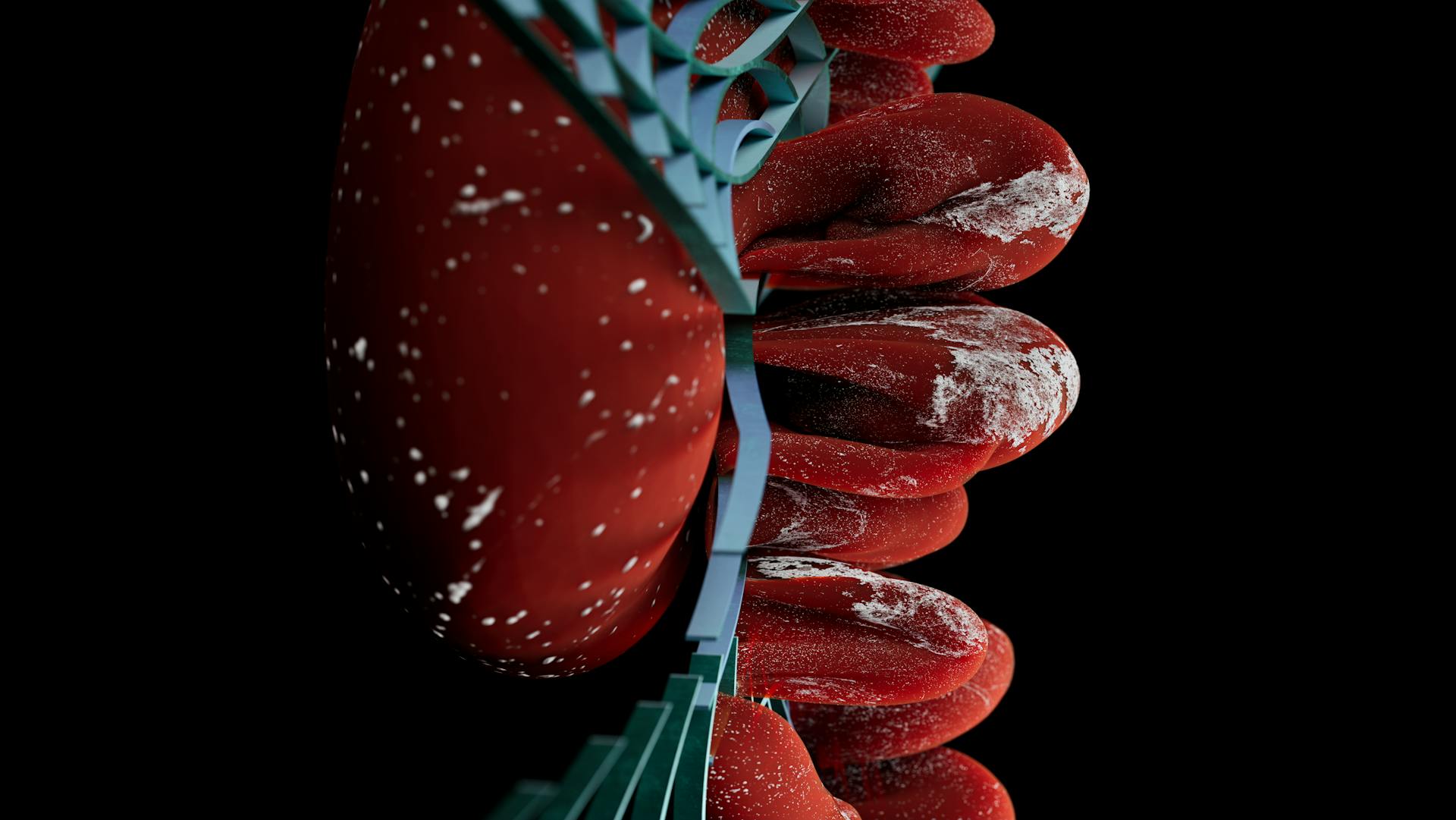
Generative AI fake news is a growing concern that can have serious consequences. According to research, 70% of online adults in the United States have experienced or witnessed fake news at some point.
The rise of generative AI has made it easier for fake news to spread. AI-generated content can be created in a matter of seconds, and it can be tailored to fit specific agendas or biases. This has led to an increase in disinformation campaigns, with some studies showing that up to 60% of online news consumption is actually fake news.
It's not just individuals who are affected by fake news - entire elections and social movements have been influenced by it. For example, in the 2016 US presidential election, fake news was spread through social media platforms, potentially swaying the outcome of the election.
