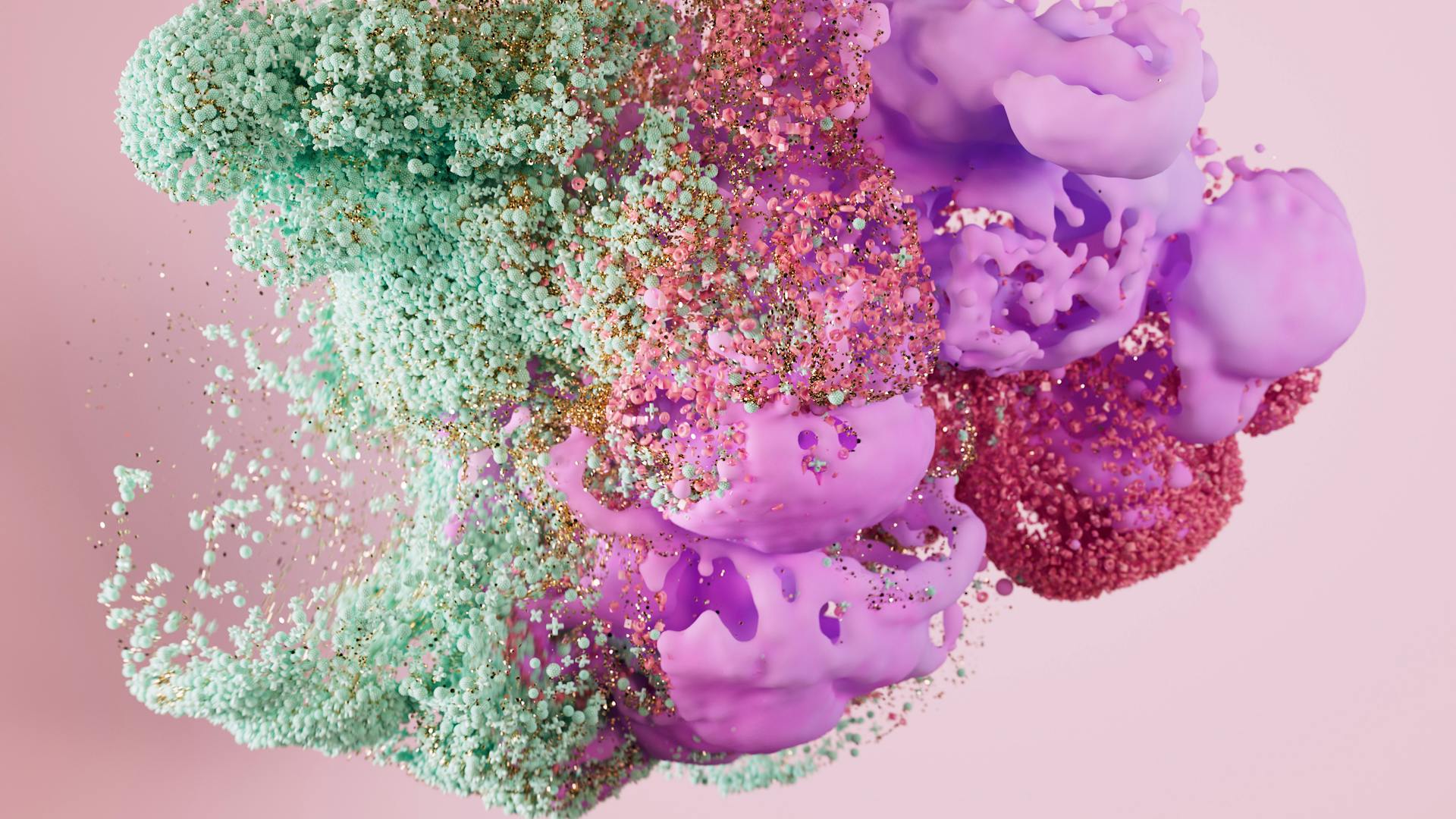
Generative AI in banking is revolutionizing the way financial institutions operate. According to a study, 80% of banks are already exploring or implementing AI technologies to improve their services.
One of the key benefits of generative AI in banking is its ability to automate routine tasks, freeing up staff to focus on more complex and high-value activities. By automating tasks such as data entry and document processing, banks can reduce costs and improve efficiency.
For example, a leading bank has implemented an AI-powered chatbot that has reduced customer query resolution time by 50%. This has not only improved customer satisfaction but also reduced the workload of customer support staff.
