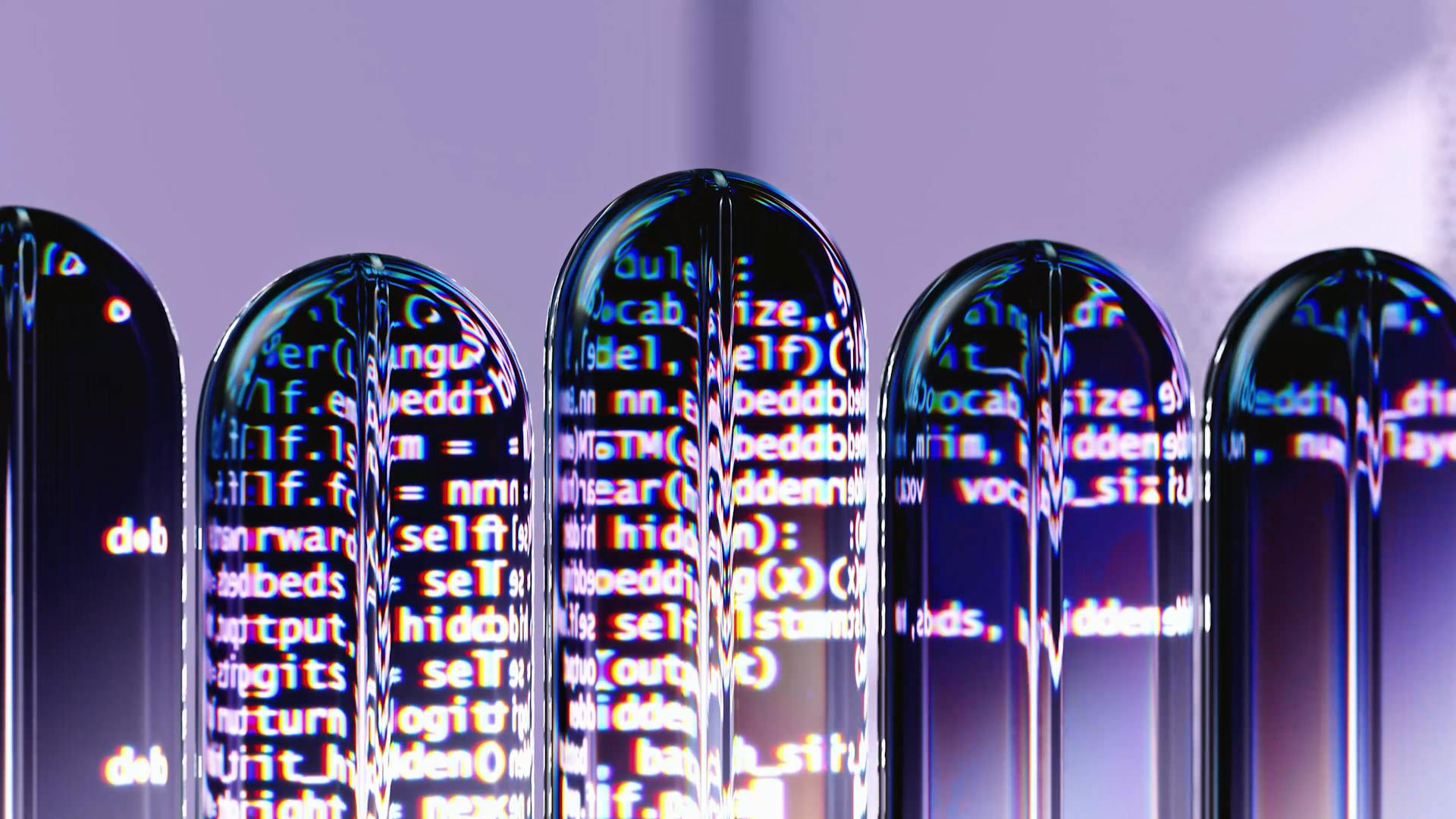
Generative AI is transforming the modern workplace in profound ways. It's not just about automating tasks, but also about augmenting human capabilities.
According to a recent study, 70% of businesses are already using generative AI to improve productivity. This is especially true in industries like customer service, where chatbots and virtual assistants are taking over routine inquiries.
