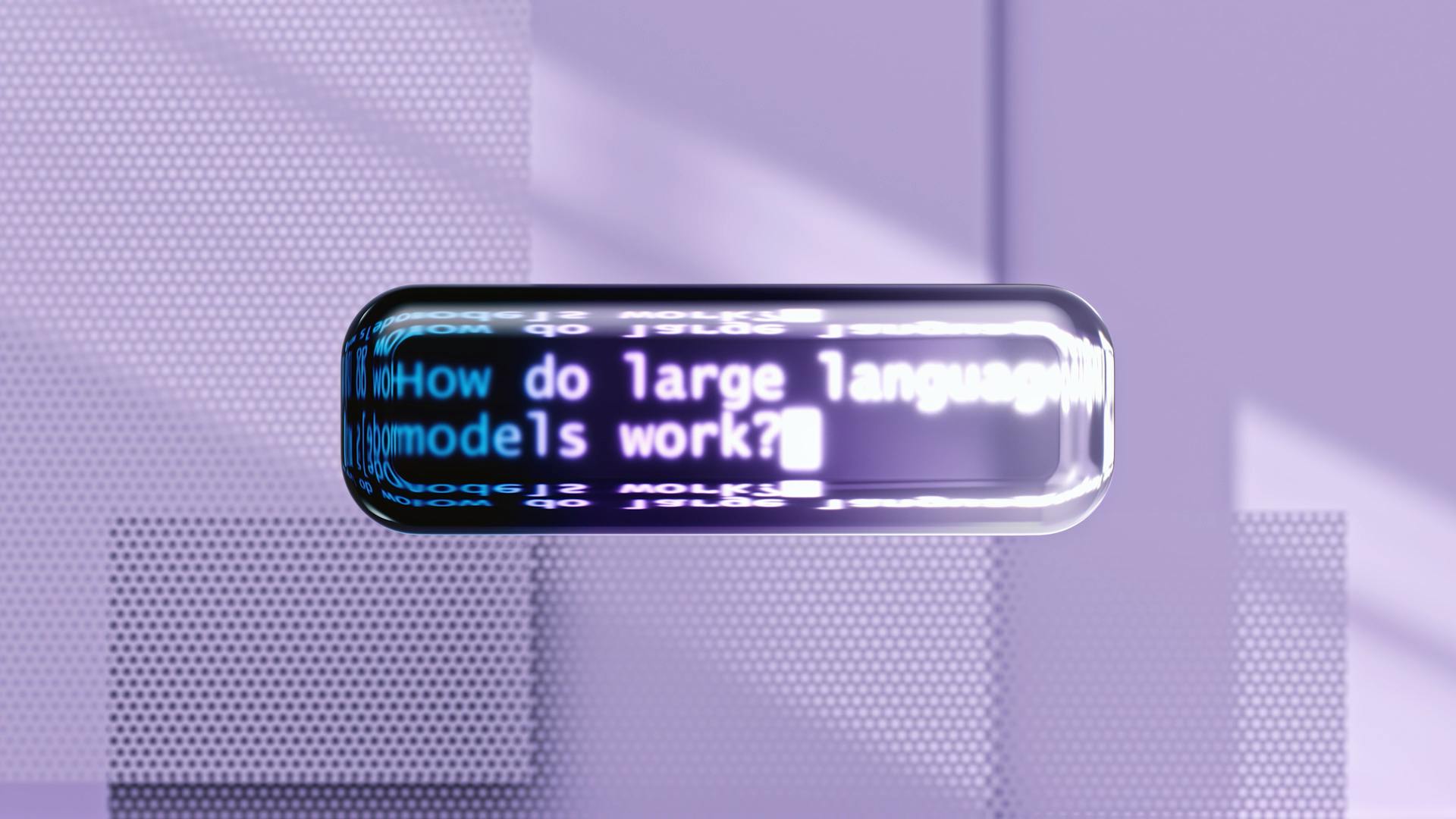
Generative AI is a type of AI that can create new content, such as images, music, or text, based on a given prompt.
These models are trained on vast amounts of data, allowing them to learn patterns and relationships that enable them to generate new content that's often surprisingly realistic.