As generative AI continues to evolve, we can expect to see even more innovative applications in the banking sector.
A different take: Generative Ai for Customer Experience
Benefits and Applications
Generative AI is transforming the banking industry in profound ways, and understanding its benefits and applications is key to harnessing its potential.
Elevated customer interactions are a major advantage, as generative AI enables more human-like, natural, and conversational exchanges, cultivating deeper engagement and personalization.
Breaking language barriers is another significant benefit, as the technology allows interactions in multiple languages, expanding a bank's reach to a global clientele and fostering inclusivity.
By pinpointing and capitalizing on new opportunities through in-depth analysis of consumer data, transaction histories, and market trends, generative AI unlocks revenue potential.
Real-time risk mitigation is also a critical application, as artificial intelligence can identify anomalies and potential fraud, safeguarding both the institution and its customers.
Streamlined operations and effortless compliance are additional benefits, as generative solutions optimize routine processes, simplify resource allocation, and automate safety checks, reducing the burden on compliance teams and other units.
Here are some of the key applications where generative AI is making the most significant impact:
- Elevated customer interactions
- Breaking language barriers
- Unlocking revenue potential
- Real-time risk mitigation
- Streamlined operations
- Effortless compliance
Fraud Detection and Prevention
Generative AI is a game-changer in the battle against fraudulent activities in banking. By training on past instances of scams and continuously scrutinizing financial operations, it swiftly pinpoints unusual behavior and promptly notifies clients.
Generative AI can help with fraud identification measures in several ways. Behavioral biometrics scrutinize user interactions to detect anomalies that might signal unauthorized access. Real-time transaction monitoring constantly evaluates transactions for suspicious activity, flagging potential threats for immediate investigation. Anomaly detection in large datasets analyzes extensive transaction data to uncover hidden patterns and irregularities that could signify complex fraud schemes.
According to Natalie Faulkner, Global Fraud Lead at KPMG International, banks need to be agile to respond to threats and embrace new approaches and technologies to predict and prevent fraud.
Generative AI can identify abnormal patterns in large volumes of financial transactions and raise alerts immediately, allowing banks to halt suspicious transactions and maintain customer trust.
This technology is expected to help banks improve their fraud detection rate by 20%, with rates reaching as much as 300% in some cases, according to Mastercard.
Generative AI can automate the identification of suspicious behavior, reduce the need for manual review, and minimize financial losses from fraud.
You might like: Anomaly Detection Using Generative Ai
Enhanced Operational Efficiency
Generative AI in banking can significantly enhance operational efficiency. By automating routine tasks with impressive accuracy, processes like document review, data entry, and compliance checks can be handled by AI systems.
This frees up human resources to focus on more impactful activities, leading to greater efficiency. Banks can also use AI to scan, process, and categorize physical documents in secure cloud storage, lifting some of the burden from operation staff.
Data analysis, report generation, and document processing become more streamlined, creating a more responsive financial ecosystem. AI-driven technologies enhance workflows, reduce processing times, and improve decision-making.
Fewer errors, cost savings, and better performance are just a few benefits of implementing generative AI in banking. By leveraging AI, banks can optimize their operations and provide better services to their clients.
Consider reading: Free Generative Ai Application for Document Extraction
Customer Experience and Service
Generative AI is revolutionizing customer service in banking, providing natural language models, personalized communication, and contextual awareness. This results in higher customer satisfaction and loyalty.
For another approach, see: Generative Ai for Customer Service
Banks can automate routine tasks, reducing operational costs and focusing on delivering value-added services.
Generative AI-powered chatbots can generate relevant and personalized responses to customer inquiries, making the banking experience more enjoyable.
In fact, nearly 70% of decision-makers in the banking industry believe that personalization is critical to serving customers effectively.
By analyzing customer data and preferences, AI can suggest customized investment recommendations that align with individual needs and preferences.
This level of personalization boosts customer satisfaction and builds long-term loyalty.
Generative AI can even create customized content, messages, and product offerings that speak to the individual needs and preferences of each customer.
This increases customer engagement and encourages loyalty, in addition to increasing conversion rates.
Banks can also use generative AI to offer customized products and services, such as personalized savings plans and tailored loan options.
Take a look at this: Generative Ai Services
Wealth Management
Generative AI in banking is revolutionizing the way wealth management is handled.