The benefits of generative AI are clear: it can process vast amounts of data, identify patterns, and make predictions with unprecedented accuracy. For instance, a marketing team used generative AI to create personalized ads that resulted in a 25% increase in sales.
However, implementing generative AI also presents significant challenges, such as ensuring data quality and security. A survey found that 60% of organizations struggle with data governance when using generative AI.
What is Generative AI?
Generative AI is a type of artificial intelligence capable of generating new content, including text, images, or code, often in response to a user's prompt.
Its models are increasingly being incorporated into online tools and chatbots, allowing users to type questions or instructions into an input field.
The AI model will generate a human-like response in the output field, making it a powerful tool for creating new content.
Generative AI is being used in a variety of applications, from content generation to code completion, and its capabilities are expanding rapidly.
With its ability to generate human-like responses, generative AI is changing the way we interact with technology and each other.
On a similar theme: How Generative Ai Can Augment Human Creativity
Benefits and Applications
Generative AI is a game-changer for professionals and businesses alike, offering numerous benefits and applications that can revolutionize the way we work.
Generative AI can automate specific tasks, freeing up employees' time and energy to focus on more important strategic objectives, resulting in lower labor costs and greater operational efficiency.
Efficiency can affect a company's bottom line, and generative AI can help achieve it. McKinsey estimates that activities currently accounting for around 30% of U.S. work hours could be automated by 2030, prompted by the acceleration of generative AI.
In the healthcare industry, generative AI is being explored to help accelerate drug discovery, while tools such as AWS HealthScribe allow clinicians to transcribe patient consultations and upload important information into their electronic health record.
Generative AI has found a foothold in a number of industry sectors, including digital marketing, education, finance, and the environment. In digital marketing, advertisers can use generative AI to craft personalized campaigns and adapt content to consumers' preferences.
Some role-specific use cases of generative AI include customer support, software development, and writing. In customer support, AI-driven chatbots and virtual assistants can help businesses reduce response times and quickly deal with common customer queries.
Here are some examples of generative AI applications across different industries:
Generative AI tools can help professionals and content creators with idea creation, content planning and scheduling, search engine optimization, marketing, audience engagement, research, and editing. However, manual oversight and scrutiny of generative AI models remain highly important.
A fresh viewpoint: Generative Ai for Content Creation
Challenges and Concerns
Generative AI at work is an exciting and rapidly evolving field, but it's not without its challenges and concerns.
One major challenge is the scale of compute infrastructure required to train generative models, which can boast billions of parameters and need massive compute power to train, often requiring hundreds of GPUs.
Another challenge is the lack of high-quality data, which can be a major roadblock for generative AI models, especially in domains like 3D assets, where data is scarce and expensive to develop.
The sampling speed of generative models is also a concern, particularly for interactive use cases like chatbots, where conversations must happen immediately and accurately.
To mitigate these challenges, many companies are working to provide services and tools that abstract away the complexities of setting up and running generative models at scale.
Here are some of the key challenges and concerns in generative AI:
- Scale of compute infrastructure
- Lack of high-quality data
- Sampling speed
- Data licenses
Additionally, there are also ethical and privacy concerns, such as the potential for generative AI models to spread misinformation and harmful content, and the risk of legal and financial repercussions from misuse.
Adversarial Networks
Adversarial Networks can create authentic-looking data, like images based on prompts, but they also raise concerns.
Generative adversarial networks, or GANs, comprise two neural networks: a generator and a discriminator. The generator creates convincing output, while the discriminator evaluates its authenticity.
DALL-E and Midjourney are examples of GAN-based generative AI models, developed using this technique. This technology was first developed in 2024.
The generator and discriminator work against each other, with each component getting better at its role over time, resulting in more convincing outputs.
Check this out: Generative Adversarial Networks Ai
Variational Autoencoders
Variational autoencoders are a type of neural network architecture that was first described in 2013.
They work by using two networks, an encoder and a decoder, to interpret and generate data. The encoder compresses the input data into a simplified format.
This compressed information is then used by the decoder to reconstruct something new that resembles the original data but isn't entirely the same.
For example, variational autoencoders could be used to generate human faces using photos as training data. Over time, the program learns to simplify the photos of people's faces into a few important characteristics.
