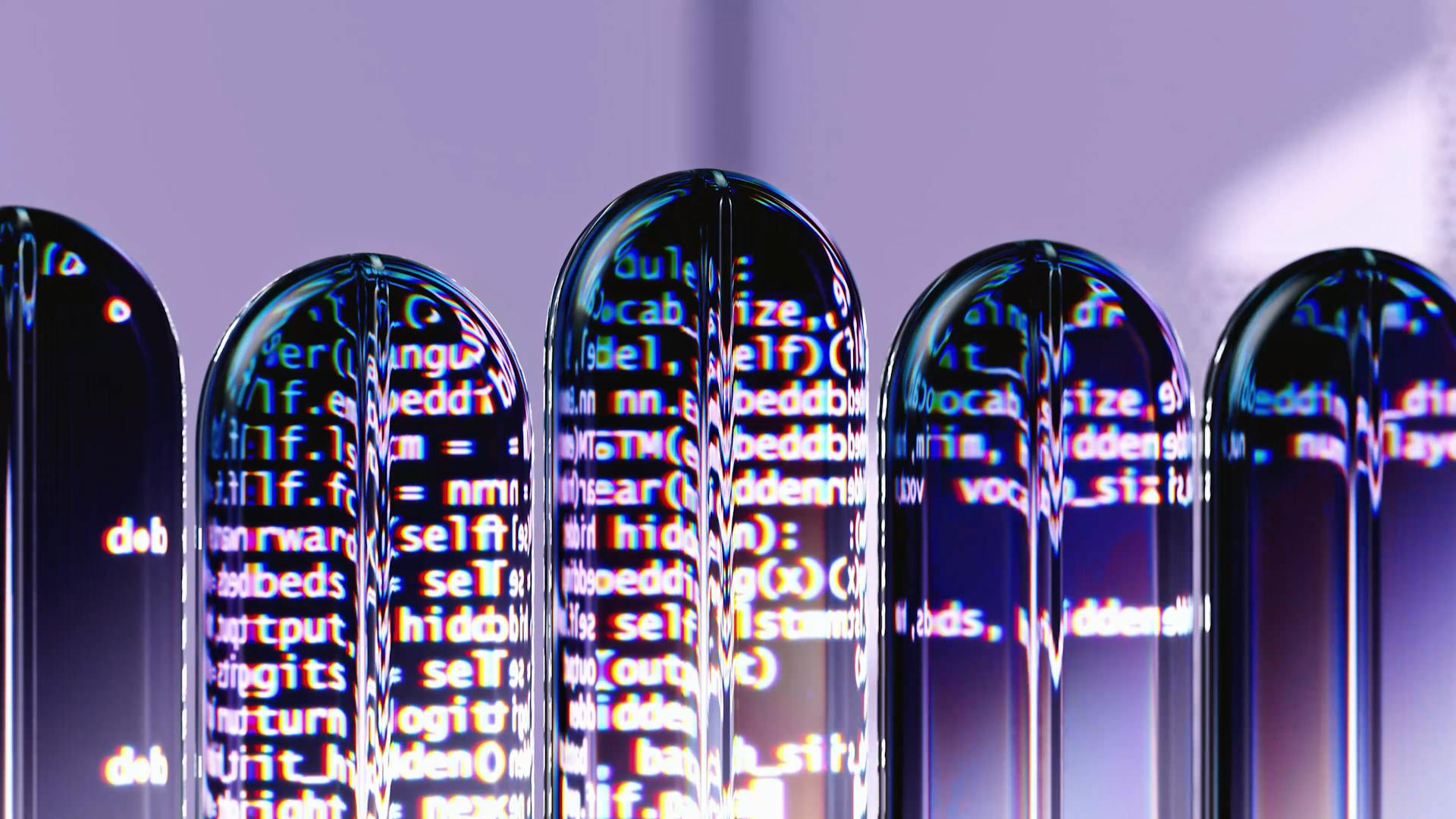
Generative AI in pharma is revolutionizing the way drugs are developed and approved. According to a study, generative AI can reduce the time it takes to develop a new drug from 10-15 years to just 5-7 years.
