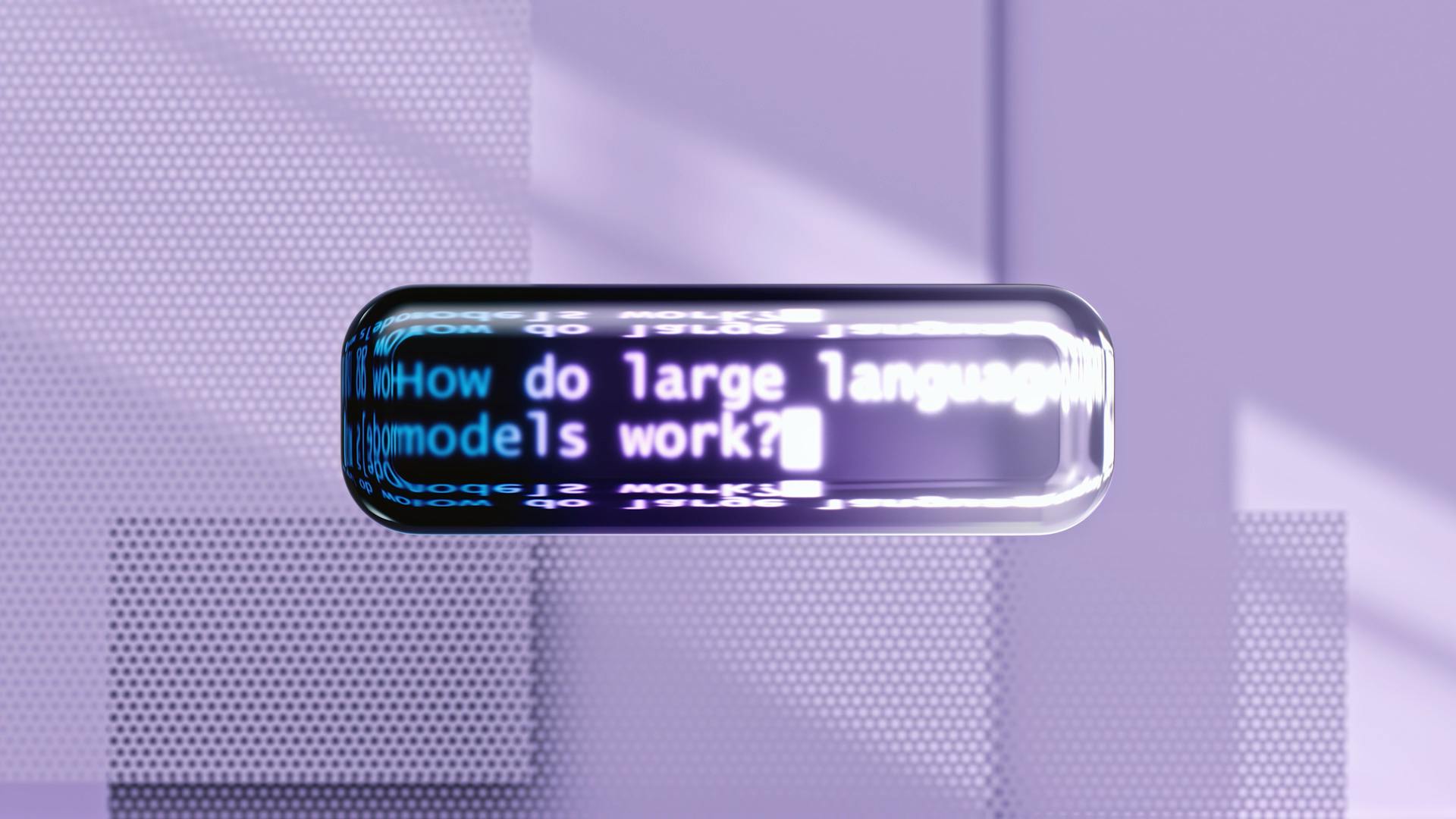
Generative AI is designed to create new content, such as images, music, or text, while Predictive AI focuses on forecasting future events or outcomes.
Generative AI is particularly useful for tasks like generating realistic images or music, as seen in the examples of AI-generated portraits and music compositions.
Predictive AI, on the other hand, is more concerned with making predictions about future events, like stock prices or weather forecasts.