Curious to learn more? Check out: Generative Ai Online
What is Generative AI Fake News?
Generative AI fake news is a type of misinformation that's created using artificial intelligence and machine learning algorithms. This can include deepfakes, which are digital forgeries that use AI to generate realistic images, videos, or audio recordings that appear to be authentic but are actually fake.
Misinformation can be spread both unintentionally and intentionally, and it's often referred to as the umbrella term that includes false or partially false information. The authors of this work chose to focus on misinformation as a broader category than disinformation, which implies an intention to deceive or mislead people.
Deepfakes can be created using open-source software or customized tools, making it easy to spread misinformation, propaganda, or to defame someone.
What Does Mean?
Generative AI is a technology that can autonomously produce content in any form, including language and meaning. This is a new area that was previously reserved for humans.
It's often impossible to tell if content originates from a human or a machine, which raises questions about trustworthiness. Media users are beginning to understand that something is broken in their relation to media.
Generative tools bypass traditional journalistic principles, such as relying on trusted sources. This means goodbye to the idea that there are authors behind every text or a creator behind every piece of visual content.
The connection between the creator and the content no longer exists, making it difficult to verify the source of information. This is a significant change that affects how we consume and trust information.
Discover more: Generative Ai for Content Creation
What Are They?
Generative AI is a technology that allows for the autonomous production of content in any form, making it difficult to tell if content originates from a human or a machine.
It's like trying to spot a fake photo - generative AI can create realistic images, videos, or audio recordings that appear authentic but are actually fake.
These manipulated media files are created by superimposing one person's face onto another's body or by altering the voice, facial expressions, and body movements of a person in a video.
Deepfakes are a form of digital forgery that use artificial intelligence and machine learning to generate these realistic images, videos, or audio recordings.
Authoritarian regimes may exploit generative AI to craft and spread messages that aim to bolster their legitimacy, discredit opponents, or manipulate public opinion about key issues related to the practice of democracy.
Gen AI has the potential to simplify the production and delivery of false content that is highly tailored for specific audiences, making it even more convincing.
Additional reading: Generative Ai Content
This technology can be used to create "precision cognitive attacks", which would use highly tailored information operations to target individuals or small groups.
Researchers in China are working on a gen AI-based system for precision cognitive attacks, which would use highly tailored information operations to target individuals or small groups.
This approach could be particularly useful for interfering in subnational elections, which are singled out for manipulation less frequently than national contests due to their greater number and the diversity of relevant campaign issues at the subnational level.
Intriguing read: Generative vs Cognitive Ai
Risks of ChatGPT and Open-Source Language Models
The risks of ChatGPT and open-source large language models are real and concerning. Generative AI tools like ChatGPT are now widely available, but this also means they can be used for negative purposes.
Proprietary models like those used by ChatGPT and Google's Gemini are owned by their companies, which raises concerns about transparency and the use of personal data for training purposes.
Consider reading: How Can Generative Ai Be Used in Cybersecurity
The lack of transparency in these models is a significant issue, as it makes it difficult to understand how they work and what data they use.
Other powerful open-source large language models are freely available, but they often lack integrated safeguards, making them more susceptible to misuse.
Research by Democracy Reporting International found that these open-source LLMs can rival the quality of products like ChatGPT and Gemini, but they are also more vulnerable to creating misinformation or hate speech.
The stakes are particularly high in 2024, with over fifty national elections taking place around the world, and generative AI technologies posing new risks to democracy.
For your interest: Open-source Generative Ai
Building Trustworthy Tools
GAI applications can be combined to automate the whole process of content production, distribution, and amplification.
Fully synthetic visual material can be produced from a text prompt, and websites can be programmed automatically.
The Learning Guide includes explainers, videos, and articles to help media professionals and experts evaluate media development activities and rethinking approaches to disinformation.
It offers practical solutions and expert advice, with a focus on the Global South and Eastern Europe.
Media professionals can use this guide to gain insights and develop effective strategies to tackle disinformation in the public arena.
DW Akademie partners and civil society actors can also benefit from the guide's expert advice and practical solutions.
For another approach, see: Generative Ai Solution
Effects and Implications
The spread of generative AI fake news can have serious consequences, including the erosion of trust in institutions and the manipulation of public opinion. This is evident in the way AI-generated content can be designed to mimic the style and tone of reputable sources, making it difficult for readers to distinguish fact from fiction.