One key application of generative AI is in the field of art, where it can be used to create new images, paintings, or even entire albums of music.
But generative AI is not just about creating art; it also has practical applications, such as generating new product designs or even entire product lines.
For your interest: Generative Ai Human Creativity and Art
What is Generative AI
Generative AI is a type of machine learning approach that aims to discover a decision boundary by dividing the data space into classes. It seeks to understand how data is generated or embedded into the space.
Generative AI models follow training phases under restricted parameters, challenging the model to make conclusions based on the vital attributes of the training data. This allows them to learn the underlying patterns and features of the training data.
Related reading: How Is Generative Ai Trained
A generative AI system encompasses several key components, including input data, preprocessing modules, feature extraction layers, neural networks, and post-processing modules. These components work together to refine the results generated by the system.
Here are some of the key components of a generative AI system:
- Input data – It ensures the kind of task the AI system should perform
- Preprocessing modules – It ensures cleaning up any noise in the input
- Feature extraction layers – Extracts vital features from raw inputs
- Neural networks – Processes the data inputs extracted by feature extraction layers
- Post-processing modules – Refines the results
What is Generative AI?
Generative AI is a type of machine learning approach that aims to discover a decision boundary by dividing the data space into classes. It seeks to clarify the manner of data generation or how the data is embedded into the space.
Generative AI models follow training phases under restricted parameters, challenging the model to make conclusions based on the vital attributes of the training data. They learn to recognize the underlying patterns and features of the training data, enabling them to generate new content with lifelike simulations.
A generative AI system encompasses several key components, including input data, preprocessing modules, feature extraction layers, neural networks, and post-processing modules. These components work together to ensure that the AI system can perform efficiently.
Some of the key components of a generative AI system include:
- Input data – It ensures the kind of task the AI system should perform
- Preprocessing modules – It ensures cleaning up any noise in the input
- Feature extraction layers – Extracts vital features from raw inputs
- Neural networks – Processes the data inputs extracted by feature extraction layers
- Post-processing modules – Refines the results these components generate
Generative AI has numerous practical use cases across various domains, including image synthesis and editing, text generation and natural language processing, music composition, and more.
What Is ChatGPT?
ChatGPT is an AI chatbot developed by OpenAI. It's a great example of how generative AI can be used for personal and professional work.
ChatGPT is based on GPT-4, a large language model using transformer architecture. This architecture is specifically designed to understand and generate human-like text.
ChatGPT popularized the use of generative AI, making it more accessible and widely adopted.
You might like: Generative Ai Architecture Design
Benefits and Applications
Generative AI can automate tasks, freeing up time and resources for more important objectives. According to the State of IT report, 86% of IT leaders expect Generative AI to play a significant role in their organizations soon.
Generative AI can help businesses improve their digital marketing campaigns by generating SEO-friendly and high-quality content. This is especially useful for companies looking to innovate their marketing strategies.
For your interest: Generative Ai in Marketing
By automating content generation, businesses can increase efficiency and productivity. For example, generative AI can generate news articles, financial data, summaries, and other types of content quickly and accurately.
Generative AI can also help predict potential failures and schedule maintenance by analyzing device data. This can result in significant cost savings and improved operational efficiency.
The application areas of generative AI are diverse and far-reaching. Some examples include:
- Automating customer support and quality assurance
- Generating personalized recommendations based on customer behavior
- Screening candidate profiles and conducting performance evaluations
- Creating new designs, logos, and musical notes
- Generating high-quality content for marketing and SEO purposes
By leveraging generative AI, businesses can improve their bottom line and stay ahead of the competition.
How it Works
Generative AI models learn the underlying patterns and structures within a training dataset, allowing them to generate new data that resembles the original.
These models often utilize probabilistic modeling to capture the distribution of the training data, and some, like Generative Adversarial Networks (GANs), employ adversarial training to create increasingly realistic samples.
GANs consist of two competing neural networks: the generator and the discriminator, which work together in a competition to create realistic data and distinguish between real and fake data.