The key difference between the two lies in their objectives, with Generative AI aiming to create new content and Predictive AI aiming to forecast future outcomes.
Explore further: New Generative Ai
What is Generative AI
Generative AI is a type of artificial intelligence that can create new, original content such as images, music, and text. This is in contrast to predictive AI, which is focused on making predictions or forecasting future events.
Generative AI uses algorithms and machine learning techniques to generate new data that is similar in style and structure to existing data. For example, a generative AI model can create new images of faces that are similar to those in a training dataset.
For your interest: Getty Images Nvidia Generative Ai Istock
Generative AI has many practical applications, including content creation, art, and even music composition. In fact, some artists are already using generative AI to create new and innovative works.
A key characteristic of generative AI is its ability to learn from data and generate new content based on that data. For instance, a generative AI model can learn from a dataset of images of dogs and generate new images of dogs that are not in the original dataset.
You might like: Generative Ai Content
Key Features
Generative AI creates new, original content, whereas predictive AI uses existing data to forecast future outcomes.
One key feature of generative AI is its ability to learn from patterns and create new data, such as generating new images or writing new text.
Predictive AI, on the other hand, relies on statistical models to make predictions based on historical data, like forecasting sales or stock prices.
Generative AI can also be used for creative tasks, like composing music or creating art, whereas predictive AI is more focused on analytical tasks.
For your interest: Google Announces New Generative Ai Search Capabilities for Doctors
The output of generative AI can be entirely new and unique, whereas predictive AI's output is based on existing data and trends.
Generative AI can learn from large datasets and create new content that is tailored to specific contexts or tasks, whereas predictive AI is typically limited to the data it was trained on.
On a similar theme: Why Is Controlling the Output of Generative Ai Systems Important
Benefits and Advantages
Generative AI offers numerous benefits and advantages, especially in innovation and product development. It can rapidly prototype and iterate ideas, allowing businesses to get new products out faster and ahead of the competition.
Predictive AI, on the other hand, focuses on refining existing products and delivering excellent after-sales service. It enhances user experiences and helps corporations make informed decisions.
Generative AI has the power to create novel content, from text and images to complex engineering designs and software code. It can synthesize entirely new content, combine disparate concepts, and adapt to specific style requirements or constraints.
Readers also liked: Generative Ai Content Creation
Here are some key differences between generative and predictive AI:
State-of-the-art generative AI models, like ChatGPT and Claude 3.5 Sonnet, demonstrate remarkable capabilities in natural language processing and generation. These advancements have led to significant breakthroughs in model architecture, training techniques, and application domains.
Operational Efficiency
Generative AI automates complex data analysis processes, significantly reducing the time and effort required for manual analysis. This automation streamlines operations, enhances productivity, and allows employees to focus on high-value tasks that require human expertise.
Predictive AI is not mentioned in this section, but we know from Example 5 that it automates complex data analysis processes, which is similar to what generative AI does. In fact, generative AI can even synthesize entirely new content that adheres to learned patterns and rules (Example 2).
By automating repetitive tasks, generative AI optimizes business workflows, leading to greater efficiency and productivity (Example 3). This allows employees to focus on strategic and creative work, increasing overall productivity.
Here's an interesting read: Which Is an Example Limitation of Generative Ai Interfaces
Some examples of how generative AI achieves operational efficiency include:
- Automating complex data analysis processes
- Generating new ideas, designs, and solutions
- Creating novel content across various domains
These capabilities are not only limited to text and images but also extend to complex engineering designs and software code (Example 2). The development of larger, more sophisticated transformer-based models has dramatically improved the quality, coherence, and contextual understanding of generated content (Example 2).
Suggestion: Generative Ai with Python and Tensorflow 2 Pdf
Advanced Risk Management
Advanced Risk Management is a game-changer for businesses. Predictive AI can identify potential threats and vulnerabilities across various domains, such as financial risks, cybersecurity threats, and supply chain disruptions.
This proactive approach allows businesses to implement preventive measures and mitigate risks effectively. With predictive AI, you can stay one step ahead of potential problems and avoid costly mistakes.
By leveraging predictive AI, businesses can reduce the likelihood of financial losses due to risks like market fluctuations and unexpected expenses. This can lead to increased stability and growth.