The impact of generative AI fake news can be far-reaching, with potentially devastating effects on individuals and society as a whole. For instance, AI-generated fake news can be used to sway elections, influence public policy, and even incite violence.
In the absence of effective regulation and fact-checking, the proliferation of generative AI fake news can have a corrosive effect on democratic institutions, undermining the very foundations of a free and informed society.
For your interest: Claude Ai News
Effects of Concrete on Dis
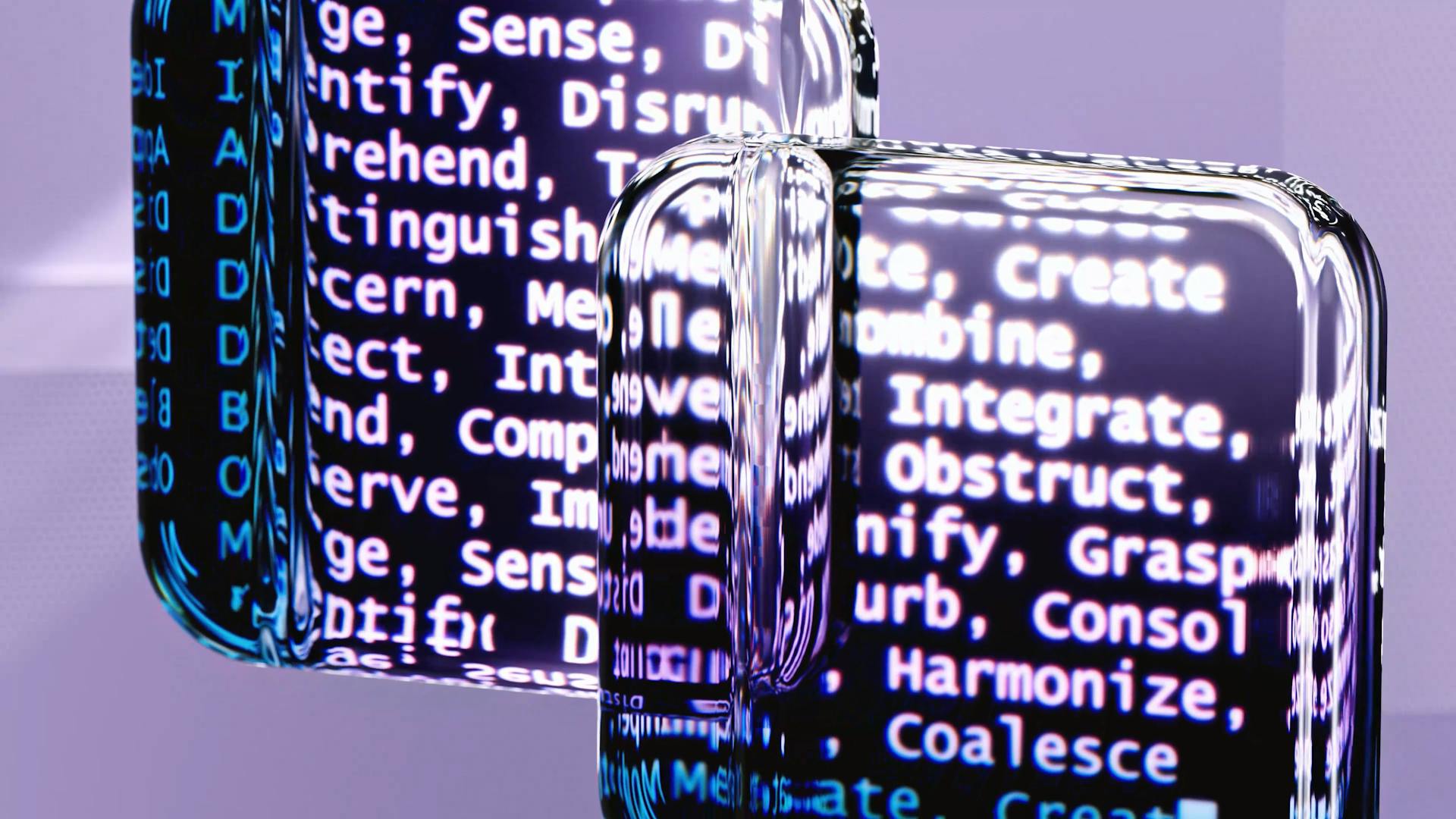
Concrete can cause significant damage to structures, including buildings and bridges, due to its high compressive strength and tendency to crack over time.
The weight and bulk of concrete can also lead to soil settlement and subsidence, causing foundations to shift and become unstable.