See what others are reading: Generative Adversarial Networks Ai
The generator takes a random input and creates new data, while the discriminator evaluates the content to determine if it is real or fake. Through this process, the generator learns to create more realistic data, and the discriminator becomes better at distinguishing between real and fake data.
Here are some common working principles of generative models:
- Probabilistic Modeling: capturing the distribution of the training data
- Latent Space Representation: learning a lower-dimensional representation of the training data
- Adversarial Training: GANs employ a unique working principle to create realistic samples
- Autoregressive Modeling: modeling the conditional probability of each element in a sequence
- Reconstruction and Error Minimization: focusing on reconstructing the original input data
Decision-Making and Problem-Solving
Gen AI models are designed to aid in decision-making processes by producing a wide range of potential solutions or scenarios. This allows decision-makers to factor in a variety of options and make more informed choices.
One of the key benefits of Gen AI models is that they help to enhance decision-making and problem-solving. They can produce a wide range of potential solutions or scenarios, making it easier to identify the best course of action.
By considering multiple options, decision-makers can make more informed choices and avoid potential pitfalls. This is particularly useful in complex or high-stakes situations where the outcome is uncertain.
Gen AI models can also help to identify potential problems or areas of risk, allowing decision-makers to take proactive steps to mitigate them.
Here's an interesting read: The Economic Potential of Generative Ai the Next Productivity Frontier
Chatbots
Chatbots have a multitude of applications, ranging from GenAI in eCommerce to customer service, and are extremely helpful in offering buyers relevant information about products.
They enable buyers to make a purchase decision faster and provide after-sales support smoothly. Chatbots can be programmed to answer customer inquiries naturally and perfectly, improving customer satisfaction and creating brand loyalty to a great extent.
The key to chatbots' success lies in their ability to process and analyze natural language, thanks to the power of NLP. This allows them to understand and extract meaning from user input and generate natural language responses accordingly.
With the help of Generative AI, chatbots can be trained on large datasets of real-world examples, enabling them to learn from this training data and generate new content similar in style and structure to the original data.
You might enjoy: Can I Generate Code Using Generative Ai
How
Generative AI models use a technique called competition to train their neural networks. The generator and discriminator networks are trained together in a competition, where the generator tries to create data that can fool the discriminator into thinking it is real.