Predictive AI can also help you identify and address cybersecurity threats before they cause harm. This can save you from costly data breaches and reputational damage.
Related reading: Generative Ai Cybersecurity
Challenges and Limitations
A staggering 80% of generative AI vs predictive AI initiatives stall at the pilot stage. This highlights the significant challenges that come with implementing these technologies.
Generative AI can produce biased or inaccurate content when the training data is biased, low-quality, or incomplete. It can also generate errors because it doesn’t have common sense. Besides, it can’t perform truly creative tasks and come up with totally novel ideas. Also, this technology can be misused to create deepfakes or spread misinformation.
Predictive AI accuracy also depends on the quality of the training data, and inaccurate, incomplete, or biased data can result in flawed and misleading insights. This is a common problem that many businesses face when trying to implement predictive AI effectively.
Content generated by artificial intelligence can sometimes be unpredictable, and the results can be either poor quality or completely wrong. This unpredictability makes it difficult to rely solely on AI for critical tasks without human oversight.
Discover more: Generative Ai Photoshop Increase Quality
Comparison and Analysis
Generative AI and predictive AI are two distinct technologies with different core functionalities. Generative AI generates new original content like images, text, and music through complex algorithms and deep learning models, while predictive AI forecasts future events based on historical data with statistical algorithms and ML models.
Generative AI excels in creative arts, product design, and data augmentation, producing original content, enhancing creative processes, and being versatile in various fields. Predictive AI, on the other hand, shines in scenarios demanding accurate forecasts, improving decision-making, and streamlining operations.
Here's a comparison of the two technologies in a table format:
This comparison highlights the unique strengths and applications of each technology, enabling businesses to choose the right tool for their specific needs.
Comparative Analysis
Generative AI and Predictive AI are two distinct technologies with different core functionalities. Generative AI generates new original content like images, text, and music through complex algorithms and deep learning models such as GANs and VAEs.
Their applications sometimes overlap, but they serve different purposes. Generative AI is useful in creative arts, product design, and data augmentation, while Predictive AI is applied in healthcare, finance, and retail.
One of the key differences between the two is their approach to data. Generative AI relies on unlabeled data, often large datasets, whereas Predictive AI uses labeled historical data.
Here's a comparison of their capabilities:
