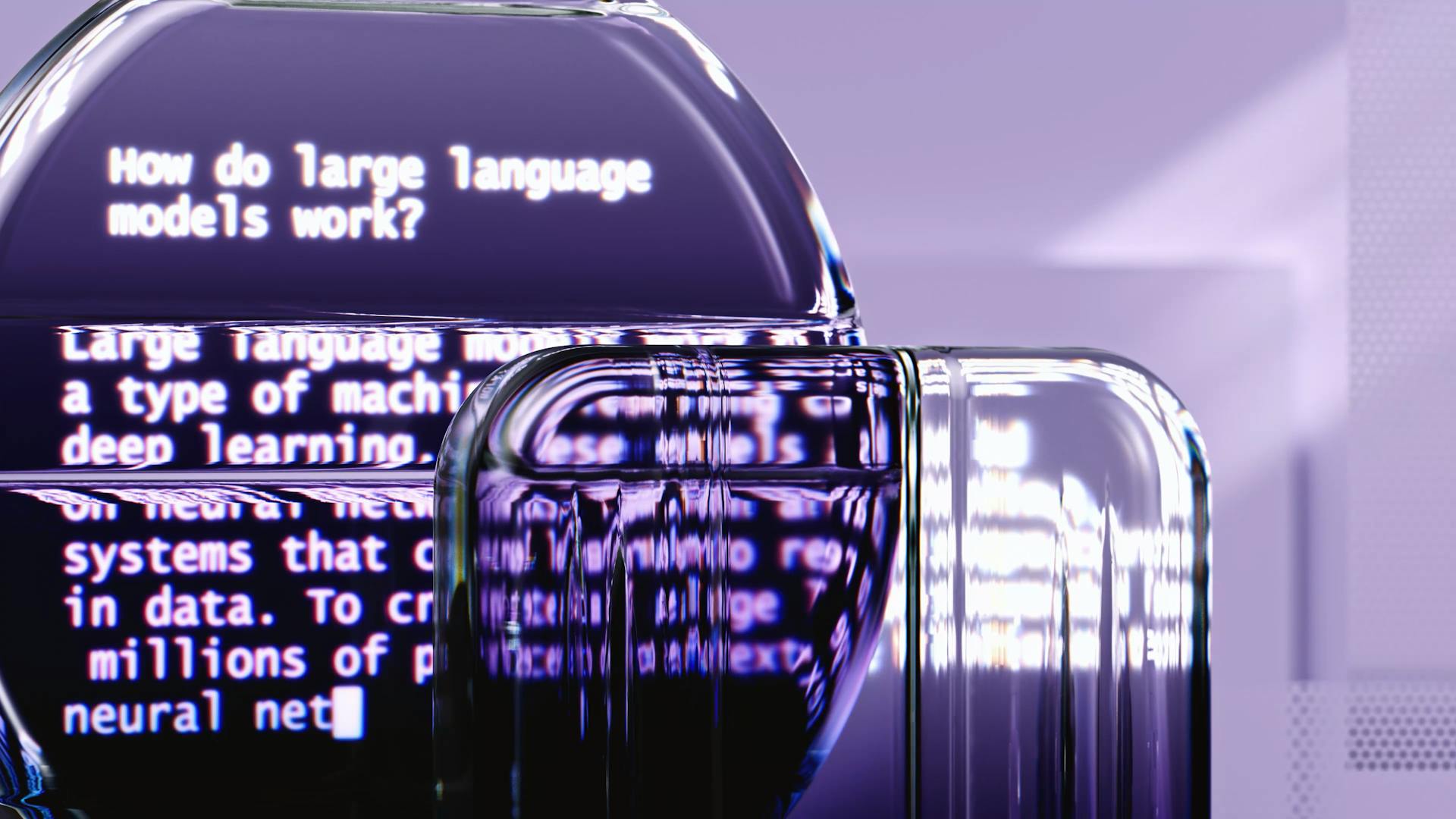
Generative AI has been making waves in the tech world, and it's essential to understand the basics before diving deeper. Generative AI models can create new content, such as images, music, or text, based on patterns and data they've learned from existing examples.