In extreme cases, concrete can even cause catastrophic failures, such as the collapse of a bridge or the cracking of a dam.
Concrete's high pH level can also accelerate the corrosion of steel rebar, leading to a weakened structure and increased risk of collapse.
The durability of concrete is highly dependent on its mix design, with factors such as cement type, aggregate size, and water content all playing a crucial role in its long-term performance.
Proper curing and finishing techniques are essential to prevent surface defects and ensure a strong, durable finish.
COVID-19
COVID-19 misinformation has spread quickly since the start of the pandemic, covering a variety of content, including prevention, treatment, vaccine, and politics.
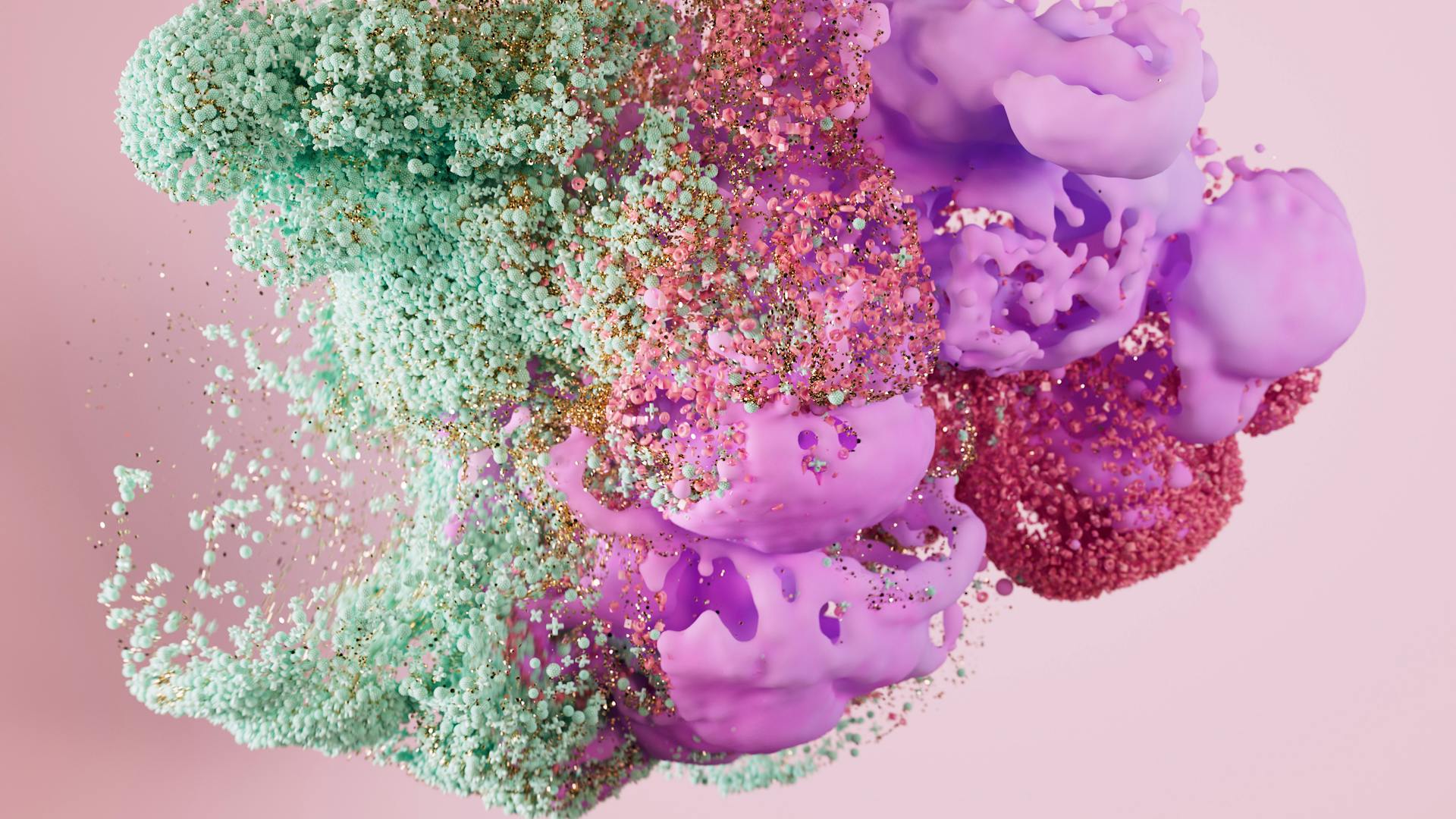
28% of Americans think that Bill Gates uses vaccines to implant microchips in people. This conspiracy idea is just one example of the many false claims circulating online.