In terms of core algorithms, Generative AI uses GANs, VAEs, and Transformers, while Predictive AI relies on Random Forests, SVMs, and Neural Networks. This difference in approach affects their computational requirements, with Generative AI generally requiring more power.
Synergies and Complementarities
The integration of generative and predictive AI creates powerful hybrid systems that leverage the strengths of both approaches. Generative AI's ability to create novel solutions complements predictive AI's capacity for accurate forecasting and pattern recognition.
In advanced product design, generative AI can create multiple design iterations based on specified parameters, while predictive AI evaluates these designs, forecasting their performance, manufacturability, and market potential. This combination accelerates the design process and improves the likelihood of developing successful products.
A unique perspective: Create with Confidence Using Generative Ai
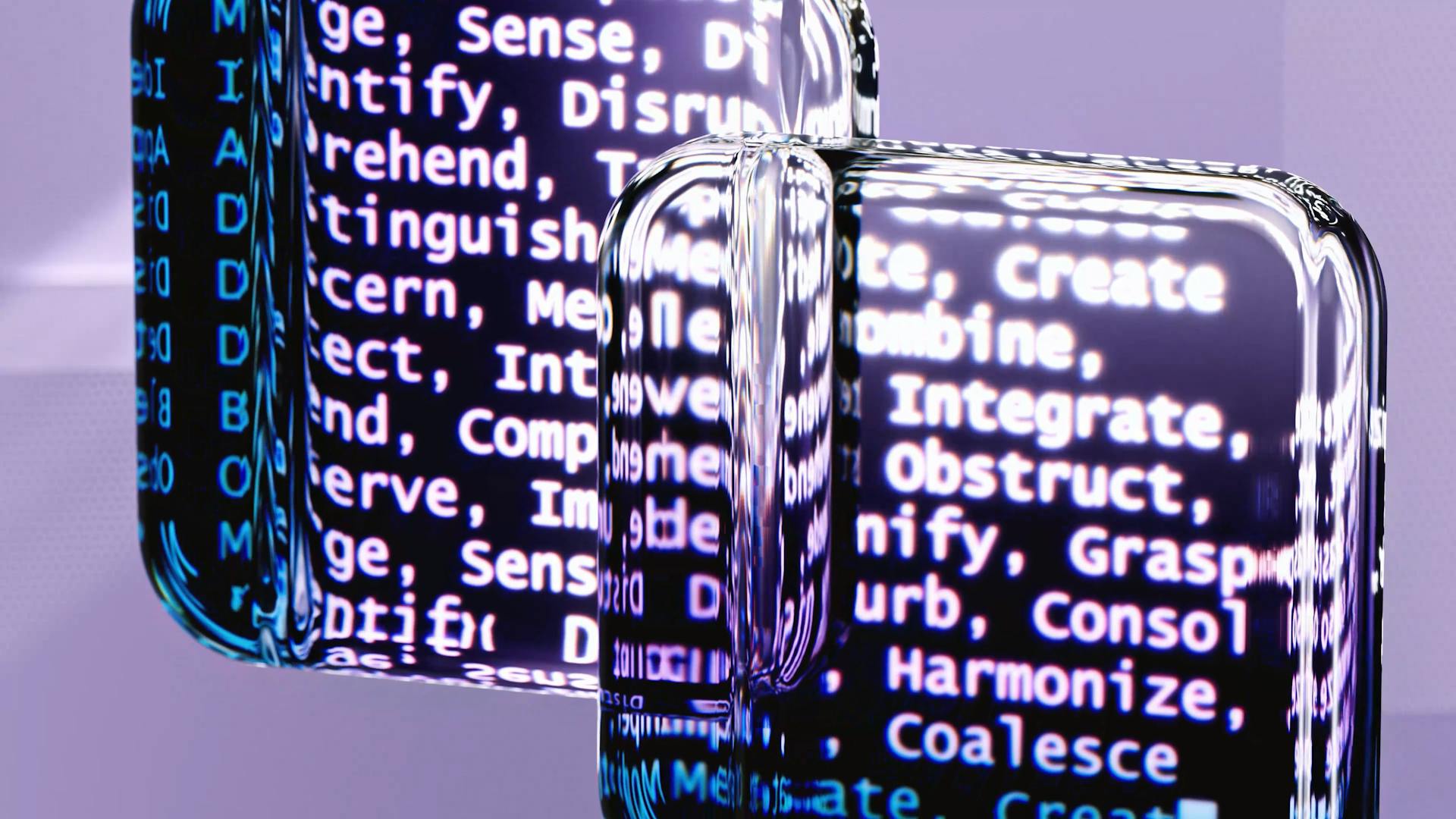
Retail companies can use Predictive AI to anticipate shopping trends and Generative AI to design targeted marketing campaigns. This helps businesses stay ahead of the competition and improve customer satisfaction.
Generative AI can propose new molecular structures or material compositions, while predictive AI forecasts their properties and performance characteristics. This synergy accelerates the discovery of novel materials with desired properties.
The combination of generative and predictive AI also enables the development of more efficient, sustainable, and resilient urban environments. Generative AI can create multiple city layout or infrastructure designs, while predictive AI models traffic flow, energy consumption, and environmental impact.
Here are some examples of hybrid AI systems that leverage both generative and predictive capabilities:
- Advanced product design: Generative AI creates design iterations, while predictive AI evaluates and forecasts performance.
- Aerospace engineering: Generative AI generates innovative aircraft component designs, while predictive AI forecasts aerodynamic performance and structural integrity.
- Autonomous vehicles: Generative AI creates diverse simulation scenarios, while predictive AI forecasts vehicle behavior and potential safety risks.
- Smart manufacturing: Generative AI proposes production line configurations or process optimizations, while predictive AI assesses and forecasts impact on efficiency, quality, and resource utilization.
- Materials science: Generative AI proposes new molecular structures, while predictive AI forecasts properties and performance characteristics.
- Urban planning and civil engineering: Generative AI creates city layout or infrastructure designs, while predictive AI models traffic flow, energy consumption, and environmental impact.
The potential for innovation at this intersection is vast, and as these hybrid systems evolve, we can anticipate breakthroughs in complex problem-solving. This could lead to more adaptive and intelligent engineering systems capable of tackling multifaceted challenges.
