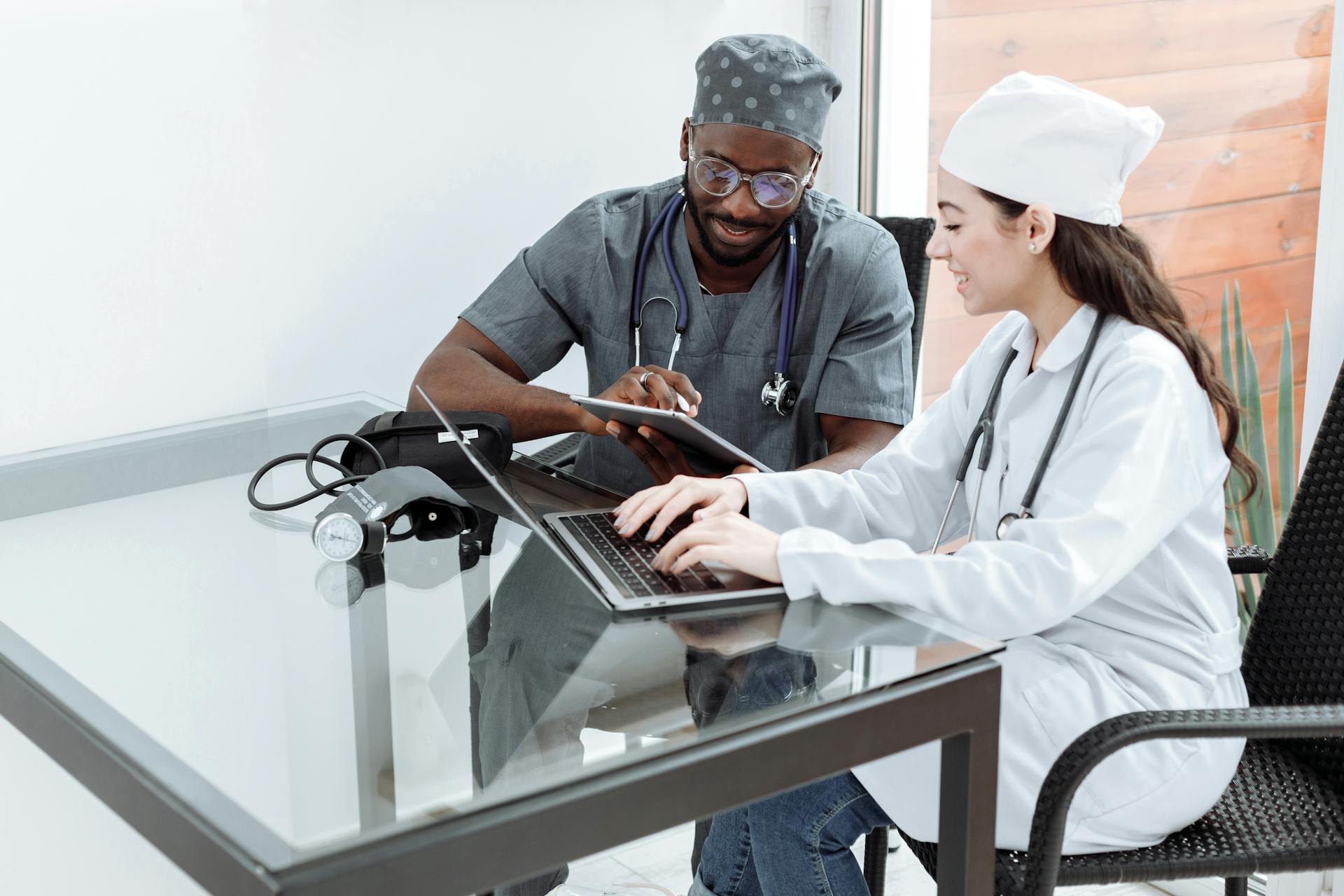
Genai healthcare is revolutionizing the way we approach patient care.
With the ability to analyze vast amounts of medical data, genai healthcare systems can identify patterns and correlations that human clinicians might miss.