One of the key benefits of generative AI in pharma is its ability to generate new molecular structures that have never been seen before. This is made possible by the vast computational power of AI algorithms, which can analyze vast amounts of data and identify patterns that humans might miss.
In fact, researchers have used generative AI to design new compounds that have shown promising results in clinical trials. For example, a team of scientists used a generative AI model to design a new compound that was able to target a specific disease-causing protein with high accuracy.
Related reading: How Multimodal Used in Generative Ai
What is Generative AI in Pharma?
Generative AI in pharma is a game-changer in the field of drug discovery and development. It utilizes artificial intelligence to design new drug molecules, predict their efficacy, and optimize their chemical structures with unprecedented speed and precision.
Generative AI drugs can do in minutes what traditional methods take months or years to accomplish. This is a significant breakthrough in the pharmaceutical industry.
One of the key benefits of generative AI in pharma is the ability to create personalized treatment plans for patients.
Curious to learn more? Check out: Role of Generative Ai in Drug Discovery
Benefits and Impact
Generative AI in pharma has the potential to revolutionize the industry, and its benefits are already being felt in real-world applications.
Developing a new drug can take over a decade and cost around USD 2.6 billion, but generative AI can reduce this timeline to just a few years and lower costs significantly.
Generative AI accelerates the drug development process by rapidly generating novel compounds and optimizing existing ones, making it a game-changer for the pharmaceutical industry.
This technology can reduce the need for extensive lab work and minimize failed experiments, allowing resources to be allocated more effectively.
Generative AI uses advanced algorithms to design drug molecules with high precision, ensuring that the compounds created possess the intended properties.
This precision minimizes the chances of adverse effects and improves the chances of success in clinical trials.
Integrating generative AI in drug discovery leads to higher success rates by accurately predicting molecular behavior and biological interactions.
If this caught your attention, see: Generative Ai in Tourism
By analyzing individual patient data, such as genetic profiles, generative AI facilitates the development of personalized treatments that are more effective and have fewer side effects.
Virtual screening powered by generative AI allows researchers to quickly analyze how millions of compounds interact with specific biological targets.
This method speeds up the identification of viable drug candidates and bypasses the need for time-consuming and costly physical testing.
Generative AI excels in drug repurposing by analyzing existing medications and identifying new therapeutic uses, saving time and money and providing new treatment options for diseases that previously had limited or no effective therapies.
Several companies, such as Insilico Medicine, Exscientia, and Freenome, are already experiencing real-world benefits from using generative AI in drug discovery and diagnostics.
Applications in R&D
Generative AI applications in R&D are revolutionizing the entire drug development lifecycle. Generative AI can optimize drug discovery, formulation, and repurposing, as well as experiment tracking and record-keeping.
A virtual assistant fueled by Generative AI for pharmaceutical drug R&D promises a wealth of transformative benefits for R&D teams, empowering researchers to maintain real-time access to the latest clinical trial data.
Generative AI can accelerate the drug discovery timeline, enhance drug efficacy and safety, and reduce costs by streamlining the discovery process. By leveraging GANs, reinforcement learning, and NLP, researchers can identify and optimize drug candidates more efficiently.
Here are some key applications of Generative AI in R&D:
Generative AI's potential in formulation optimization includes opportunities for solubility prediction, excipient selection, dosage form design, process enhancement, polymer design for controlled-release formulations, and stability prediction.
A unique perspective: Generative Ai in Graphic Design
What Is the Role of?
In the realm of R&D, generative AI is revolutionizing the way we approach drug discovery. Generative AI's role in drug discovery is game-changing, offering a powerful toolset for AI in pharma and biotech sectors.
By leveraging GANs, reinforcement learning, and NLP, researchers can accelerate the drug discovery timeline. This means that AI can reduce the time needed to identify and optimize drug candidates.
AI models can also enhance drug efficacy and safety by predicting and refining molecular structures to meet therapeutic goals. This is a significant advantage in the field of drug discovery.