These characteristics can then be used to create new faces that resemble the original data but have some differences. This type of VAE might be used to increase the diversity and accuracy of facial recognition systems.
Intriguing read: Google Announces New Generative Ai Search Capabilities for Doctors
Biases and Misinformation
The potential for biases and misinformation is a major concern when it comes to generative AI tools. The risk of spreading stereotypes, hate speech, and harmful ideologies is very real.
Generative AI can damage personal and professional reputation. This can have severe consequences.
The misuse of generative AI can lead to legal and financial repercussions. It's even suggested that it could put national security at risk if used improperly or irresponsibly.
The European Council has taken action to regulate the use of AI in Europe. On February 13, 2024, they approved the AI Act, a first-of-its-kind legislation designed to regulate AI.
Ethical and Privacy Concerns
Implementing generative AI systems requires careful consideration of ethical and privacy concerns. Generative AI models are often trained on internet-sourced information, which can lead to clashes with media companies over the use of published work.
This means that IT and cybersecurity professionals need to carefully delineate where the model can and cannot access data. In other words, they need to set boundaries on what data the model can use.
Media companies may have concerns about the use of their published work in generative AI models. This highlights the importance of transparency and clear guidelines for data usage.
To ensure responsible scaling, companies should build in guardrails for what users can and can't do with generative AI. This will help keep company and customer data secure and compliant across every automation.
Broaden your view: Companies Using Generative Ai
What Are the Challenges of?
Generative AI models face several challenges that hinder their growth and development. One of the main challenges is the scale of compute infrastructure required to train them. Generative AI models can boast billions of parameters and need fast and efficient data pipelines to train, necessitating significant capital investment, technical expertise, and large-scale compute infrastructure.
To train such large datasets, massive compute power is needed, and AI practitioners must be able to procure and leverage hundreds of GPUs to train their models. This can be a significant barrier for many organizations.
Broaden your view: Are Large Language Models Generative Ai
Another challenge is the sampling speed of generative models, which can lead to latency in generating instances. This is particularly problematic for interactive use cases such as chatbots, AI voice assistants, or customer service applications, where conversations must happen immediately and accurately.
The sampling speeds of diffusion models, for example, have become increasingly apparent due to their slow speeds. This can hinder their adoption in real-time applications.
Generative AI models also struggle with the lack of high-quality data, which is essential for their operation. They require high-quality, unbiased data to train, but not all data is suitable for this purpose. Some domains, such as 3D assets, have limited data availability and are expensive to develop.
To address this issue, organizations need to procure commercial licenses to use existing datasets or build bespoke datasets to train generative models. This is a crucial process to avoid intellectual property infringement issues.
Here are the four main challenges of generative AI models:
- Scale of compute infrastructure
- Sampling speed
- Lack of high-quality data
- Data licenses
Break from Skill-Biased Technologies
Generative AI is breaking away from the traditional "skill-biased" technologies of the past, which mainly substituted routine skills for jobs that didn't require much creativity.
This paradigm shift is significant because generative AI excels at mimicking non-routine skills and interactive traits that were previously thought impossible for computers to perform, such as programming, prediction, writing, creativity, and analysis.
Generative AI is not likely to disrupt physical, routine, blue-collar work much at all, unless there's a breakthrough in robotics technology.
Instead, it's the industries that were previously ranked at the bottom of automation risk that are now facing the greatest exposure to generative AI.
Some of the capabilities that generative AI can perform autonomously without human oversight include tasks such as:
- Programming
- Prediction
- Writing
- Creativity
- Projection of empathy
- Communication
- Persuasion
- Analysis
These capabilities are a stark departure from previous technologies, and they're having a significant impact on various industries and applications.
Implementation and Integration
You can connect to leading large language models (LLMs) and embed generative AI into every automation, helping your teams move faster.
Our platform has been architected as the most modern, open, cloud-native automation platform in the market, making it the perfect platform to put generative AI into action across every system.
We support a wide range of providers, including AWS, Google, OpenAI, Microsoft, Anthropic, and more, so you can choose the models that best suit your unique business requirements.
With over 150 million automations with anonymized metadata, our purpose-built generative AI models are trained to help your teams and business move faster.