A range of financial data, economic indicators, market trends, and individual client profiles are analyzed by generative AI models to suggest the best asset allocations and investment plans.
These models can dynamically adjust portfolios when opportunities and market conditions change in real-time, efficiently managing potential risks and optimizing returns.
With AI-driven wealth management, banks can increase client satisfaction and loyalty by offering more individualized asset allocations and investment strategies.
More than 70% of decision-makers in the banking industry believe that personalization is critical to serving customers effectively, and generative AI is helping to bridge this gap.
Security and Compliance
Generative AI in banking can be a double-edged sword, offering improved security measures while introducing data risks and compliance challenges.
Financial regulations can restrict banks from using certain data to train deep learning models, posing a significant obstacle to generative AI adoption.
Generative AI strengthens security within financial institutions by analyzing vast datasets to detect unusual patterns and potential security breaches.
This technology supports the development of advanced cybersecurity measures, including better authentication protocols and protections for sensitive financial information.
Navigating the complex web of financial regulations is one of the main obstacles to generative AI in banking, with strict regulations in place to safeguard consumer interests.
To stay out of legal issues, financial companies must ensure that AI adoption is compliant with current regulations.
For more insights, see: Generative Ai Security
Implementation and Challenges
Implementing generative AI in banking requires careful planning and strategic execution. This is a significant step that needs to be navigated with ease and peace of mind.
OTAKOYI's team of experts will work closely with you to understand your specific needs and challenges. They'll develop customized generative AI solutions that align with your business goals, ensuring a seamless integration process.
The advantages of working with OTAKOYI include profound expertise and knowledge, as well as life-long learning and only the latest technologies.
Challenges of Implementation
Implementing Generative AI in financial services isn't a straightforward process. There are challenges to overcome, but with careful management, long-term benefits can be achieved.
One of the key challenges is determining the challenges themselves. We wouldn't want you to think that implementing Gen AI is all about flowers and candies, as there are some challenges to overcome.
Careful management is crucial to ensuring long-term benefits. However, we're not given specific details on what this management entails.
Explore further: Generative Ai Benefits
Implementing Gen AI requires financial institutions to be aware of the challenges they'll face. Determining the challenges of Gen AI implementation is a crucial step in this process.
Financial institutions need to be aware of the potential challenges to overcome. We're not given specific information on what these challenges are, but it's clear that they exist.
Implementation and Challenges
Implementing generative AI in financial services is not a straightforward process, as there are several challenges to overcome. One of the key challenges is ensuring long-term benefits, which requires careful management.
Financial institutions need to have the capabilities to scale machine learning technology organization-wide, rather than maintaining siloed operations. This involves consolidating different departments and preparing staff with skills, values, and mindsets to embrace AI.
Banks must also reexamine their technological capabilities and infrastructure to support AI systems, including secure storage of large amounts of data. This is crucial for training and deploying generative AI models.
Embedding generative AI models to support decision-making in different domains requires a pragmatic approach, with proper risk assessment and continuous improvement of the AI models.
Banks and Institutions
Organizations like Swift, ABN Amro, ING Bank, BBVA, and Goldman Sachs are experimenting with Generative AI in banking to automate processes, enhance customer interactions, and more.
Most banks are still in the early stage of deploying generative AI, but leading players are proactively exploring opportunities.
They're focusing on developing lower-risk, internal-facing applications that demonstrate tangible productivity benefits in day-to-day operations.
Generative AI-powered "co-pilot" tools are being designed to augment employee decision-making processes, particularly within the back- and middle-office functions.
These tools are often off-the-shelf solutions with simple contextualization and customization options, making them scalable and catering to various departments.
Companies like Marsh McLennan have already deployed proprietary generative AI assistants, such as LenAI, which have reported significant benefits.
Early adopters of LenAI reported saving an average of eight hours per week and spending 20% less time on simple, repetitive tasks.
A unique perspective: What Are the Generative Ai Tools
Cases and Examples
Generative AI in banking can bring about significant changes by automating numerous human interaction points. Banking is an industry that involves numerous human interaction points.
Recommended read: How Generative Ai Can Augment Human Creativity
One of the most effective examples of the application of generative AI in banking is automating customer service. Generative AI can be used to create chatbots that can respond to customer inquiries and provide personalized support.
Generative AI can also be used to create personalized loan offers for customers. This can be done by analyzing customer data and generating offers that are tailored to their specific needs and financial situation.