Additional reading: How Are Modern Generative Ai Systems Improving User Interaction
Applications and Use Cases
Generative AI applications are diverse and can be implemented in various industries. They excel in content creation and have multiple use cases, including customer service, gaming, healthcare, marketing and advertising, and software development.
In customer service, gen AI-powered chatbots and virtual agents can offer real-time support and provide personalized responses. This can help organizations improve customer satisfaction and reduce response times.
In gaming, gen AI models can assist with creating real-world environments, lifelike characters, and dynamic animations. This can enhance the gaming experience and make games more engaging.
Predictive AI, on the other hand, is mainly used in finance, retail, e-commerce, and manufacturing. It can be used for financial forecasting, fraud detection, inventory management, personalized recommendations, and supply chain management.
Here are some specific examples of predictive AI applications:
- Financial forecasting: Predictive AI models can forecast market trends, stock prices, and other economic factors.
- Fraud detection: Predictive AI can spot suspicious transactions in real time that signify fraudulent activities.
- Inventory management: Predictive AI can help companies plan and control inventory levels by projecting sales and demand.
- Personalized recommendations: Predictive AI models can analyze patterns in customer behavior data for more tailored suggestions.
- Supply chain management: Predictive AI can aid in the optimization of logistics and operations, production plans, resource allocation, and workload scheduling.
Data Synthesis
Data Synthesis is a game-changer for machine learning models, especially when actual data is scarce or contains privacy concerns.
Generative AI is incredibly adept at synthetic data generation that closely resembles real-world datasets, making it a vital tool for training models.
This synthetic data can be used to augment existing datasets, helping to improve model accuracy and robustness.
In scenarios where actual data is expensive or hard to obtain, generative AI can step in and provide a cost-effective solution.
Here's an interesting read: Synthetic Data Generation Using Generative Ai
Use Cases
Generative AI and predictive AI have distinct use cases that can benefit businesses in various ways. Generative AI excels in content creation and has applications in customer service, gaming, healthcare, marketing and advertising, and software development.
In customer service, generative AI-powered chatbots and virtual agents can offer real-time support and provide personalized responses to customers.
Gaming is another area where generative AI models can create realistic environments, lifelike characters, and dynamic animations.
Healthcare is also a key industry for generative AI, as it can create synthetic data to train and test medical imaging systems, and even propose new molecules for drug discovery.
Marketing and advertising can also benefit from generative AI, which can design engaging visuals and craft compelling ad copy tailored to each target audience.
Software development can speed up the process of writing new code and automate debugging and testing phases with code generation tools.
Predictive AI, on the other hand, is mainly used in finance, retail, e-commerce, and manufacturing. It can help financial institutions forecast market trends and stock prices, spot suspicious transactions for fraud detection, and project sales and demand for inventory management.
See what others are reading: Generative Ai for Customer Support
In finance, predictive AI can also aid in personalized recommendations, supply chain management, and forecasting. It can help businesses tailor their marketing efforts and product recommendations to individual customers, increasing customer satisfaction and loyalty.
Here are some key use cases for predictive AI:
- Financial forecasting: Predictive AI models forecast market trends, stock prices, and other economic factors.
- Fraud detection: Predictive AI spots suspicious transactions in real-time.
- Inventory management: Predictive AI projects sales and demand to help companies plan and control inventory levels.
- Personalized recommendations: Predictive AI analyzes customer behavior data for tailored suggestions.
- Supply chain management: Predictive AI optimizes logistics and operations, production plans, resource allocation, and workload scheduling.
Career Opportunities
Career Opportunities abound in the realm of AI. Both generative AI and predictive AI offer exciting career paths for tech-savvy individuals.
Generative AI engineers design, develop, and implement generative models for real-world applications like chatbots and content generation. This requires learning machine learning frameworks like TensorFlow, PyTorch, and Keras.
Machine learning engineer is another career option in generative AI. To pursue this path, you'll need to learn neural networks, deep learning, and specific models like GANs, VAEs, and transformers.
A career in predictive AI can lead to becoming a data scientist who builds predictive models and figures out future trends and patterns. This requires a strong foundation in statistics, mathematics, and probability.
Data scientists use machine learning techniques like regression, classification, clustering, and time series analysis to analyze data. They also need to be proficient in programming languages such as Python, R, and SQL.