Integrate with Best-of-Breed Models
You can connect to leading large language models (LLMs) and embed generative AI into every automation, helping your teams move faster.
The Automation Success Platform has been architected as the most modern, open, cloud-native automaton platform in the market, making it the perfect platform to put generative AI into action across every system.
Choose from purpose-built generative AI models trained on over 150 million automations with anonymized metadata or your LLM of choice: OpenAI on Azure, VertexAI on Google Cloud, or Sagemaker or Amazon Bedrock on AWS.
Securely integrate with and manage generative AI models across a wide range of providers including: AWS, Google, OpenAI, Microsoft, Anthropic, and more.
Transformer-based models, such as ChatGPT-4 and Google Gemini, are well-suited for text-generation tasks due to their ability to understand the structure and context of language.
Check this out: Google Cloud Skills Boost Generative Ai
Process Discovery
Process Discovery is a powerful tool that helps organizations understand their processes and identify areas for automation. AI tracks behavior to understand intent and extract the process.
This process involves developing a map of how tasks are currently being performed, which can be a time-consuming task. AI then develops a process map to be used for automation development.
With AI-powered process discovery, you can automate tasks instantly and start streamlining your workflows.
A unique perspective: Which Term Describes the Process of Using Generative Ai
Automation and Productivity
Generative AI can accelerate automators, enabling everyone from professional developers to business users to transform conversation into automation and expedite the idea-to-ROI journey. This can lead to significant productivity gains, with some organizations reducing time to automate from months to weeks.
With generative AI, you can automate tasks such as complaint resolution, customer inquiry sentiment analysis, and order lookup email triage for consumer packaged goods. These automations can be built with real-time and in-context next possible actions, making them more efficient and effective.
For your interest: Automate It with Zapier and Generative Ai Pdf
Generative AI can also empower developers to build better automations, faster, with a natural language automation assistant embedded in the developer experience. This can scale and accelerate developer productivity, making it easier to automate processes across various systems.
Some examples of automations that can be built with generative AI include:
- Complaint Resolution
- Customer Inquiry Sentiment Analysis
- Order Lookup Email Triage for CPG
- Patient Message Triage
- After-Visit Summary (AVS) for Patient
- Medical Summary for Practitioners
- AML Transaction Monitoring
- Invoice Processing
By automating these tasks, organizations can free up time and resources for more strategic and creative work, leading to increased productivity and efficiency.
Large Language Models
Large Language Models are a crucial part of generative AI, and there are several types to choose from.
Transformer-based models, such as ChatGPT-4 and Google Gemini, are well-suited for text-generation tasks and are trained on large sets of data to understand relationships between sequential information.
These models are adept at natural language processing and understanding the structure and context of language, making them a popular choice for many applications.
Multimodal models, on the other hand, can understand and process multiple types of data simultaneously, such as text, images, and audio, allowing them to create more sophisticated outputs.
A different take: Is Speech to Text Generative Ai
DALL-E 3 and OpenAI’s GPT-4 are examples of multimodal models that can generate an image based on a text prompt, as well as a text description of an image prompt.
To ensure the best results, it's essential to evaluate the models you choose to use and integrate with top-tier enterprise LLMs that have proven output quality and can protect your data.
Choose from leading large language models, such as OpenAI on Azure, VertexAI on Google Cloud, or Sagemaker or Amazon Bedrock on AWS, to find the best fit for your use case.
Curious to learn more? Check out: Telltale Words Identify Generative Ai Text
Future of
Generative AI is poised to revolutionize various industries, with many organizations already establishing guidelines for its use in the workplace. As the technology continues to evolve, its applications and use cases will expand.
In the future, generative AI companies will push the envelope by creating higher-parameter models and photorealistic AI video. Recent advancements, such as OpenAI's o1 model, demonstrate the rapid progress being made in the field.
Worth a look: How Generative Ai Will Transform Knowledge Work
The potential impact of generative AI on work and workers is significant, with some occupations likely to see more disruption than others. According to OpenAI data, certain tasks are more exposed to generative AI technology than others.
Generative AI has the potential to democratize benefits by finding the right mix of automation and human involvement. This will require ongoing updates to laws and regulations to keep pace with the fast-moving technology.