Generative AI can help banks to reduce their operational costs by automating tasks such as data entry and document processing. This can be done by using generative AI to create digital versions of documents and automate the data entry process.
Generative AI can also be used to detect financial crimes such as money laundering and fraud. This can be done by analyzing large amounts of data and identifying patterns that may indicate suspicious activity.
Take a look at this: Generative Ai for Cybersecurity
Future and Risks
The future of Generative AI in banking looks incredibly promising. Generative AI can be used to create virtual assistants for employees and customers, speed up software development, and make customized content. It's expected that Generative AI in banking could boost productivity by 2.8% to 4.7%, adding about $200 billion to $340 billion in revenue.
However, this technology also comes with risks. Banks must adopt a cautious, responsible approach to integrate Generative AI, with proper mitigation strategies like robust data governance, rigorous testing and validation, prioritization of transparency and explainability, and an ethical AI framework.
The risks include bias and fairness, privacy and security, reputational risk, performance and explainability risks, strategic risks, and third-party risks. Some of the specific risks include perpetuating biases, unauthorized data disclosure, malicious exploitation, and noncompliance with ESG standards or regulations.
Check this out: Risks of Generative Ai
Future of
The future of Generative AI in banking is incredibly promising, with the potential to boost productivity by 2.8% to 4.7% and add $200 billion to $340 billion in revenue.
Generative AI can be used to create virtual assistants for employees and customers, speed up software development, and make customized content.
Financial institutions are already actively employing Gen AI in their operations, and the technology's potential for transforming the industry is vast.
If this caught your attention, see: The Economic Potential of Generative Ai
By 2026, front-office employee efficiency is expected to boost by 27% to 35%, resulting in up to $3.5 million in additional revenue per employee.
Banks need a solid AI implementation strategy to start with, but once implemented, both bankers and customers will benefit from the efficiency and personalization that generative AI brings.
Generative AI will transform how banks operate and engage their customers, with use cases similar to ChatGPT but repurposed for finance operations.
Risks
Generative AI in finance and banking comes with its share of risks. Bias and fairness are major concerns, as AI models can perpetuate and intensify biases in training data, leading to unfair or discriminatory outcomes.
The use of sensitive financial records raises concerns about privacy breaches and cybersecurity threats. This includes unauthorized data disclosure and malicious exploitation by bad actors.
Misuse of AI or unforeseen errors could damage a bank's reputation and erode customer trust. This reputational risk can be severe and long-lasting.
Models may provide factually incorrect answers or outdated information, and their decision-making processes may lack transparency. This performance and explainability risk can undermine trust in the bank.
Noncompliance with ESG standards or regulations due to AI implementation could lead to societal or reputational harm. This strategic risk can have far-reaching consequences.
Banks must establish a robust risk and control framework to effectively manage risks emerging from generative AI. This framework should prioritize the management of reputational and legal risks.
Here are some of the risks associated with generative AI in finance and banking:
- Bias and fairness
- Privacy and security
- Reputational risk
- Performance and explainability risks
- Strategic risks
- Third-party risks
Technology and Integration
Leading banks are focusing on integrating generative AI with other AI technologies to unlock new capabilities. This integration will propel the potential of AI within the financial industry.
Generative AI is being combined with conventional predictive AI/machine learning technologies to create sophisticated applications. We've already seen impactful pilot applications, such as one prominent global bank empowering its risk and compliance managers with real-time regulatory updates and key insights.
Here's an interesting read: Generative Ai Applications
One innovative solution automatically keeps compliance managers informed about regulatory updates and changes, extracting key insights in a manner that aligns with their expertise. This is a big win for the bank, transforming its operating model.
A bank deployed a surveillance tool that combines generative AI and reinforcement learning, monitoring employees' social media marketing activities and flagging suspicious or unauthorized behavior.
Regulations and Governance
Navigating the complex web of financial regulations is one of the main obstacles to generative AI in banking. Strict regulations are in place to safeguard consumer interests, stop fraud, and uphold market integrity in the financial sector.
Inaction is not the low-risk option when it comes to generative AI, and banks must establish a robust risk and control framework to effectively manage risks emerging from generative AI. This framework should prioritize the management of reputational and legal risks.
Consulting firm Marsh McLennan's new generative AI tool, LenAI, prioritizes security, setting it apart from competitors and making it ideal for business.