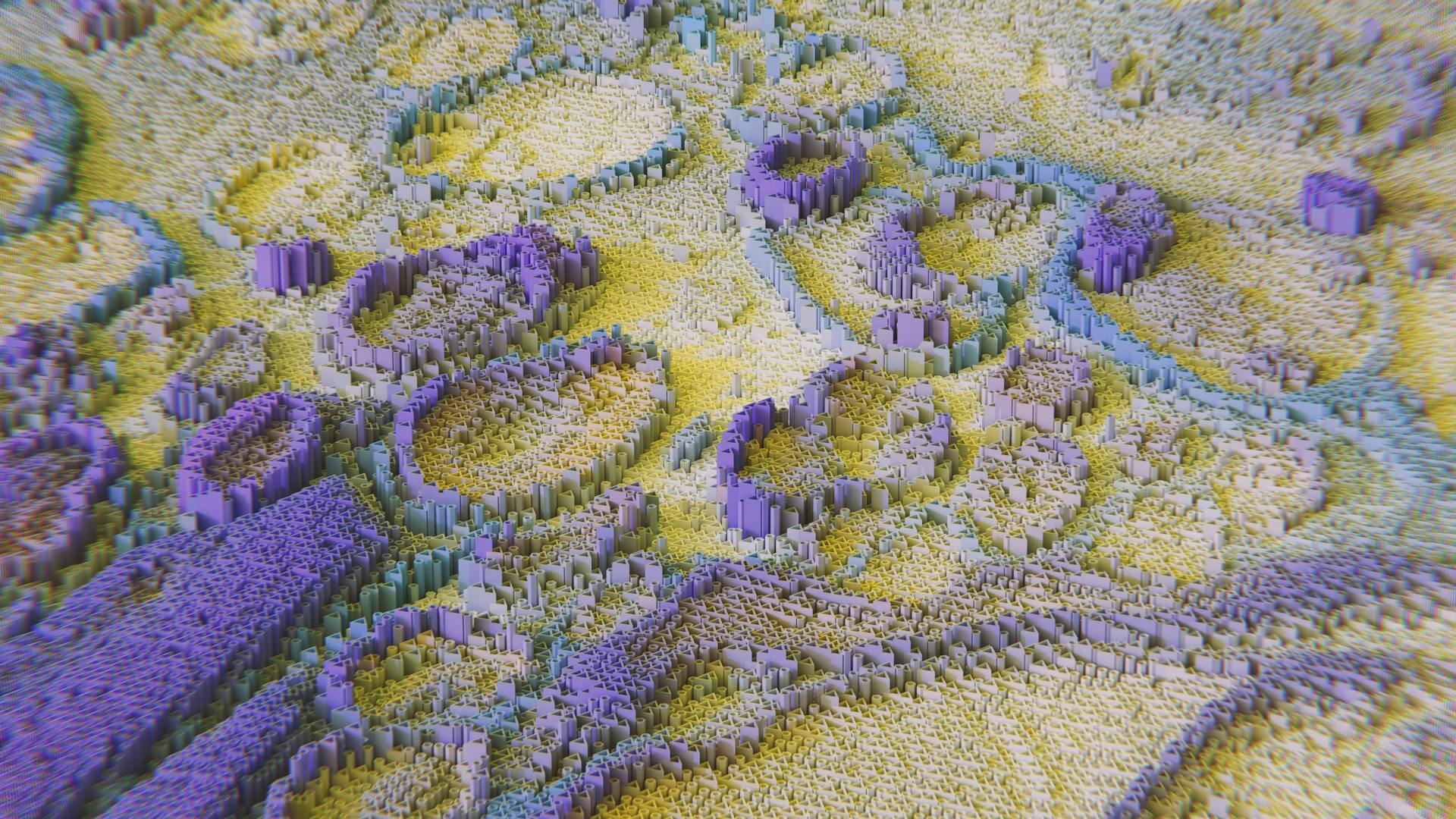
The generator produces new data, such as images or audio, from a random input. Initially, the generated data is random and meaningless.
The discriminator receives both real and generated data and learns to differentiate between them. Through this process, the generator learns to create more realistic data, and the discriminator becomes better at distinguishing between real and fake data.
Autoencoders are another type of generative AI model that compresses and reconstructs input data. They learn a compressed representation of the input data that can then be used to generate new, similar data.
Autoencoders can be used for image and audio compression, and they can generate highly realistic images and videos. Researchers have used autoencoders to create deepfake videos, which are videos that utilize AI face swaps to replace one person's face with another.
Generative AI models use natural language processing algorithms and huge language models to automate content generation. They are trained on massive datasets containing existing content examples.
Expand your knowledge: Getty Images Nvidia Generative Ai Istock
How It Differs
Generative AI differs from other types of AI in its output, focusing on creating new and original content that doesn't exist in the training data.
It stands out from supervised and unsupervised learning, which are focused on learning patterns and making predictions based on existing data.
Generative AI uses unsupervised learning techniques to learn patterns and relationships in data, but its focus is on creating new content rather than making predictions or classifications.
Discriminative modeling, on the other hand, is used for classifying existing data points, whereas generative modeling tries to understand the dataset structure and generate similar examples.
Generative AI models can generate new content based on their input, such as text or images, and can even create realistic images or stories.
This technology has applications in speech synthesis, where it's used to convert written text into synthesized speech, offering natural-sounding vocal interactions.
Generative AI models can also be used in text-to-speech systems and voice assistants, providing users with hands-free access to information and control over their environments.
A fresh viewpoint: Generative Ai Content
How Do Gans?
A Generative Adversarial Network (GAN) is a type of generative model that consists of two neural networks: the generator and the discriminator. The generator produces new data, while the discriminator verifies whether the generated data is real or fake.
The generator takes a random input and creates new data, such as images or audio. Initially, the generated data is random and meaningless. The discriminator then receives both real and generated data and learns to differentiate between them.