This can lead to more accurate diagnoses and more effective treatment plans.
Personalized medicine is a key benefit of genai healthcare, allowing doctors to tailor treatments to an individual's unique genetic profile.
Benefits of
Generative AI is revolutionizing medicine and healthcare by leveraging vast amounts of data to generate predictions, simulations, and insights.
By learning from complex datasets, Gen AI produces novel, actionable intelligence, unlocking unprecedented efficiencies and capabilities in healthcare. This technology is enhancing disease diagnosis, treatment personalization, drug discovery, patient care, and medical research.
Generative AI has achieved remarkable feats, such as passing the US medical licensing exam and effectively summarizing clinical notes, demonstrating its advanced understanding of complex medical concepts and terminology.
John Snow Labs shares insights from deployed software, highlighting the importance of tailoring generative models to meet healthcare-specific needs. Their experiences provide a grounded perspective on both the potential and the pitfalls of these technologies.
The emergence of Gen AI technology in the field of healthcare has brought forth opportunities to improve patient outcomes, streamline operations, and drive innovation throughout the healthcare system.
Generative AI models allow healthcare providers to segment high-risk patient groups using free-text prompts, improving the accuracy of patient treatment strategies. This application of Gen AI helps healthcare providers implement tailored interventions and improve patient outcomes by offering more precise data-driven insights.
The Boston Consulting Group (BCG) highlights the ability of Gen AI in healthcare to significantly enhance efficiency and care quality. They also stress the importance of addressing challenges such as data privacy and bias.
By integrating insights from John Snow Labs and aligning with the recommendations of BCG, healthcare providers can better navigate the challenges of deploying Gen AI in healthcare.
Consider reading: Ai and Ml in Data Analytics
Disease Diagnosis and Treatment
Generative AI is revolutionizing disease diagnosis by enhancing accuracy and efficiency in interpreting data. This technology extracts insights from physician records, patient histories, and diagnostic reports, facilitating precise diagnosis.
For conditions like cancer, diabetes, and heart diseases, early detection can be life-saving. AI-driven diagnostic tools can analyze thousands of images rapidly, identifying patterns invisible to the human eye.
Traditional diagnostic methods can be time-consuming and prone to human error. Gen AI, particularly through deep learning models, has shown remarkable accuracy in diagnosing diseases from medical images.
AI algorithms have outperformed human radiologists in detecting diseases such as breast cancer in mammograms. A study published in Nature (McKinney et al., 2020) supports this finding, highlighting the potential of AI-driven diagnostic tools.
Advanced NLP and machine learning models can analyze clinical notes, radiology images, and other medical data to assist in early and accurate disease detection. This can ultimately improve patient outcomes.
AI solutions can analyze vast amounts of data to create personalized treatment plans, ensuring that patients receive the most effective care tailored to their specific needs.
Readers also liked: Ai Trading Pattern Detection
Genai Healthcare Applications
Genai is transforming the healthcare industry in numerous ways, from improving disease diagnosis to streamlining drug discovery and development.
Generative AI is being used to create synthetic medical images for training and research, enhancing the skills of medical professionals without compromising patient privacy. This technology can also reconstruct high-resolution images from low-quality inputs, improving diagnostic accuracy.
By analyzing patient data, genai models can help healthcare providers deliver personalized care plans, taking into account individual patient histories, preferences, and genetic information to suggest tailored treatments and interventions.
Some key applications of genai in healthcare include:
These applications are already being implemented in various healthcare organizations, with promising results. As genai continues to evolve, we can expect even more innovative applications in the healthcare industry.
You might like: Machine Learning Healthcare Applications
Chatbots
Chatbots are revolutionizing the way patients interact with healthcare systems. Medical chatbots powered by Gen AI are available around the clock to provide communication services, offering advice, answering questions, and guiding patients through their healthcare journey.
These chatbots use natural language processing to assess symptoms, suggest steps, and even notify healthcare providers in some cases. This improves access to care and optimizes healthcare resources.