One of the key aspects of generative AI is its ability to learn from large datasets, which enables it to generate new content that's often indistinguishable from human-created work. This technology has far-reaching implications for various industries, from entertainment to education.
For instance, generative AI can be used to create realistic images or videos that can be used in advertising, film, or gaming. This can be particularly useful for companies looking to create engaging content without the need for extensive resources or expertise.
The possibilities with generative AI are vast, and it's exciting to think about the potential applications in the future.
A unique perspective: Google Announces New Generative Ai Search Capabilities for Doctors
Generative AI Basics
Generative AI is a type of artificial intelligence that creates new content, such as images, music, or text, based on a given input or pattern.
It works by learning from a large dataset and generating new data that is similar in style and structure. This process is called "generative modeling".
Generative AI has many applications, including art, music, and writing.
For your interest: Generative Ai for Music
Academic Artificial Intelligence
The academic discipline of artificial intelligence was established at a research workshop held at Dartmouth College in 1956. This marked the beginning of a long journey in the field of AI.
Harold Cohen created a computer program called AARON in the early 1970s, which generated paintings and was exhibited as a generative AI work. This was a pioneering effort in using AI to create artistic works.
Generative AI planning systems were a "relatively mature" technology by the early 1990s, using symbolic AI methods such as state space search and constraint satisfaction. They were used to generate crisis action plans for military use and process plans for manufacturing.
The field of machine learning has used both discriminative models and generative models since its inception. However, the emergence of deep learning in the late 2000s drove progress and research in tasks such as image classification and natural language processing.
For your interest: Chatgpt Openai's Generative Ai Chatbot Can Be Used for
Neural Nets (2014-2019)
In 2014, advancements such as the variational autoencoder and generative adversarial network produced the first practical deep neural networks capable of learning generative models.
These new models were a game-changer, allowing for the first time to output not only class labels for images but also entire images.
The Transformer network enabled further advancements in generative models, leading to the development of the first generative pre-trained transformer (GPT) in 2018.
GPT-1, as it was known, marked a significant milestone in the field of generative AI.
In 2019, GPT-2 was released, demonstrating the ability to generalize unsupervised to many different tasks as a Foundation model.
This breakthrough allowed for large neural networks to be trained using unsupervised learning or semi-supervised learning, rather than the supervised learning typical of earlier models.
Unsupervised learning removed the need for humans to manually label data, enabling the training of larger networks.
Worth a look: Pre Trained Multi Task Generative Ai
Content Quality
Content Quality is crucial in Generative AI, and it's often overlooked.
High-quality training data is essential for generating accurate and relevant content.
A Generative AI model's performance is only as good as the data it's trained on, which is why data quality is vital.
You can't train a model on low-quality data and expect it to produce high-quality content.
In fact, a study showed that even a small amount of high-quality data can significantly improve a model's performance.
Discover more: Generative Ai Content
Applications and Uses
Generative AI news can be used in various applications, from content creation to data analysis.
One notable application is in journalism, where AI can help generate news articles, allowing for faster and more efficient reporting.
AI-powered chatbots can also use generative AI news to provide users with personalized news feeds, tailoring the content to their interests.
For example, a user can ask an AI chatbot to provide news on a specific topic, and the chatbot can generate a summary of relevant articles.
Additional reading: Generative Ai Fake News
Software and Hardware
Generative AI models are used to power a variety of products, including chatbots like ChatGPT, programming tools like GitHub Copilot, and text-to-image products like Midjourney.
These models have also been integrated into existing commercially available products, such as Microsoft Office (Microsoft Copilot), Google Photos, and the Adobe Suite (Adobe Firefly).
The LLaMA language model is an example of an open-source software that can be run on personal computers, including a Raspberry Pi 4.
Additional reading: Premiere Pro Generative Ai
Stable Diffusion is another open-source model that can be run on an iPhone 11.
Larger models with tens of billions of parameters can run on laptop or desktop computers, but may require accelerators like NVIDIA's GPU chips or Apple's Neural Engine to achieve an acceptable speed.