One of the most significant benefits of generative AI in drug discovery is that it can reduce costs. By streamlining the discovery process, AI can significantly lower R&D expenses.
Here are some key benefits of generative AI in drug discovery:
- Accelerate the Drug Discovery Timeline
- Enhance Drug Efficacy and Safety
- Reduce Costs
How Works
Generative AI is revolutionizing the drug discovery process, and its impact is being felt across the pharmaceutical and biotech sectors. It leverages machine learning models, particularly generative adversarial networks (GANs) and transformer models, to create new drug candidates.
These AI models are trained on vast chemical compounds and biological data datasets, enabling them to generate novel molecules that meet specific therapeutic criteria. This is a game-changer for researchers, who can now accelerate the drug discovery timeline and reduce costs.
Generative AI can design new molecules by predicting their structure, which might not be easily discovered through conventional methods. It can also optimize existing compounds by suggesting modifications to enhance their efficacy, reduce side effects, and improve their drug-like properties.
Here are some key benefits of using generative AI in drug discovery:
- Accelerates the drug discovery timeline
- Enhances drug efficacy and safety
- Reduces costs
Generative AI can also predict biological activity, forecasting how a new drug will interact with biological targets. This helps to prioritize the most promising candidates for further development.
In addition to these benefits, generative AI can also generate novel chemical compounds for disease targets, exploring vast chemical spaces to propose unique molecular structures for further investigation. It can also predict the binding affinity of generated compounds to target proteins using molecular docking simulation, prioritizing compounds with desired biological activity.
Applications in R&D
Generative AI is revolutionizing the entire drug R&D and discovery lifecycle with many powerful applications. It's helping to optimize drug discovery, formulation, and repurposing, making it easier to identify and optimize drug candidates.
Generative AI can accelerate the drug discovery timeline by reducing the time needed to identify and optimize drug candidates. This is achieved through the use of machine learning models, particularly generative adversarial networks (GANs) and transformer models, to create new drug candidates.
A fresh viewpoint: Telltale Words Identify Generative Ai Text
A virtual assistant fueled by Generative AI for pharmaceutical drug R&D promises a wealth of transformative benefits for R&D teams. It empowers researchers to maintain real-time access to the latest clinical trial data, facilitating swift and informed actions that ensure the safety and efficacy of ongoing trials.
Generative AI's role in drug discovery is game-changing, offering a powerful toolset for AI in pharma and biotech sectors. By leveraging GANs, reinforcement learning, and NLP, researchers can accelerate the drug discovery timeline, enhance drug efficacy and safety, and reduce costs.
Generative AI can design new molecules, optimize existing compounds, and predict biological activity. It can create new drug candidates by predicting the components in a molecule's structure, which might not be easily discovered through conventional methods.
Here are some of the key applications of Generative AI in R&D:
* ApplicationDescriptionDrug discoveryGenerative AI can optimize drug discovery, formulation, and repurposing.Experiment tracking and record-keepingGenerative AI can help researchers maintain real-time access to the latest clinical trial data.R&D trial tracking & smart materials/chemical searchGenerative AI can empower researchers to conduct intelligent and highly efficient searches for materials and chemicals.Clinical trial feedback summarizationGenerative AI can help researchers summarize clinical trial feedback and make informed decisions.
Generative AI's potential in formulation optimization includes opportunities for solubility prediction, excipient selection, dosage form design, process enhancement, polymer design for controlled-release formulations, and stability prediction.
Curious to learn more? Check out: Generative Ai for Design
Optimizing Clinical Trials
Generative AI can significantly improve the success rate of clinical trials by analyzing vast amounts of data, including medical literature, patient data, and hypotheses. This can help pharmaceutical companies estimate costs, risks, and probable results before proceeding with clinical trials.
By leveraging generative AI, companies can assemble more diverse and representative clinical-trial populations, which can lead to a 10 percent increase in the possibility of success (PoS) for trials. This, in turn, can result in a 20 percent reduction in trial costs and duration, and accelerate time to approval by one to two years.