Readers also liked: What Are Genai Use Cases Agents Chatbots
John Snow Labs offers technologies to develop medical chatbots that provide reliable and instant support to patients. These chatbots can triage symptoms, answer health-related questions, and even facilitate virtual consultations, improving access to care.
By analyzing patient data, John Snow Labs' AI models can help healthcare providers deliver personalized care plans. Our solutions take into account individual patient histories, preferences, and genetic information to suggest tailored treatments and interventions.
Here are some key features of medical chatbots:
HCA Healthcare is collaborating with Google Cloud to streamline documentation processes, easing the workload for doctors and nurses.
Transforming Imaging
Gen AI is transforming medical imaging by enhancing the quality of imaging data and automating the detection of abnormalities. This not only improves diagnostic accuracy but also reduces the workload on radiologists.
AI algorithms can reconstruct high-resolution images from low-quality inputs, making it possible to create synthetic medical images for training purposes. This helps to overcome the challenges of data privacy and availability.
Consider reading: Ai and Ml Images
Generative models can create synthetic medical images that are indistinguishable from real ones, helping to enhance the skills of medical professionals without compromising patient privacy. This is especially useful in cases where real medical images are not available for training.
AI-powered diagnostic tools can analyze thousands of images rapidly, identifying patterns invisible to the human eye and providing clinicians with highly accurate diagnostic information. For instance, AI algorithms have outperformed human radiologists in detecting breast cancer in mammograms.
The Netherlands Cancer Institute used NVIDIA and VMware's AI solution to deploy AI workloads on virtualized servers, improving performance, scalability, and efficiency. This is just one example of how AI is being used to advance cancer research.
United Imaging Healthcare deployed NVIDIA AI and accelerated computing solutions in an AI-enabled MR scanner to reduce patient time in MR machines while increasing access to MR procedures. This shows how AI can be used to enhance the speed and quality of medical imaging.
Take a look at this: What Are the Four Commonly Used Genai Applications
Interpretability and Transparency
Generative AI models in healthcare are often criticized for their "black box" nature, making it difficult for healthcare professionals to understand how decisions are made.
This lack of interpretability and transparency can hinder trust and adoption.
John Snow Labs addresses this challenge by offering AI solutions that prioritize explainability.
Their platforms are designed to provide clear, understandable insights into how AI models reach their conclusions.
This enhances trust among clinicians and patients alike.
John Snow Labs' approach is a crucial step towards making generative AI more trustworthy and reliable in the healthcare industry.
For your interest: Key Challenges Faced by Genai Models
NVIDIA Opens BioNeMo for Digital Biology
NVIDIA has opened BioNeMo, a platform that enables digital biology for global biopharma and scientific industries. This platform is being used by organizations such as Amgen, Argonne National Laboratory, and Flagship Pioneering to advance biomolecular science.
BioNeMo is a powerful tool that allows researchers to train large language models (LLMs) on proprietary data, predicting protein properties and developing biologics with enhanced properties. This is demonstrated by Amgen's use of NVIDIA DGX Cloud and BioNeMo to train LLMs on their proprietary data.
Discover more: Getty Images Nvidia Generative Ai Istock
NVIDIA's collaboration with Microsoft is also supporting global AI startups across industries, with an initial focus on healthcare and life sciences companies. This partnership aims to accelerate the development of AI solutions for these industries.
BioNeMo's capabilities are being leveraged by various organizations to advance their research and development efforts. For instance, Amgen is using BioNeMo to predict protein properties and develop biologics with enhanced properties.
Here are some key features and applications of BioNeMo:
- Training large language models (LLMs) on proprietary data
- Predicting protein properties
- Developing biologics with enhanced properties
- Accelerating biomolecular science research and development
- Supporting global biopharma and scientific industries
By opening BioNeMo, NVIDIA is providing researchers and developers with a powerful tool to advance biomolecular science and accelerate the discovery of new treatments and therapies.
Challenges and Limitations
Deploying Generative AI in healthcare is not without its challenges, which include issues related to interpretability, data requirements, transparency, ethics, risk, and bias.
Interpretability is a major hurdle, as it's difficult for healthcare professionals to understand how a generative AI model arrived at a particular diagnosis or treatment plan.