The advantages of running generative AI locally include protection of privacy and intellectual property, and avoidance of rate limiting and censorship.
The subreddit r/LocalLLaMA is a community that focuses on using consumer-grade gaming graphics cards to run generative AI models locally.
Modalities
Generative AI systems can be constructed using unsupervised machine learning, such as neural network architectures like GANs, VAE, and Transformer.
The capabilities of a generative AI system depend on the modality or type of the data set used. For example, one version of OpenAI's GPT-4 accepts both text and image inputs, making it a multimodal system.
Generative AI can be either unimodal or multimodal; unimodal systems take only one type of input, whereas multimodal systems can take more than one type of input. This is seen in the case of Midjourney, DALL-E 2, and Stable Diffusion, which can generate plausible disinformation images when prompted to do so.
Here's an interesting read: What Is One Challenge in Ensuring Fairness in Generative Ai
The modality of a generative AI system can greatly impact its capabilities and applications. For instance, generative AI trained on annotated video can generate temporally-coherent, detailed, and photorealistic video clips, as seen in Sora by OpenAI, Gen-1 and Gen-2 by Runway, and Make-A-Video by Meta Platforms.
Generative AI systems can be trained on various types of data, including images, videos, audio clips, and text. The type of data used can determine the type of output the system can produce.
Additional reading: Ai Generative Fill for Video
3D Modeling
3D Modeling is a powerful application of artificial intelligence. Artificially intelligent computer-aided design (CAD) can automate 3D modeling using text-to-3D, image-to-3D, and video-to-3D.
AI-based CAD libraries can be developed using linked open data of schematics and diagrams. This allows for more efficient design and collaboration.
AI CAD assistants are used as tools to help streamline workflow. They can save designers time and effort by automating repetitive tasks.
Explore further: Generative Ai for 3d Models
Energy and Environment
Generative models are having a significant environmental impact, with high CO2 emissions and large amounts of freshwater used for data centers.
Scientists and journalists are sounding the alarm about the environmental costs of developing and deploying these models. They're concerned that as these models become more widespread, their environmental impact will only increase.
High amounts of electricity are being used to power data centers, which is contributing to the problem. In fact, the electricity usage is expected to rise as more models are incorporated into search engines and chatbots.
Factoring in potential environmental costs prior to model development or data collection could help mitigate the problem. This means considering the carbon footprint of a model before it's even built.
Increasing efficiency in data centers is also a key strategy for reducing energy usage. By making data centers more efficient, we can reduce the amount of electricity and water being used.
Building more efficient machine learning models is another way to reduce the environmental impact. This can be achieved by minimizing the number of times models need to be retrained.
Developing a government-directed framework for auditing the environmental impact of these models is also a crucial step. This would help ensure that companies are transparent about their environmental costs.
For another approach, see: Generative Ai Environmental Impact
Regulating for transparency of these models is also essential. By making it clear how much energy and water is being used, we can hold companies accountable for their environmental impact.
Encouraging researchers to publish data on their models' carbon footprint is another important step. This would help us better understand the environmental impact of these models and make more informed decisions.
Increasing the number of subject matter experts who understand both machine learning and climate science is also critical. By bringing together experts from these two fields, we can develop more effective solutions to the environmental challenges posed by generative models.
Worth a look: Impact of Generative Ai
Concerns and Risks
Generative AI has enormous potential for good and evil at scale, according to the United Nations Secretary-General António Guterres.
Concerns about generative AI have been raised by governments, businesses, and individuals, resulting in protests, legal actions, and calls to pause AI experiments. The "black box" nature of some generative AI models and unclear decision-making algorithms are major concerns for many executives.
Nearly half of chief data officers view a lack of transparency and difficulty in explaining the reasoning behind complex generative AI models as issues affecting their adoption. Algorithm bias remains pervasive in current models, with 86% of respondents and 96% of chief data officers agreeing.
Generative AI can reflect and amplify cultural bias present in the underlying data, leading to issues such as racial and gender bias. For example, a language model might assume doctors and judges are male, and secretaries or nurses are female, if those biases are common in the training data.