Here are some key benefits of using generative AI in clinical trials:
- About a 10 percent increase in the possibility of success (PoS) for trials
- About a 20 percent reduction in trial costs and duration
- Time to approval accelerated by one to two years
- Potential double-digit impact on the net present value (NPV) of assets or portfolios
Experiment Tracking and Record-Keeping
Experiment tracking and record-keeping is a crucial aspect of clinical trials. Pharmaceutical companies can be dealing with tonnes of information related to 100-150 ongoing drug discovery experiments at any given time.
Generative AI can help automate experiment tracking by generating summaries or reports of these experiments using text and image-based models. This can help medical researchers keep better records and track progress more efficiently.
Optimizing Clinical Trials
Optimizing clinical trials is crucial for the success of pharmaceutical research. Generative AI can influence the trial's success by analyzing medical literature, patient data, hypotheses, and other relevant information researchers may overlook.
Pharmaceutical companies can use AI predictions to estimate costs, risks, and probable results before proceeding with clinical trials. This helps them make informed decisions and avoid costly mistakes.
Clinical trials require careful planning as they impact the candidate's safety and drug research outcome. Generative AI can help streamline this process by automating data analysis, identifying patterns, and providing insights that human researchers might miss.
For instance, precision oncology researchers use biomarkers to stratify patients according to their probability of progression or to predict their response to different treatments. This approach can help assemble more diverse and representative clinical-trial populations, leading to a 10 percent increase in the possibility of success (PoS) for trials.
By using gen AI models built on data from medical-imaging techniques such as X-rays, CAT scans, and MRIs, scientists could even identify entirely new biomarkers: deeply hidden visual signatures of disease activity and severity that lead to unforeseen new treatments. This can accelerate time to approval by one to two years, resulting in a potential double-digit impact on the net present value (NPV) of assets or portfolios.
Consider reading: Generative Ai Environmental Impact
Here are some benefits of using generative AI in clinical trials:
• Approximately 20 percent cost efficiency improvement
• 10 to 20 percent faster enrollment
• About a 10 percent increase in the possibility of success (PoS) for trials
• About a 20 percent reduction in trial cost and duration
These benefits can be achieved by leveraging generative AI's ability to analyze vast amounts of data, identify patterns, and provide insights that human researchers might miss. By using AI-powered tools, pharmaceutical companies can optimize their clinical trials, reduce costs, and accelerate time to market.
Additional reading: What Are the Benefits of Generative Ai
Data Management and Security
Data management in pharmaceuticals is a labor-intensive process that can be automated with generative AI, resulting in a 30 percent-plus cost savings and a 50 percent-plus reduction in time to database lock.
Automating data management allows for databases to be created with one click, case report forms to be auto-generated, and data to be reviewed and cleaned in real time.
However, handling sensitive patient data and proprietary research information raises concerns about data security and privacy. Data security is paramount to prevent data breaches and privacy violations.
Robust cybersecurity measures, such as encryption, access controls, and regular security audits, are essential to safeguard patient data and proprietary research findings.
Consider reading: Generative Ai for Product Management
Data Security
Data security is crucial when handling sensitive patient data and proprietary research information. Protecting patient data during generative AI-driven clinical trials is paramount to prevent data breaches and privacy violations.
Robust cybersecurity measures are essential to safeguard patient data and proprietary research findings. This includes encryption, access controls, and regular security audits to identify and mitigate vulnerabilities.
Companies will need to partner with other organizations or build their own way toward a level of functionality that creates meaningful value in order to meet the high bar for data security and information governance.
For more insights, see: How Has Generative Ai Affected Security
Smart Data Management
Smart data management is a game-changer for clinical trials. By combining traditional and generative AI capabilities, data management can be automated across multiple steps.
Databases can be created with just one click based on the protocol, and case report forms can be auto-generated based on protocol, patient profile, and visit type. This streamlines the process and reduces the risk of human error.
Take a look at this: Generative Ai and Knowledge Management
Data can then be reviewed and automatically cleaned in real time, and queries can be intelligently and efficiently auto-generated based on trial context, patient status, and site actions. This helps researchers focus on the most critical data gaps to be addressed before database lock.