Data requirements can be a challenge, as generative AI models need large amounts of high-quality data to train effectively, which can be time-consuming and expensive to collect.
Transparency is also a concern, as healthcare professionals need to be able to trust the decisions made by a generative AI model, but it's hard to achieve transparency when the inner workings of the model are complex and difficult to understand.
Ethics and risk are also major concerns, as generative AI models can perpetuate existing biases and make decisions that have serious consequences for patients.
Broaden your view: Data Labeling Companies
Data Limitations
Deploying generative AI in healthcare is not without its challenges, which include issues related to interpretability, data requirements, transparency, ethics, risk, and bias.
Extracting the greatest value from the gen-AI opportunity will require broad, high-quality data sets, making data fidelity and accuracy a top priority.
Improving data quality through strategic partnerships and interoperability investments is crucial for healthcare leaders to unlock the full potential of gen AI.
For more insights, see: Ai Training Data Center
To train gen-AI models, organizations should ensure they are processing data within secure firewalls, and may choose to outsource various parts of their tech stack after evaluating their internal capabilities.
Healthcare leaders must assess their AI tech stack to determine where their technological capabilities will need to be augmented to leverage large language models at scale.
Here's an interesting read: Generative Ai Capabilities
Data Security
Data Security is a significant challenge in the field of AI, particularly when it comes to handling sensitive information like healthcare data. HIPAA regulations in the United States must be strictly complied with, and data platforms and AI models must be built with privacy-by-design principles.
Data platforms and AI models can be built with robust encryption and anonymization techniques to ensure secure data handling. This approach makes it easier for enterprises to manage risk and maintain data security.
The need for large datasets in training AI models raises concerns about data privacy and security. Data handling must comply with strict regulations, and enterprises must prioritize secure data management.
John Snow Labs offers a solution to these concerns by ensuring that data platforms and AI models are built with privacy-by-design principles. This approach facilitates secure data handling and compliance with healthcare data regulations.
Recommended read: Generative Ai Design
Bias and Fairness
Bias and fairness are crucial considerations when it comes to deploying generative AI in healthcare. AI systems can inadvertently learn and perpetuate biases present in their training data, leading to unfair or biased outcomes in patient care.
John Snow Labs helps healthcare organizations develop AI models that are fair and equitable through diverse and comprehensive datasets, along with bias detection and mitigation tools. Their commitment to diversity in data collection and algorithmic transparency is key to minimizing bias.
Protecting members' and patients' personally identifiable information is essential, as open-source gen-AI tools may not provide the necessary level of security. This risk is particularly concerning as the technology evolves.
Integrating gen-AI platforms with other hospital systems, such as billing systems, can lead to inefficiencies and erroneous expenses if done incorrectly.
Scalability and Integration
Scalability and integration are crucial for healthcare enterprises looking to deploy genai solutions. Healthcare enterprises often struggle with scaling AI solutions across different departments and integrating them with existing IT infrastructure.
Additional reading: Ai & Ml Solutions
To overcome these challenges, John Snow Labs provides scalable AI platforms and integration services that ensure seamless deployment of AI applications across various healthcare settings. Its solutions are designed to work with existing healthcare IT ecosystems, facilitating smoother adoption and scalability.
For genai solutions to be successful, integration with existing healthcare systems and workflows is essential. This involves technical integration into IT systems, as well as ensuring that healthcare professionals are trained to utilize these new tools effectively.
Scalability and Integration
Scalability and Integration is a crucial aspect of deploying AI solutions in healthcare. Scalability refers to the ability to expand or contract the size of an AI system to meet changing demands.
Healthcare enterprises often struggle with scaling AI solutions across different departments and integrating them with existing IT infrastructure. This can be a major challenge, but there are solutions available.
John Snow Labs provides scalable AI platforms and integration services that ensure seamless deployment of AI applications across various healthcare settings. Their solutions are designed to work with existing healthcare IT ecosystems, facilitating smoother adoption and scalability.
For successful integration, technical integration into IT systems is essential. This involves aligning Gen-AI applications with clinical goals and patient care standards to ensure they add value without disrupting care delivery.
