.
Data storage solutions can be expensive, especially when dealing with vast datasets. You'll need a reliable system to hold all your data, which can add up quickly.
Cleaning and labeling data is a crucial step before it's ready for use in AI projects. This process can be time-consuming and labor-intensive, but it's essential for getting accurate results.
Computational and Infrastructure
Computational and infrastructure costs can be a significant burden on any project. The initial investment for high-performance GPUs, TPUs, or other specialized hardware necessary for training and running AI models can be substantial.
Training and running a large language model (LLM) or other AI is highly resource intensive. This means you'll need to consider the costs of hardware, cloud services, servers, networking, security, and electricity.
The costs of cloud services can add up quickly for large-scale projects. Cloud platforms like AWS, Google Cloud, or Azure charge based on usage.
Setting up and maintaining servers and network infrastructure incurs additional costs. This is essential for running and training AI models, but it's not cheap.
Intriguing read: Ai Ml Development Services
Implementing robust security measures to protect data and infrastructure is essential. This adds to the overall costs of developing and running AI models.
Here's a breakdown of the key infrastructure costs:
- Hardware: High-performance GPUs, TPUs, or other specialized hardware
- Cloud Services: Usage-based costs on platforms like AWS, Google Cloud, or Azure
- Servers and Networking: Setting up and maintaining infrastructure
- Security: Implementing robust security measures to protect data and infrastructure
- Electricity: Consuming a significant amount of electricity to run high-performance hardware
Training
Training is a significant contributor to the cost of developing artificial intelligence, with costs ranging from $4 million to $200 million per training run, according to a McKinsey report.
The time it takes to train large language models (LLMs) depends on the expertise of data scientists, model size, and available computational power. This prolonged training time can result in higher costs.
Training an LLM from scratch is a costly endeavor, requiring a large amount of data, computational power, and time. Fine-tuning an existing LLM can be much less expensive and may yield better results.
Training costs can be broken down into several factors, including the cost of computing for data scientists, their expertise, and the time required for training. For example, training an AI model can cost $4 million to $200 million per training run, which includes computing costs for 4-6 data scientists working for 3-6 months.
Broaden your view: Ai Time Magazine
Fine-tuning an existing chatbot with company data and industry knowledge may only cost $100,000 to $6 million per training run. Using an off-the-shelf AI solution that only requires integration can avoid training costs altogether.
Here's a rough estimate of the costs associated with training LLMs:
Keep in mind that these estimates can vary depending on the specific requirements of your project.
Sources
- https://itrexgroup.com/blog/calculating-the-cost-of-generative-ai/
- https://www.future-processing.com/blog/ai-pricing-is-ai-expensive/
- https://flyaps.com/blog/how-much-does-ai-cost/
- https://gigster.com/blog/how-much-does-it-cost-to-develop-artificial-intelligence-applications/
- https://scribehow.com/library/cost-of-ai
Featured Images: pexels.com