The nature of the COVID-19 public health crisis, featuring high levels of polarization, constantly changing situations, and uncertainty, has made people more vulnerable to worry and conspiracy ideas.
Individuals have turned to unofficial sources for information, which is when misinformation campaigns effectively generate confusion.
Mortality and hospital admissions have resulted from the misinformation that drinking methanol or alcohol-based cleaning products can cure the virus. This is a tragic reminder of the dangers of false information.
Summary
In 2024, more than fifty national elections will take place globally, making it a critical year for democracy.
Authoritarian actors have been using generative AI to manipulate the information space and undermine democracy for a while now.
Faster and more expansive generative AI technologies are creating new risks, making it even more challenging for democracy to thrive.
These technologies have the potential to accelerate harmful narratives in a wide range of country contexts.
The stakes are high, and civil society organizations are facing a tough challenge in pushing back against authoritarian efforts.
Generative AI is being used by both authoritarians and civil society organizations, highlighting the complex and nuanced nature of this issue.
The report explores the ways in which generative AI is being used to tip the scales against democracy and how civil society organizations are using it to push back.
Broaden your view: How Multimodal Used in Generative Ai
Detection and Prevention
Detecting AI-generated fake news can be tricky, but there are some common-sense steps you can follow. Public figures like politicians or celebrities can be a giveaway if their statements are inconsistent with what has already been publicly reported.
If you're unsure about an audio clip, compare it with previously authenticated video or audio clips that feature the same person's voice. Are there any inconsistencies in the sound of their voice or their speech mannerisms?
You might enjoy: Generative Ai Audio
Awkward silences or robotic speech patterns can also indicate that someone is using AI-powered voice cloning technology. Any unusually verbose manner of speaking could be a sign of voice cloning combined with a large language model.
Here are 4 steps to help you recognize if audio has been cloned or faked using AI:
- Check if the statement is consistent with what has already been publicly reported or shared about the public figure's views and behavior.
- Compare the audio clip with previously authenticated video or audio clips that feature the same person's voice.
- Look for awkward silences or unusual pauses while speaking.
- Be wary of robotic speech patterns or an unusually verbose manner of speaking.
Evaluating Existing Detection Models
Evaluating Existing Detection Models is a crucial step in the fight against misinformation. We need to assess how well current detection models perform on AI-generated misinformation.
Pre-existing misinformation detection models have been evaluated on AI-generated misinformation, with some surprising results. Figure 1 illustrates the outcomes of these evaluations.
Fact checkers are employing generative AI to expedite the verification of information, which has led to the development of fact-checking chatbots. These chatbots can quickly verify the veracity of content in response to user requests.
The Perevirka chatbot, developed by Gwara Media in Kharkiv, Ukraine, specializes in debunking Kremlin messaging online. Users send in a text, photo, video, or link, and receive a response immediately if the item has already been investigated.
For more insights, see: Anomaly Detection Using Generative Ai
Traditional AI capabilities are being used to understand and interpret fact-checking requests, but the addition of generative AI is accelerating this trend. This requires significant human effort and review, but hastens the collection of basic information surrounding the questionable content or underlying narrative.
Cofacts, a Taiwanese civic tech community, is experimenting with the use of generative AI for their chatbot-based fact-checking system. The system operates primarily on closed-door messaging apps and uses generative AI to provide more substantive responses to user submissions.
Evaluating Existing Assessment Guidelines
Evaluating existing assessment guidelines is crucial in detecting and preventing misinformation. We can start by looking at the guidelines in the areas of journalism work practice, misinformation empirical and review studies, and public education on media literacy.
Sources are important, and citing them is a good practice. Sources of information are cited, such as "The University of Vienna has sent a memo." Establishing credibility of sources is also essential, as seen in "This investigation is made by the World Health Organization."