The two networks are trained together in a competition: the generator tries to create data that can fool the discriminator into thinking it is real, while the discriminator tries to identify which data is real and which is generated correctly. Through this process, the generator learns to create more realistic data, and the discriminator becomes better at distinguishing between real and fake data.
GANs are often used for tasks such as image and video generation, as well as music generation. They have been used to generate realistic images, videos, and even music.
Discover more: Generative Ai Synthetic Data
Here are some key characteristics of GANs:
- Generator: Creates new data based on a random input
- Discriminator: Verifies whether the generated data is real or fake
- Training: The two networks are trained together in a competition
- Output: The generator learns to create more realistic data, and the discriminator becomes better at distinguishing between real and fake data
GANs have many applications, including:
- Image generation: Generating realistic images from scratch
- Video generation: Generating realistic videos from scratch
- Music generation: Generating music from scratch
Overall, GANs are a powerful tool for generating new and realistic data, and have many applications in fields such as computer vision, natural language processing, and music generation.
Preprocessing
Before data is fed into a generative AI model, it needs to be preprocessed to make it easier for the model to understand.
This can involve converting the data into a format that the model can work with, such as converting images into pixels or text into numerical vectors.
Types and Techniques
Generative AI uses two distinct techniques: Generative Adversarial Networks (GANs) and Variational Autoencoders (VAEs). These techniques are used to generate new content that resembles real data.
Generative Adversarial Networks (GANs) consist of a generator and a discriminator network that compete against each other. The generator creates synthetic samples, while the discriminator tries to distinguish between real and generated samples.
A unique perspective: Generative Ai in Real Estate
Variational Autoencoders (VAEs) learn a compressed representation of the input data called the latent space. They consist of an encoder that maps the data to the latent space and a decoder that reconstructs the data from the latent space.
There are various types of generative AI models, including Generative Adversarial Networks (GANs), Variational Autoencoders (VAEs), Autoregressive Models, Flow-based Models, Restricted Boltzmann Machines (RBMs), and PixelCNN.
Autoregressive models model the conditional probability of each element in a sequence given the previous elements. They generate new data by sequentially predicting the next element based on the previous ones.
Flow-based models learn an invertible transformation from a simple probability distribution to a complex data distribution. By sampling from the simple distribution and applying the inverse transformation, flow-based models generate samples that match the complex data distribution.
To get the most out of generative AI, it's essential to learn how to prompt effectively. This involves modifying prompts to get better results, which requires some practice.
Generative AI models can be broadly categorized into the following types: Generative Adversarial Networks (GANs), Variational Autoencoders (VAEs), Autoregressive Models, Flow-based Models, Restricted Boltzmann Machines (RBMs), and PixelCNN.
Here's an interesting read: What Are the Two Main Types of Generative Ai Models
Applications Across Industries
Generative AI is revolutionizing various industries with its vast range of applications. Generative AI can transform many industries by providing new opportunities for innovation and growth, including healthcare where it can efficiently convert X-rays and CT scans into more realistic images, allowing clinicians to use the images for diagnosis purposes.
Generative AI is being used in the banking and finance industry to analyze huge financial data sets, gain deeper insights into consumer behavior, and recognize potential risks and fraudulent activities. It can also be used in film and game design, music composition, and personalized content recommendations in the media and entertainment industry.
Some of the most promising applications of generative AI across industries include:
- Healthcare: Efficiently converting X-rays and CT scans into more realistic images for diagnosis purposes.
- Banking and finance: Analyzing financial data to gain deeper insights into consumer behavior and recognize potential risks and fraudulent activities.
- Media and entertainment: Creating personalized content recommendations, generating new music or game levels, and enhancing film and game design.
Healthcare
In healthcare, generative AI is a game-changer. The technology can efficiently convert X-rays and CT scans into more realistic images.
This allows clinicians to use the images for diagnosis purposes, which can lead to more accurate diagnoses and better patient outcomes.
Generative AI can also be used for sketches-to-photo translation, giving doctors detailed views of a patient's internal body.
This can aid in early detection of harmful diseases, potentially saving lives.
Explore further: Generative Ai by Getty Images
Finance
In the finance industry, generative AI is a game-changer. By analyzing huge financial data sets, financial organizations can gain deeper insights into consumer behavior and recognize potential risks and fraudulent activities.