With smart data management, potential benefits include a 30 percent-plus cost savings, a 50 percent-plus reduction in time to database lock, and 70 percent fewer manual queries.
If this caught your attention, see: Vector Database Generative Ai
High-Quality Data Requirements
High-quality data is essential for making informed predictions, especially in fields like drug discovery where vast and accurate genomic data is required to develop new cancer drugs.
Generative AI models demand extensive and precise datasets to function properly. Incomplete or erroneous data can lead to inaccurate predictions and failed experiments.
Pharmaceutical companies need comprehensive molecular data, patient profiles, and clinical trial results to develop effective treatments.
Broaden your view: Develop Generative Ai Solutions with Azure Openai Service
Regulatory Intelligence and Compliance
Gen AI-enabled intelligence engines can help pharma companies proactively anticipate Health Authority Queries (HAQs), reducing their number by approximately 30 percent. This enables teams to respond faster and more efficiently.
The pharmaceutical industry is heavily regulated to ensure patient safety, with the FDA having stringent criteria for approving new drugs. Pharmaceutical companies must ensure that their generative AI models meet regulatory standards.
Predictive analytics can help teams anticipate HAQs, thus reducing both initial and follow-up queries by 50 percent. This not only saves time but also informs regulatory strategy, risk management, and broader R&D strategy.
Demonstrating compliance with regulatory requirements is a significant hurdle, requiring extensive testing and validation to demonstrate safety and efficacy. This involves providing the necessary documentation for the approval of new drugs.
Readers also liked: Generative Ai Strategy
Challenges and Opportunities
Generative AI in pharma is a promising field, but it's not without its challenges. Data quality and integration are significant hurdles, as high-quality and comprehensive data are essential for AI models to succeed.
Integrating data from diverse sources, including clinical trials, genomic databases, and electronic health records, is a complex task. This challenge is exemplified by the need to establish clear guidelines for AI-driven drug discovery, which is crucial for ensuring safety and efficacy.
A different take: Generative Ai Photoshop Increase Quality
The lack of transparency in AI models can hinder trust and adoption in the scientific community. Model explainability and interpretability are crucial for understanding how AI models arrive at their predictions.
To fully unlock the potential of generative AI in pharma, we must proactively tackle these obstacles. This necessitates efficient collaboration among a multitude of stakeholders, including scientists, regulatory bodies, and the pharmaceutical industry.
Here are some of the key challenges and opportunities in generative AI for pharma:
- Data Quality and Integration: Ensuring high-quality and comprehensive data is essential for AI models to succeed.
- Model Explainability and Interpretability: Understanding how AI models arrive at their predictions is crucial for trust and adoption.
- Regulatory Considerations: Establishing clear guidelines for AI-driven drug discovery is crucial for ensuring safety and efficacy.
Despite these challenges, the opportunities presented by generative AI are vast and far-reaching. By addressing these challenges and fostering collaboration, we can unlock the full potential of AI to revolutionize drug discovery and development.
Worth a look: What Challenges Does Generative Ai Face
The Future is
The future of drug discovery is no longer confined to the lab bench. It lies in the realm of intelligent algorithms, powerful computing, and the boundless creativity of generative AI.
Pharmaceutical manufacturers are already using foundational models for purposes such as identifying targets, developing validation assays, and singling out promising leads.
Gen AI could help accelerate the process of discovering and developing new drugs, potentially cutting drug discovery timelines in half.
The average cost of bringing one drug to market is rising, not falling. Gen AI could help address this issue.
Generative AI is not just a technological marvel; it’s a beacon of hope, promising a future where life-saving medicines reach patients faster.
The potential opportunity for gen AI in the pharma industry is massive, estimated to be between $15 billion to $28 billion.
On a similar theme: Generative Ai Not Working Photoshop
Industry and Expertise
Bridging the gap between generative AI experts and life scientists is crucial for effective collaboration in the pharmaceutical industry. This requires cross-disciplinary training programs that teach the basics of generative AI and data science to life scientists, while generative AI researchers gain insights into the nuances of biology and chemistry.