Recommended read: What Is a Best Practice When Using Generative Ai
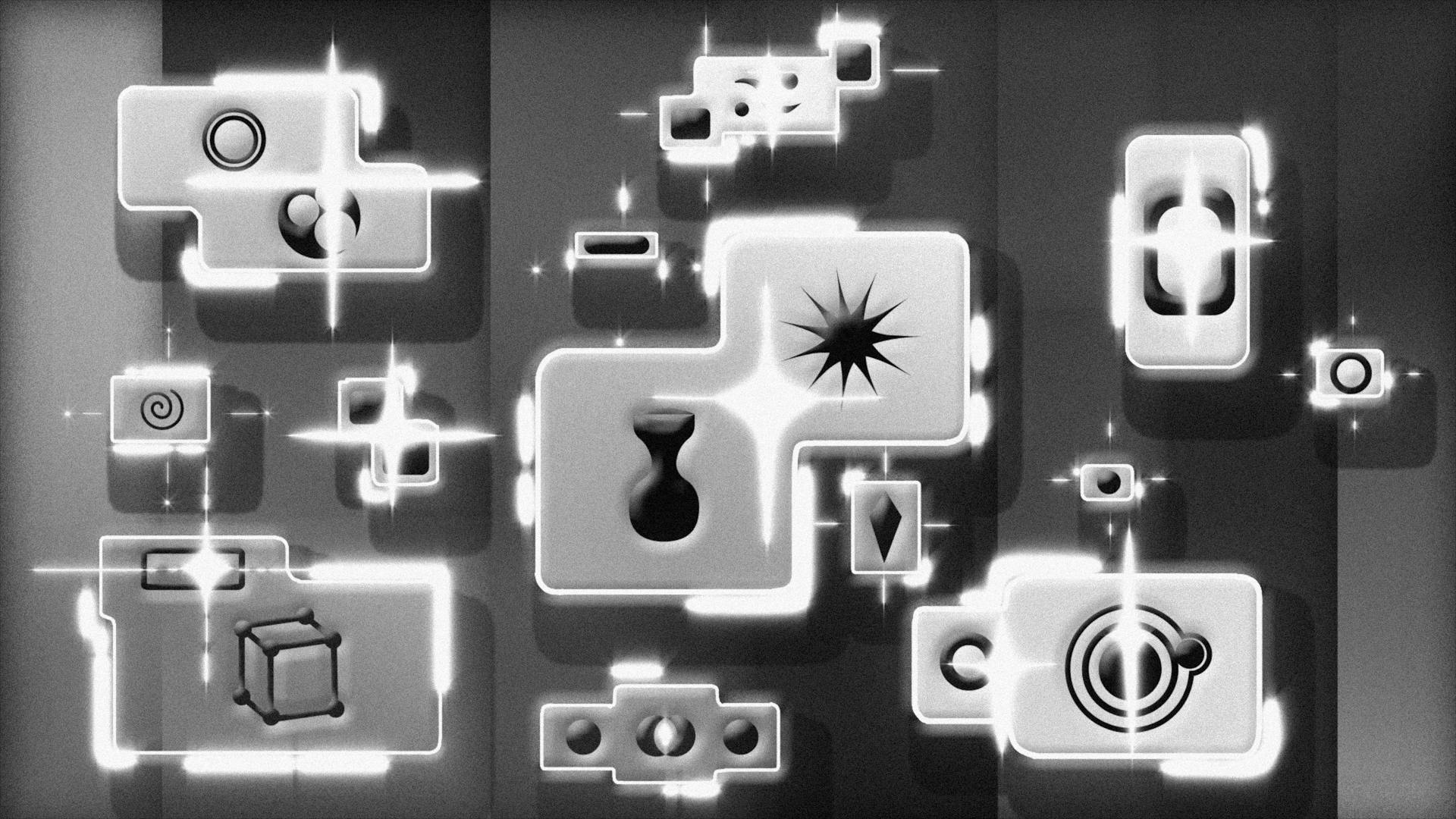
To evaluate evidence, we can look at the types of evidence presented. Statistical evidence is used, such as survey results or census data. Testimonial evidence is also used, like expert suggestions or research studies. Documented evidence, like videos or photos, is another type of evidence. Anecdotal evidence, which includes personal observations or stories, is also used. Analogical evidence, which uses comparisons to demonstrate similarities or differences, is another type of evidence.
Evidence vetting is also important. Mentioning the effort or process to vet evidence, regardless of the results, is a good practice. For example, "the government has not responded or confirmed this report."
In addition to evaluating evidence, we should also consider alternative explanations or understandings. Assertive confidence, such as using imperative expressions like "should" and "must", can be used to demonstrate certainty. Acknowledging alternatives or uncertainties is also important, such as mentioning other ways to achieve a goal or other explanations of a phenomenon.
Here's a summary of the types of evidence and how to evaluate them:
By following these guidelines and considering multiple types of evidence, we can improve our ability to detect and prevent misinformation.