Financial institutions can use generative AI to detect patterns that indicate fraudulent activity or identify potential risks in a portfolio or account. This capability helps prevent potential threats and improves consumer trust by ensuring the safety and security of their monetary transactions.
Generative AI can also help financial institutions optimize their investment portfolios, maximizing returns while minimizing risk.
Worth a look: Generative Ai Use Cases in Financial Services
Types of Applications
Generative AI has a plethora of practical applications in different domains, such as computer vision, where it can enhance the data augmentation technique.
Generative AI models can be used for various tasks, including text and image generation. For instance, Google's Gemini chatbot can answer questions and generate text from prompts, while OpenAI's DALL-E 3 can create images and artwork from text prompts.
Explore further: Generative Ai Text Analysis
Text and image generation models are arguably the most well-known types of generative AI applications. Google and Meta have demonstrated photorealistic image generators, although these are not publicly available as of October 2024.
Generative AI models typically rely on a user feeding a prompt into the engine, which then guides it towards producing some sort of desired output, such as text, images, videos, or music.
Here are some examples of generative AI models and products:
Applications Across Industries
Generative AI is transforming various industries by providing new opportunities for innovation and growth. Generative AI can generate highly realistic images that resemble photographs or artistic styles, making it a valuable tool in the art and design industry.
In the finance and insurance industry, generative AI can help customers manage their finances better through a financial wellness program. This technology can analyze huge financial data sets, gain deeper insights into consumer behavior, and recognize potential risks and fraudulent activities.
On a similar theme: Impact of Generative Ai on Tax Industry
Generative AI is also being used in the media and entertainment industry to create personalized content recommendations. By analyzing data on customer preferences, generative AI can help create more engaging and personalized content, such as recommending movies and TV shows based on a user's viewing history.
In the manufacturing industry, generative AI can be used in product design, process optimization, and predictive maintenance. By analyzing data on product performance, generative AI can help manufacturers optimize product design, improving functionality and reducing costs.
Here are some notable examples of generative AI applications across various industries:
Generative AI can also be used in healthcare to convert X-rays and CT scans into more realistic images, allowing clinicians to use the images for diagnosis purposes. Additionally, generative AI can be used in music composition, enabling the creation of original compositions or accompaniments.
Worth a look: Chatgpt Openai Generative Ai Chatbot Can Be Used for
Opportunities and Advancements
Generative AI is poised to revolutionize content creation in the art and marketing industries, as well as in the fields of pharmaceuticals and biotech.
Computers will be creatively more powerful to generate compelling content, which will accelerate the pace of drug discovery by mitigating time and managing resource allocation for identifying promising compounds.
Generative AI will ensure its prominence in MedTech as a catalyst to create personalized treatment plans for patients based on the learned patterns of generative AI models using huge data of patients associated with their medical history, symptoms, and other factors.
The technology will pave the path for tailored medical assistance to speed up the recovery process of patients, based on the data-backed insights provided by the AI tools.
Generative AI will play a central role in various industries, including content creation, software development, medicine, productivity, business transformation, and much more.
The capabilities of generative AI have already proven valuable in these areas, and as the technology continues to evolve, so too will generative AI’s applications and use cases.
Generative AI companies are pushing the envelope by creating higher-parameter models, photorealistic AI video, and incorporating AI closely into enterprise software.
One potential change generative AI might bring to computing is the use of natural language commands to both find information and command the system.
Recommended read: Generative Ai Content Creation
Generative AI could also play a critical role in scientific research, enabling researchers to simulate complex phenomena and generate new hypotheses rapidly.
With the ability to create vast amounts of data quickly, generative AI could accelerate scientific breakthroughs in fields like medicine, physics, and engineering.
Generative AI is rapidly evolving, and its future promises even greater impact across industries, with more sophisticated models that can generate highly realistic content, from lifelike images and videos to coherent text.
Systems will be able to seamlessly generate content that blends different formats, such as text, images, and audio, offering richer and more immersive experiences.
The integration of generative AI into everyday applications will become more common, making it an integral part of both personal and professional life.