Explore further: Generative Ai Regulation
Compliance and Regulations
Navigating the complex web of financial regulations is a significant obstacle to generative AI in banking. Strict regulations are in place to safeguard consumer interests, stop fraud, and uphold market integrity.
Financial companies must ensure that AI adoption is compliant with current regulations to stay out of legal issues and uphold financial service industry standards. Compliance is crucial to avoid reputational and legal risks.
Regulations are in place to protect consumers and maintain market integrity. This means financial companies need to be mindful of the long-term impact of generative AI on their standing.
Consulting firm Marsh McLennan’s new generative AI tool, LenAI, prioritizes security, setting it apart from competitors and making it ideal for business. This highlights the importance of prioritizing security and compliance in AI adoption.
Here's an interesting read: Generative Ai Adoption
Quality Data Availability
Ensuring quality data availability is crucial for AI in financial services, as it prevents inaccurate data from compromising AI-driven insights.
Access to sensitive databases can be a challenge, and accuracy may be compromised, which can lead to knowledge gaps that impair the functionality of AI models.
To resolve data availability concerns, financial institutions must prioritize high-quality data inputs, which is essential for accurate AI-driven insights.
Inaccurate data can compromise the entire AI system, making it imperative to ensure high-quality data inputs from the start.
Broaden your view: Generative Ai Photoshop Increase Quality
Balancing Risk and Governance
Banks must establish a robust risk and control framework to effectively manage risks emerging from generative AI.
Inaction is not the low-risk option when it comes to generative AI. Banks must establish a robust risk and control framework, setting guardrails firmly grounded in a comprehensive understanding of AI risks and their potential consequences.
Existing risk management processes need an overhaul to effectively manage risks emerging from generative AI. These processes often focus on traditional model risk metrics such as minimizing error rates, an approach that may be myopic.
Organizations must recognize the long-term impact of generative AI on their standing and prioritize the management of reputational and legal risks. Marsh McLennan’s new generative AI tool, LenAI, prioritizes security, setting it apart from competitors and making it ideal for business.
The limitations and risks of generative AI in banking include bias and fairness, privacy and security, reputational risk, performance and explainability risks, strategic risks, and third-party risks.
Related reading: How Has Generative Ai Affected Security
Here are some of the key risks associated with generative AI in banking:
- Bias and fairness: AI models can perpetuate and intensify biases in training data, leading to unfair or discriminatory outcomes.
- Privacy and security: Generative AI raises concerns about privacy breaches and cybersecurity threats, including unauthorized data disclosure and malicious exploitation.
- Reputational risk: Misuse of AI or unforeseen errors could damage a bank’s reputation and erode customer trust.
- Performance and explainability risks: Models may provide factually incorrect answers or outdated information, and their decision-making processes may lack transparency.
- Strategic risks: Noncompliance with ESG standards or regulations due to AI implementation could lead to societal or reputational harm.
- Third-party risks: Reliance on external tools or unknown vendors could expose banks to data leakage or other vulnerabilities.
Frequently Asked Questions
How big is the generative AI market in banking?
The global generative AI market in banking is projected to reach USD 21,824.46 million by 2034, growing at a significant 33% CAGR. This rapid growth highlights the increasing adoption of generative AI in the banking sector.
What is generative AI for central banks?
Generative AI for central banks enhances crisis prediction and mitigation, while improving monetary policy and financial regulation
What AI is used in financial services?
Machine learning (ML) is the primary AI technology used in financial services to enhance analysis, management, and decision-making. It enables financial institutions to make more informed and efficient decisions about money
What are the solid results from generative AI in financial services?
Generative AI detects and prevents financial fraud by identifying anomalies and patterns in transactions. It also safeguards sensitive data with robust encryption and access controls
Which banks use generative AI?
Several major banks, including Swift, ABN Amro, ING Bank, BBVA, and Goldman Sachs, are leveraging Generative AI. These industry leaders are using the technology to transform various aspects of their banking operations.
Sources
- Morgan Stanley (openai.com)
- Citigroup (bloomberg.com)
- Mastercard (mastercard.com)
- OCBC (ocbc.com)
- Erica (americanbanker.com)
- Deutsche Bank (db.com)
- BBVA (bbva.com)
- How Banks Can Adopt Generative AI In The Future (oliverwyman.com)
- Capturing the full value of generative AI in banking (mckinsey.com)
- Deutsche Bank (db.com)
- Accenture forecasts (accenture.com)
- Mastercard (cnbc.com)
Featured Images: pexels.com