A number of methods for mitigating bias have been attempted, such as altering input prompts and reweighting training data. However, these methods may not be effective in eliminating bias entirely.
Risks of Generative AI
Concerns
Generative AI has enormous potential for good and evil at scale, according to the United Nations Secretary-General António Guterres. He warned that its malicious use could cause horrific levels of death and destruction, widespread trauma, and deep psychological damage.
Concerns about generative AI have been raised by governments, businesses, and individuals, resulting in protests, legal actions, and calls to pause AI experiments. Multiple governments have taken actions in response to these concerns.
One in three CISOs are uncomfortable with the "black box" nature of some generative AI models and its unclear decision-making algorithms. This lack of transparency is a major issue.
Legacy infrastructure is a significant barrier to using generative AI, with 90% of executives saying it affects business agility. Nearly 8 in 10 respondents are unsure of the actual benefits of generative AI to their operations.
Chief data officers are particularly hesitant about GenAI, with nearly half viewing a lack of transparency and the difficulty in explaining the reasoning behind complex generative AI models as major issues.
Algorithm bias remains pervasive in current models, with 86% of all respondents and 96% of chief data officers agreeing. This is a significant concern for the adoption of generative AI.
Generative AI has been used to create deepfakes, which are AI-generated media that take a person in an existing image or video and replace them with someone else's likeness. Deepfakes have been used in various forms of cybercrime, including phishing scams, fake news, and hoaxes.
If this caught your attention, see: How Multimodal Used in Generative Ai
Here are some examples of the types of cybercrime that have been committed using generative AI:
- Phishing scams
- Fake news and hoaxes
- Financial fraud
- Covered foreign election interference
The use of copyrighted content in training generative AI systems is also a concern, with several lawsuits ongoing. Proponents of fair use training argue that it is a transformative use, while critics argue that it infringes on copyright holders' rights.
Generative AI has also been used to create realistic fake content, including audio deepfakes, which have been used in social engineering attacks and phishing attacks.
Here's an interesting read: Generative Ai for Content Creation
Racial and Gender Bias
Racial and gender bias is a significant concern in generative AI models. They can reflect and amplify cultural biases present in the underlying data.
A language model might assume doctors and judges are male, and secretaries or nurses are female, if those biases are common in the training data. This can lead to inaccurate or unfair representations of certain groups.
Generative AI models can also create images that reflect racial biases if trained on a racially biased data set. For example, an image model prompted with the text "a photo of a CEO" might disproportionately generate images of white male CEOs.
Altering input prompts and reweighting training data are methods that have been attempted to mitigate bias in AI models.
Broaden your view: Generative Ai by Getty
Meity Drafts Ethics Code for Firms
The Indian government's Ministry of Electronics and Information Technology (MeitY) is working on a voluntary ethics code for AI firms, with a focus on measures during the training, deployment, and commercial sale of Large Language Models (LLM) and AI platforms.
The code will likely include principles for companies to identify and rectify instances of potential misuse of their LLM and AI platforms, as per a government official.
This move is part of the government's efforts to regulate the use of generative AI and ensure responsible innovation in the field.
The code will be released soon, and its exact details are yet to be announced.
It remains to be seen how the code will be enforced and what kind of impact it will have on the AI industry in India.
If this caught your attention, see: Can I Generate Code Using Generative Ai
Frequently Asked Questions
What is the next in generative AI?
The next step in generative AI is explainability, where AI decisions are transparent and understandable by humans. This shift prioritizes fairness and accuracy by reducing biases in AI systems.
Sources
- https://en.wikipedia.org/wiki/Generative_artificial_intelligence
- https://www.techrepublic.com/article/ciso-generative-ai-problems-ntt-data/
- https://theconversation.com/topics/generative-ai-133426
- https://charliebeckett.medium.com/what-we-have-learnt-about-generative-ai-and-journalism-and-how-to-use-it-7c8a9f5e86fd
- https://economictimes.indiatimes.com/topic/generative-ai
Featured Images: pexels.com