A unique perspective: Telltale Words Identify Generative Ai Text
Key Concepts and Techniques
Generative AI uses two distinct techniques: Generative Adversarial Networks (GANs) and Variational Autoencoders (VAEs). These techniques are the foundation of generative AI.
Generative models, such as GANs, VAEs, and Transformer-based models, learn data patterns and generate new outputs. They're like super-smart pattern recognizers that can create new content based on what they've learned.
See what others are reading: New Generative Ai
Neural networks, like recurrent neural networks (RNNs) and convolutional neural networks (CNNs), are used in generative AI to understand and generate data. These networks are like complex webs of interconnected nodes that process and learn from data.
Training generative AI models requires large datasets, like vast amounts of text data for text-generating models. The more data, the better the model can learn and generate new content.
The latent space is a lower-dimensional representation of data where generative models manipulate patterns to create variations of the original content. It's like a secret recipe book that helps models create new and interesting content.
Expand your knowledge: Google Announces New Generative Ai Search Capabilities for Doctors
What Drives AI
Generative artificial intelligence is driven by natural language processing (NLP), a subfield of AI that enables computers to understand text and spoken words like humans.
NLP is the core competency behind generative AI's rapid evolution, allowing it to generate creative content and inventive works.
Generative AI's ability to generate new text and speech data, such as news articles and synthetic voices, is made possible by NLP.
NLP's proficiency in understanding text and spoken words is key to generative AI's growing capabilities.
Readers also liked: Is Speech to Text Generative Ai
NLP, Deep Learning, and Machine Learning
NLP, deep learning, and machine learning are the key components of generative AI, each playing different but complementary roles in generating new content. NLP is particularly important for generative AI that deals with natural language, as it involves the processing and analyzing of textual data.
Deep learning is a type of machine learning that uses artificial neural networks to analyze and learn patterns in data, making it well-suited for generating complex, high-dimensional data like images, music, and video. This is critical in applications such as chatbots, where the AI must be able to generate natural language responses based on user input.
Machine learning is the foundation of all generative AI, providing the algorithms and techniques necessary to train models on large datasets of real-world examples. These models learn from this training data, allowing them to generate new content similar in style and structure to the original data.
Generative AI models typically use deep learning architectures such as recurrent neural networks (RNNs), convolutional neural networks (CNNs), and transformers to understand and generate data. This is why deep learning is essential for generating complex data like images and music.
Training data is also crucial for generative AI models, which require large datasets to learn patterns and structures. For example, training a text-generating model involves feeding it vast amounts of text data.
Suggestion: Neural Network vs Generative Ai
AI Technologies and Models
Generative AI is a single technology that powers endless possibilities, but it's made up of different AI models that work together to achieve various operations.
The most common type of generative AI model is a Generative Adversarial Network, or GAN for short.
GANs consist of two neural networks: a generator and a discriminator, which work together in a competition-like setup.
The generator's job is to create realistic outputs that mimic the input data, while the discriminator's job is to identify whether the output is real or fake.
The GAN framework was first proposed in 2014 by Ian Goodfellow and his colleagues at the University of Montreal.
The generator network is trained to create new samples similar to the training data, while the discriminator network is trained to distinguish between real and fake data.
This competition between the generator and the discriminator allows the generator to create realistic outputs that fool the discriminator.
GANs have been used for various applications, including image and video generation, text generation, and music composition.
The ability of GANs to create realistic and diverse outputs has made them one of the most exciting AI research areas.
You might enjoy: Generative Ai Fake News
Getting Started
Getting Started is the most exciting part of learning about Generative AI. Begin with Curiosity, as it's the best way to understand the potential and limits of GenAI solution.
To start, experiment with different prompts and explore different results. See how slight changes in your input can result in varying outcomes.
Don't be afraid to try new things and make mistakes. It's a normal part of the learning process, and it's the correct way to understand the GenAI solution.
Start with a Generative AI Tutorial, which can provide a comprehensive guide to getting started. Lesson 1 of 14 is a great place to begin.
Suggestion: How to Get Started with Generative Ai
Sources
Featured Images: pexels.com