Tools and Techniques
Generative AI tools like Midjourney and Dall-e can generate high-quality images from text prompts, making it easier to create realistic-looking content.
Midjourney uses a combination of neural networks to create images, while Dall-e is trained on a large dataset of images and can generate a wide range of images, from realistic to abstract.
These tools can produce fully synthetic visual material from a text prompt, and websites can be programmed automatically to distribute and amplify this content.
Stable Diffusion is another GAN that can generate realistic images and videos, with the key feature of stabilizing the transition between two different states of the image.
If this caught your attention, see: Getty Generative Ai
Abstract Documents into Narrative Prompts
Abstract documents can be transformed into narrative prompts using a technique called "inference mapping." This involves identifying key concepts and relationships within the document and rephrasing them in a more story-like format.
By rephrasing abstract concepts, you can make them more engaging and accessible to a wider audience. For example, a technical report on a new medical breakthrough can be reworked into a narrative prompt that asks, "What if a new treatment could cure a previously incurable disease?"
On a similar theme: New Generative Ai
Inference mapping requires an understanding of the underlying structure and relationships within the document. This can be achieved by analyzing the document's key terms, concepts, and relationships. For instance, a document on a new scientific discovery might include key terms like "photosynthesis" and "plant growth", which can be used to create a narrative prompt like "How does the process of photosynthesis impact plant growth in different environments?"
By breaking down complex information into smaller, more manageable chunks, you can create narrative prompts that are both engaging and informative.
Additional reading: Google Generative Ai Key
Tools Used
Midjourney is a GAN AI that generates high-quality images of objects, people, and landscapes.
Dall-e from OpenAI is a GAN that can generate unique images from textual inputs, trained on a large dataset of images.
Stable Diffusion is a GAN that generates realistic images and videos, with the key feature of stabilizing the transition between two different states of the image.
AI tools like Midjourney, Dall-e, and Stable Diffusion are being used to create deepfakes.
Worth a look: Stable Diffusion Generative Ai
Deep Fakes Examples
Deep Fakes Examples are being created by generative AI and going viral in social media.
These images are so realistic they've fooled millions of people worldwide.
In May, an AI-generated deepfake image of a bomb at the Pentagon exploding went viral on Twitter.
This caused US markets to plummet, with the S&P 500 stock index falling 30 points in minutes.
As a result, $500 billion was wiped off the market cap.
Combating Fake News
Google has launched a new tool called 'About This Image' to help people spot fake AI images on the internet, providing additional context alongside pictures, including details of when the image first appeared on Google and any related news stories.
Educating the public about the potential harm of AI-generated deepfakes may be crucial in preventing their spread, and being vigilant when consuming media, verifying its source and contextual information, and using critical thinking when interpreting its contents is essential.
The emergence of gen AI as a tool for information manipulation by authoritarians may not fundamentally change the nature of democratic responses to such efforts, but building awareness about the capabilities of gen AI tools can help the public at-large prepare for what they might see around elections or at other critical moments of public discourse.
For your interest: When Was Generative Ai Open Source
How to Combat
To combat fake news, we need to be vigilant when consuming media and verify its source and contextual information.
Google has launched a new tool called 'About This Image' to help people spot fake AI images on the internet.
Educating the public about the potential harm of AI-generated deepfakes is crucial in preventing their spread.
We should be critical of the information we consume, using critical thinking when interpreting its contents.
Private companies like Microsoft are collaborating with news organizations to integrate generative AI into journalism, aiming to create financially sustainable newsrooms.
A digital watermarking system can verify the authenticity of media content, making it harder for fake news to spread.
Building awareness about the capabilities of generative AI tools can help the public at-large prepare for what they might see around elections or critical moments of public discourse.
However, overemphasizing the power and ubiquity of generative AI could strengthen the liar's dividend, making people distrust even authentic media.
A different take: Generative Artificial Intelligence News
DeSantis Campaign Tied to Trump
The DeSantis campaign has been tied to a deepfake video that attacked rival Donald Trump. The video, shared on June 5th, used AI-generated deepfakes to portray Trump as a close collaborator of Anthony Fauci.
The intention behind the attack ad was to strengthen DeSantis' support base by exploiting right-wing opposition to Fauci. This is a concerning example of how AI-generated content can be used to manipulate public opinion.
Justin T. Brown, an artist who created AI-generated images to highlight the dangers of AI, was banned from the Midjourney subreddit after sharing his work. Brown questioned the effectiveness of regulating content, highlighting the challenges of balancing free expression with accountability.