Here are the key steps to successful integration:
Scaling Gen AI solutions involves expanding their application across different departments, healthcare settings, and patient populations. This requires a flexible, scalable technology infrastructure and a strategic approach to deployment.
Enterprises Can Deploy
To deploy generative AI in healthcare, enterprises need a clear strategic plan that outlines specific objectives and challenges to address. This plan should include identifying areas such as improving diagnosis accuracy, personalizing patient care, or streamlining drug discovery.
A key aspect of successful deployment is strategic planning and investment in technology, including computing resources capable of processing big data and securing access to AI models tailored for healthcare applications.
Investment in the right technology infrastructure is crucial for effective deployment, including computing resources capable of processing big data.
To ensure seamless integration, enterprises must align Gen-AI applications with clinical goals and patient care standards, and also train healthcare professionals to utilize these new tools effectively.
Successful integration involves technical integration into IT systems, as well as ensuring that healthcare professionals are trained to utilize these new tools effectively.
The power of Gen AI in healthcare comes from its ability to analyze and generate insights from big data, which requires establishing mechanisms for collecting, storing, and analyzing large volumes of data from various sources.
To scale Gen AI solutions, enterprises need a flexible, scalable technology infrastructure and a strategic approach to deployment that can adapt to varying needs and scales of operation.
Here are the key steps for successful deployment:
- Strategic Planning and Investment: Develop a clear plan and invest in the right technology infrastructure.
- Successful Integration: Ensure seamless integration into existing healthcare systems and workflows.
- Leveraging Big Data: Establish mechanisms for collecting, storing, and analyzing large volumes of data.
- Scaling: Expand application across different departments, healthcare settings, and patient populations.
Industry and Partnerships
Industry and Partnerships are key drivers in the adoption of genAI healthcare. NVIDIA is collaborating with Deloitte to deploy digital AI agents for healthcare, improving patient experiences and reducing administrative burden.
Microsoft and Epic are integrating genAI into clinical software, allowing for automated drafting of patient messages and data analysis. This partnership has resulted in the first sites integrating GPT into EHR workflows.
Google's Med-PaLM 2 is a large language model specifically trained on medical data, enabling hospitals to analyze data and diagnose complex diseases. Nuance's integration of GPT-4 into clinical note-taking software aims to reduce physician burnout by automating note-taking tasks.
Several startups and established companies are racing to be the next manufacturing leader of genAI-powered healthcare tech, including Suki, which has launched its genAI-powered "Gen 2" for clinical note-taking.
If this caught your attention, see: Claude Ai Gpt
Industry Advancements and Competition
Microsoft and Epic have integrated generative AI into clinical software, allowing for automated drafting of patient messages and bringing genAI to Epic's hospital database.
Google's large language model, Med-PaLM 2, is specifically trained on medical data and is being used for use case trials with specific customers.
Hospitals can use Med-PaLM to analyze data and help diagnose complex diseases, fill out records, or act as a concierge for patient portals.
Intriguing read: Generative Ai Healthcare Use Cases
Microsoft's Nuance is providing a solution to streamline medical note-taking by integrating GPT-4 into its clinical note-taking software.
Nuance is set to make its documentation product, DAX Express, available to the public in early fall of 2024 after a private preview with 300 to 500 physicians.
Suki, a documentation company partnering with Google, has launched its genAI-powered "Gen 2", which can generate clinical notes by listening to conversations and filling in notes automatically.
Gen-AI technology has the potential to affect everything from continuity of care to clinical operations and contracting to corporate functions within hospitals and physician groups.
Back-office work and administrative functions within hospitals can be improved with gen AI, which can use unstructured purchasing and accounts payable data and address common hospital employee IT and HR questions through gen-AI chatbots.
Gen AI could generate discharge summaries or instructions in a patient's native language, synthesize care coordination notes or shift-hand-off notes, and create checklists, lab summaries from physician rounds, and clinical orders in real time.