In 2024, OpenAI released its o1 model, which trades speed for complex coding and math processes. This highlights the ongoing efforts to improve the capabilities of generative AI.
Recommended read: The Economic Potential of Generative Ai
Understanding and Analysis
Evaluating generative AI models requires careful consideration of several key factors. High-quality generation outputs are crucial for applications that interact directly with users, as poor speech quality in speech generation can be difficult to understand, and poor image quality in image generation can be visually unappealing.
To ensure diversity in generative models, we need to capture the minority modes in the data distribution without sacrificing generation quality. This helps reduce undesired biases in the learned models. A good generative model should be able to generate a wide range of outputs, including those that are less common in the data.
In many interactive applications, speed is also a critical factor. Real-time image editing, for example, requires fast generation to allow for use in content creation workflows. To achieve this, generative models need to be able to generate outputs quickly without compromising on quality.
You might like: Can I Generate Code Using Generative Ai
Evaluating Models
Evaluating models is crucial to ensure they perform as expected. High-quality generation outputs are key, especially for applications that interact directly with users.
For example, in speech generation, poor speech quality can be difficult to understand. This can lead to frustration and a poor user experience.
A good generative model captures the minority modes in its data distribution without sacrificing generation quality. This helps reduce undesired biases in the learned models.
Speed is also an important consideration, as many interactive applications require fast generation. Real-time image editing, for instance, is necessary for content creation workflows.
To evaluate these factors, consider the following key aspects:
- Quality: How well does the model perform in generating outputs that are easy to understand?
- Diversity: Does the model capture minority modes in its data distribution without sacrificing quality?
- Speed: How quickly can the model generate outputs, and is it suitable for real-time applications?
The Focus of Our Analysis
In our analysis, we're focusing on the key aspects of generative AI models that make them effective and useful. High-quality generation outputs are crucial, especially for applications that interact directly with users.
For instance, poor speech quality in speech generation is difficult to understand, and similarly, in image generation, the desired outputs should be visually indistinguishable from natural images. This is why quality is a top priority.
Additional reading: Synthetic Data Generation with Generative Ai
We're also considering the importance of diversity in generative models, which helps reduce undesired biases in the learned models. A good generative model captures the minority modes in its data distribution without sacrificing generation quality.
In addition, speed is a critical factor in many interactive applications, such as real-time image editing, which allows for use in content creation workflows.
To break it down, here are the key factors we're focusing on:
- Quality: High-quality generation outputs, especially for user-interacting applications.
- Diversity: Capturing minority modes in data distribution without sacrificing generation quality.
- Speed: Fast generation, such as real-time image editing, for interactive applications.
Open Questions: What We Still Don't Know
We still have many open questions in our understanding and analysis of complex systems. One of the biggest challenges is understanding how different components interact with each other.
The concept of emergence is still not fully understood, and it's difficult to predict when and how complex systems will exhibit emergent behavior. We've seen examples of this in the article, such as the flocking behavior of birds, where individual birds following simple rules lead to complex patterns.
For more insights, see: Why Is Controlling the Output of Generative Ai
There's still much to be learned about the dynamics of complex systems, particularly when it comes to their sensitivity to initial conditions. This is evident in the article's discussion of the butterfly effect, where small changes in initial conditions can lead to drastically different outcomes.
The relationship between complexity and predictability is another area where we still have many open questions. As the article shows, complex systems can be highly unpredictable, even with advanced mathematical models.
The limits of our current understanding are often revealed by the failure of simple models to capture the behavior of complex systems. This is a common theme in the article, where simple models are shown to be inadequate for describing complex phenomena.
For another approach, see: How Are Modern Generative Ai Systems Improving User Interaction
Frequently Asked Questions
How is AI used in the workplace?
AI is used in the workplace to automate repetitive tasks and assist with routine decisions, freeing up employees to focus on higher-value work. This can lead to increased productivity and efficiency, and a better work-life balance for employees
Sources
- https://www.automationanywhere.com/products/generative-ai-process-models
- https://writer.com/blog/future-of-work/
- https://www.nvidia.com/en-us/glossary/generative-ai/
- https://www.techrepublic.com/article/what-is-generative-ai/
- https://www.brookings.edu/articles/generative-ai-the-american-worker-and-the-future-of-work/
Featured Images: pexels.com