The DeSantis campaign's use of deepfakes raises questions about the role of AI in politics and the need for greater transparency and accountability in the use of AI-generated content.
A fresh viewpoint: Ai Generative Fill for Video
Methods and Results
We examined existing algorithms that detect misinformation and found that all currently published models were developed for the AAAI 2021 shared task challenge – “COVID-19 Fake News Detection in English” using the CONSTRAINT dataset. The winner of this challenge was the COVID-Twitter-BERT (CT-BERT) model, which achieved a weighted F1-score of 0.987 on the final blinded test set.
Curious to learn more? Check out: Generative Ai Challenges
The CT-BERT model was a transformer-based model pretrained on COVID-19-related Twitter documents collected from January to April 2020. We evaluated its performance on AI-generated misinformation and found that it maintained high predictability but had a significant performance drop compared to human-created misinformation.
A χ test showed that there was a significant difference between AI-misinfo and Human-misinfo data in detecting performance (χ=22.2, p<0.00001). Here's a summary of the performance comparison:
The error analysis of the 27 AI-misinfo false negative cases revealed that language complexity was a major issue, with many error cases featuring complex sentence structures or rare semantic patterns.
Explore further: Generative Ai Use Cases in Financial Services
Limitations and Future Work
The study on generative AI fake news has its limitations, and researchers are already thinking about how to improve it. One limitation is that the findings might not be directly applicable to other contexts, but the researchers believe that general patterns of AI-generated misinformation can still be applied to similar topics.
The study used a specific type of AI model, GPT-3, to create fake news, and the results might not be the same if other models or themes were used. The researchers invite future work to study content created by other models and themes.
To make AI-generated misinformation comparable to human-generated misinformation, the researchers created "narrative prompts" that capture the core elements of a narrative. However, they acknowledge that this approach might leave out some nuanced attributes.
A fresh viewpoint: Chatgpt Openai Generative Ai Chatbot Can Be Used for
The Future of
Deepfakes will soon be more realistic, not just limited to images but also videos. The technology is advancing rapidly.
Voice cloning technology has already made significant progress and will only continue to improve in the coming years. This raises serious concerns about the potential misuse of deepfake technology.
The lines between reality and fake will become increasingly blurred as the technology advances. This makes it more critical than ever to develop measures to identify and combat the spread of deepfakes.
A different take: Roundhill Generative Ai & Technology Etf
8.3 Limitations
Our research has some limitations that are worth noting. We assume that existing datasets without note of AI-generated or synthetic text are human creations, which may not always be true. This assumption can lead to inaccuracies in our findings.
We also acknowledge that our approach to creating narrative prompts may not capture all nuanced attributes of misinformation. Our prompts are based on Narrative Theory, but they may not be comprehensive enough to cover all possible scenarios.
To make AI-misinfo and Human-misinfo comparable, we created narrative prompts to capture the core elements in a narrative. However, this approach may leave out some details, and future studies can explore different strategies to generate and study AI-generated misinformation.
We evaluated pre-existing solutions that were designed for human-created misinformation, which means our results are more exploratory in nature. This is not a comprehensive evaluation of risk and applicability, but rather a starting point for future research.
Our findings can be reasonably generalized to similar topics like crisis communication and public health, but not all findings can be directly applied to other contexts. Future work can study content created by other LLMs and/or for other themes to further understand the scope of AI-misinfo.
Related reading: How Generative Ai Can Augment Human Creativity
Frequently Asked Questions
What is the problem with generative AI?
Generative AI can provide inaccurate information due to its predictive nature, which may lead to 'hallucinated' answers. This can result in unreliable results, making it essential to verify information generated by AI tools
How accurate is generative AI?
Generative AI is often inaccurate, with a study finding that it provided mostly false or incomplete answers to legal questions. Research suggests that its accuracy can be improved, but more work is needed to understand its limitations.
Sources
- https://akademie.dw.com/en/generative-ai-is-the-ultimate-disinformation-amplifier/a-68593890
- https://dl.acm.org/doi/fullHtml/10.1145/3544548.3581318
- https://www.ned.org/manufacturing-deceit-how-generative-ai-supercharges-information-manipulation/
- https://www.newscientist.com/article/2445475-how-to-avoid-being-fooled-by-ai-generated-misinformation/
- https://thumos.global/generative-ai-and-deepfakes/
Featured Images: pexels.com