Invest in People and Partnerships
As healthcare organizations consider bringing gen-AI to their operations, it's essential to remember that this technology will affect not only how work is done but also by whom it is done. A human-in-the-loop approach will be critical, where even though many processes may fundamentally change, people will still be critical to all areas touched by gen-AI.
Healthcare professionals will see their roles evolve as gen-AI helps streamline some of their work. To help bring these changes to healthcare, organizations must learn how to use gen-AI platforms, evaluate recommendations, and intervene when the inevitable errors occur. In other words, AI should augment operations rather than replace them.
Healthcare organizations may need to provide learning resources and guidelines to upskill employees. And within hospitals and physician group settings—where burnout is already high—leaders should find ways to make gen-AI-powered applications as easy as possible for frontline staff to use, without adding to their workloads or taking time away from patients.
You might like: How Generative Ai Is Changing Creative Work
Here are some key considerations for healthcare organizations looking to form strategic partnerships with technology firms:
- Adherence to regulatory compliance requirements, such as HIPAA
- Data privacy and security
- Whether the healthcare organization's data will be used to inform future foundational models
By prioritizing the responsible and safe use of this technology, healthcare leaders can protect patient privacy, create conditions for equitable clinical outcomes, and improve the experience of healthcare providers.
Real-World Implementations
Generative AI is being used in healthcare to improve patient outcomes and streamline operations.
John Snow Labs has shared insights on deploying Generative AI models in healthcare, highlighting the importance of addressing challenges like hallucinations, robustness, and biases.
The talk "Lessons Learned Applying Generative AI Models in Healthcare" provides a grounded perspective on the potential and pitfalls of these technologies.
Generative AI has achieved remarkable feats, such as passing the US medical licensing exam and effectively summarizing clinical notes.
John Snow Labs has developed a software that uses Retrieval-Augmented Generation (RAG) on FHIR, making healthcare data more accessible and transparent.
Precision population health management is another compelling use case of Gen AI, allowing healthcare providers to segment high-risk patient groups using free-text prompts.
Healthcare-specific Large Language Models (LLMs) have also proven beneficial in data discovery from clinical notes, enhancing the accuracy of generative AI outputs.
The Boston Consulting Group (BCG) highlights the potential of Gen AI in healthcare to enhance efficiency and care quality, while also stressing the importance of addressing challenges like data privacy and bias.
The Brookings Institution emphasizes the need for transparency, ethical standards, and data governance frameworks to ensure the benefits of Generative AI in healthcare are distributed equitably.
Insurance executives, hospital administrators, and physician group operators are exploring the use of gen-AI technology across the value chain, from continuity of care to network and market insights.
By integrating insights from John Snow Labs and aligning with the recommendations of BCG and Brookings, healthcare providers can better navigate the challenges of deploying genAI in healthcare.
Here's an interesting read: What Is One of the Key Challenges Faced by Genai
Accelerated Computing Solutions
Accelerated computing solutions are revolutionizing the field of genai healthcare. NVIDIA's RAPIDS technology reduced analysis time on 4 million-cell datasets from 10 hours to just three minutes at TGen, a nonprofit genomics institute.
This is achieved through the use of AI-powered tools that can simulate drug interactions with biological targets at a scale and speed impossible for human researchers. For example, Atomwise uses AI to predict molecule behavior, accelerating the identification of potential drug candidates for diseases like Ebola and multiple sclerosis.
One notable example of accelerated computing in action is the use of NVIDIA's DGX BasePOD with MONAI and NVIDIA FLARE to generate comprehensive datasets and rapidly iterate on AI models, bringing AI into clinical tools faster at the University of Wisconsin-Madison.
You might enjoy: Genai Use Cases
Simulations
Simulations are a game-changer in healthcare, allowing medical professionals to practice and enhance their skills in a risk-free environment. This is made possible by Gen AI technology, which replicates procedures or rare medical scenarios, providing invaluable hands-on experience without endangering real patients.
Medical simulations can be used to practice various procedures, such as surgeries or patient interactions, and can even be tailored to specific medical conditions or scenarios. John Snow Labs offers simulation technologies that allow healthcare professionals to train and plan in a risk-free environment, enhancing clinical skills and preparedness.