Other career options in predictive AI include ML engineer, business intelligence analyst, and predictive modeling.
Discover more: Gen Ai vs Ml
Technical Aspects
Generative AI models rely on various architectures, including diffusion models, GANs, transformer models, and VAEs. These architectures enable the creation of novel content.
Diffusion models work by adding noise to training data and then training the algorithm to iteratively diffuse the noise to reveal a desired output. This process allows for the creation of new content that is similar to the training data.
GANs consist of two neural networks: a generator that produces new content and a discriminator that evaluates the accuracy and quality of the generated content. This adversarial process encourages the model to generate increasingly high-quality outputs.
Transformer models use attention mechanisms to determine what's most important about data within a sequence, making them well-suited for tasks involving sequential data.
Here are some common architectures used in generative and predictive AI:
The choice of architecture depends on the specific task and desired properties of the generated output.
Algorithms and Architectures
Generative AI models rely on various architectures to create novel content. These architectures include Generative Adversarial Networks (GANs), Variational Autoencoders (VAEs), and Transformers.
GANs consist of two competing neural networks: a Generator and a Discriminator. The Generator learns to map from a simple noise distribution to the complex data distribution of the training set.
VAEs employ an Encoder-Decoder structure, where the Encoder compresses input data into a lower-dimensional latent space, and the Decoder reconstructs data from this latent representation.
Transformers, originally designed for natural language processing, have become a cornerstone of modern generative AI. They utilize self-attention mechanisms to handle sequential data effectively.
The choice of architecture depends on the specific task and desired properties of the generated output. For instance, GANs are often preferred for tasks requiring high-fidelity output, such as image generation.
Here are some of the key architectures used in generative AI:
- Diffusion models work by first adding noise to the training data until it's random and unrecognizable, and then training the algorithm to iteratively diffuse the noise to reveal a desired output.
- Generative Adversarial Networks (GANs) consist of two neural networks: a generator that produces new content and a discriminator that evaluates the accuracy and quality of the generated content.
- Transformer models use the concept of attention to determine what's most important about data within a sequence.
- Variational autoencoders (VAEs) are generative models that learn compressed representations of their training data and create variations of those learned representations to generate new sample data.
These architectures have different strengths and weaknesses, and the choice of which one to use depends on the specific task and desired properties of the generated output.
Computational Resource Requirements
Deep learning-based generative AI models require a lot of computational power and resources.
These models can be laborious and costly to prepare, requiring access to advanced hardware like GPUs or TPUs.
Significant amounts of energy are also needed to power these models.
Optimizing AI models for efficiency can help manage these resource requirements.
Exploring cloud-based solutions can also be a viable option for smaller businesses or those with budget constraints.
Explainability
Explainability is a significant challenge in AI development. Most generative AI models lack explainability, making it difficult to understand their decision-making processes.
Generative AI models often rely on complex algorithms and neural networks, which can be hard to interpret. This lack of transparency can make it challenging to trust their results.
Predictive AI models, on the other hand, are more explainable because they're grounded on numbers and statistics. However, even predictive AI estimates still require human judgment to interpret correctly.
An incorrect interpretation of AI results can lead to a wrong course of action. This highlights the importance of understanding the limitations of AI models and the need for human oversight.
On a similar theme: Generative Ai Human Creativity and Art Google Scholar
Frequently Asked Questions
Is ChatGPT generative AI or predictive AI?
ChatGPT is a generative AI tool, not predictive AI, as it creates original text responses rather than predicting or completing existing text. This allows ChatGPT to provide unique and personalized answers to user queries.
What is the difference between generative AI and AI?
Generative AI creates original, realistic outputs, whereas traditional AI models analyze and interpret data to make predictions or classifications. This key difference enables generative AI to produce entirely new and unique content.
Sources
- https://relevant.software/blog/generative-ai-vs-predictive-ai/
- https://ivyproschool.com/blog/generative-ai-vs-predictive-ai/
- https://www.ibm.com/blog/generative-ai-vs-predictive-ai-whats-the-difference/
- https://www.coveo.com/blog/generative-vs-predictive-ai/
- https://www.wevolver.com/article/generative-ai-vs-predictive-ai-unveiling-the-titans-of-artificial-intelligence
Featured Images: pexels.com