Collaborative research between pharmaceutical companies, generative AI researchers, and regulatory bodies can establish data-sharing standards and best practices. This can be achieved by allocating resources judiciously and focusing investments on areas where generative AI can have the most significant impact.
To facilitate collaboration, pharmaceutical companies can promote educational programs that bring together generative AI experts and life scientists. This ensures that both sides understand each other’s needs and constraints, enabling them to work together effectively.
Here's an interesting read: Companies Using Generative Ai
From Scale: Rewiring the Organization
To truly capture gen AI's value, life science companies need to design systems for eventual scaling rather than implementing the technology as isolated solutions.
A comprehensive strategy clearly linked to scientific and business value is essential, supported by a robust technology infrastructure that includes an end-to-end tech stack.
The product-platform approach is a successful model, where the IT enterprise group leads the development of the platform infrastructure and provides an API for creating use cases.
Companies must reimagine their operating models to make this shift happen, avoiding the extremes of highly decentralized or top-down platform-based models.
A balanced approach that moves between speed and innovation with quality control, financial discipline, and organizational symmetries is key.
Pharmaco leaders will need to ask important questions about structures, processes, technologies, data, people, and change management to reimagine each step of the value chain.
To balance speed and innovation, leaders must strive to find the right mix of talent and guidelines to mitigate risk and ensure trust and buy-in from stakeholders.
The goal is to create a paradigm shift in the discovery, development, and delivery of therapies to patients, but it requires a clear strategy and infrastructure to support it.
A different take: Generative Ai for Business Leaders
Life Sciences Expertise
Collaboration between generative AI experts and life scientists is essential for effective use of generative AI in the pharmaceutical industry.
To bridge the gap, cross-disciplinary training programs can help researchers in both domains understand each other's language and work collaboratively. This can be achieved through courses that teach the basics of generative AI and data science for life scientists, and insights into the nuances of biology and chemistry for generative AI researchers.
Life scientists need to understand the biological relevance and potential side effects of molecules recommended by generative AI researchers. To address this challenge, collaborative research and data-sharing standards can be established between pharmaceutical companies, generative AI researchers, and regulatory bodies.
Five key areas require synergistic efforts: collaborative research, ethical frameworks, resource planning, interdisciplinary training, and transparency and interpretability. By addressing these challenges, generative AI projects in pharma can transition from experimental silos to strategic and holistic components of the organizational roadmap.
Related reading: How Is Generative Ai Trained
Here are some key areas to focus on:
- Collaborative research: Encourage collaboration between pharmaceutical companies, generative AI researchers, and regulatory bodies.
- Ethical frameworks: Develop ethical guidelines for using patient data in drug discovery and ensure that generative AI-driven decisions prioritize patient safety and informed consent.
- Resource planning: Allocate resources judiciously by identifying areas where generative AI can have the most significant impact.
- Interdisciplinary training: Promote educational programs that facilitate collaboration between generative AI experts and life scientists.
- Transparency and interpretability: Invest in research to make generative AI models more interpretable.
Frequently Asked Questions
What is an example of generative AI in healthcare?
Generative AI in healthcare is being used to design new drug candidates, potentially speeding up the development process. This innovative approach could revolutionize the way we develop life-saving medications.
What are the three steps to value with generative AI for pharmaceutical companies?
To unlock the value of generative AI, pharmaceutical companies should focus on identifying potential use cases, building the right data infrastructure and governance, and partnering with experts to access the latest tools and strategies. By following these three steps, companies can harness the power of generative AI to drive innovation and growth.
Sources
- https://www.mckinsey.com/industries/life-sciences/our-insights/generative-ai-in-the-pharmaceutical-industry-moving-from-hype-to-reality
- https://www.veritis.com/blog/from-concept-to-cure-generative-ai-in-drug-discovery/
- https://innodata.com/generative-ai-in-drug-discovery-how-ai-is-transforming-pharma/
- https://www.griddynamics.com/blog/generative-ai-drug-development
- https://www.uptech.team/blog/generative-ai-in-the-pharmaceutical-industry
Featured Images: pexels.com