For more insights, see: Genai Free
By using simulations, healthcare professionals can improve their skills and preparedness, leading to better patient outcomes. This is especially important for rare or complex medical conditions, where real-world experience may be limited.
Here are some examples of how simulations are being used in healthcare:
Medical simulations are also being used to accelerate the learning process for medical practitioners. By providing a realistic and immersive experience, simulations can help healthcare professionals develop the skills and confidence they need to provide high-quality care.
Accelerated Computing Solutions
Accelerated computing solutions are revolutionizing the way we approach complex problems in healthcare. With NVIDIA's RAPIDS, TGen reduced analysis time on 4 million-cell datasets from 10 hours to just three minutes.
This is just one example of the incredible speed and efficiency gains that accelerated computing can bring. By leveraging AI and accelerated computing, researchers can now analyze large datasets in a fraction of the time, unlocking new insights and discoveries.
Readers also liked: Time Magazine Ai
One area where accelerated computing is making a significant impact is in medical imaging. AI algorithms can enhance the quality of imaging data, enable the reconstruction of high-resolution images from low-quality inputs, and automate the detection of abnormalities. For instance, NVIDIA's AI models can create synthetic medical images for training purposes, helping to overcome the challenges of data privacy and availability.
Here are some examples of how accelerated computing is being used in healthcare:
These solutions are being developed and deployed by leading organizations in the field, including NVIDIA, Amgen, and the University of Wisconsin-Madison. By harnessing the power of accelerated computing, we can unlock new breakthroughs and improve patient outcomes.
Clinical and Research Applications
GenAI is a game-changer in medical research, allowing researchers to generate synthetic data that mirrors real patient data. This capability is invaluable for research areas where data may be scarce or difficult to obtain, such as rare diseases.
Synthetic data can be used to simulate clinical trials, test hypotheses, and model disease progression without the ethical and privacy concerns associated with using actual patient data. This accelerates research and reduces costs.
The company provides tools for generating synthetic datasets that mimic real patient data, enabling researchers to conduct studies without ethical or privacy concerns. This accelerates medical research and facilitates the exploration of new treatments and therapies.
GenAI can also be used to analyze complex and diverse information sets, making it a suitable option for identifying a patient's potential health risks. It acts as a virtual collaborator, helping healthcare providers consider a broader spectrum of variables.
GenAI doesn't just assist with diagnosis; it also plays a crucial role in suggesting tailored treatment options. By drawing on vast databases of medical knowledge and the latest research, this technology can propose evidence-based therapies and interventions.
The Netherlands Cancer Institute used NVIDIA and VMware's AI solution to deploy AI workloads on virtualized servers, improving performance, scalability, and efficiency. This is just one example of how GenAI can be used to drive medical breakthroughs.
GenAI can create synthetic medical images for training and research, enhancing the skills of medical professionals without compromising patient privacy.
Expand your knowledge: Ethical Implications of Ai in Healthcare
Frequently Asked Questions
How does GenAI work?
GenAI works by analyzing patterns in large datasets to generate original and contextually relevant content. This process enables GenAI to produce diverse and seemingly new outputs in response to user prompts.
What is the difference between GenAI and AI?
Traditional AI excels in analysis and automation, while Gen AI specializes in creative content generation. This difference sets the stage for their distinct roles in industry transformations.
What is generative AI in medical billing?
Generative AI in medical billing uses machine learning to automatically identify and record billable services from clinical documentation, reducing errors and reliance on human coders. This technology improves charge capture accuracy by analyzing treatment records and suggesting accurate billing codes.
Sources
- https://www.johnsnowlabs.com/generative-ai-healthcare/
- https://www.nvidia.com/en-us/industries/healthcare-life-sciences/
- https://cloud.google.com/blog/topics/healthcare-life-sciences/google-cloud-gen-ai-healthcare-announcements
- https://www.alpha-sense.com/blog/trends/generative-ai-healthcare/
- https://www.mckinsey.com/industries/healthcare/our-insights/tackling-healthcares-biggest-burdens-with-generative-ai
Featured Images: pexels.com